没有合适的资源?快使用搜索试试~ 我知道了~
温馨提示
内容概要:本文探讨了高光谱图像无监督聚类面临的一些挑战,特别是数据规模大、维度高以及易受噪声污染等问题,提出了两个新方法——基于双图融合的子空间聚类模型(DGFSC)和基于光谱-空间超像素锚图聚类(S3AGC)以提高高光谱影像的聚类准确性并降低了计算复杂度。 适用人群:适合对高光谱图像分析感兴趣或从事地理信息系统相关工作的研究人员和技术开发者。 使用场景及目标:适用于那些希望改进HSI数据聚类效率且追求高准确度的情景中。 其他说明:两项新技术已经在多个公开的高光谱图像基准上进行了严格的实验验证,证明了他们相比现有技术的优势。
资源推荐
资源详情
资源评论
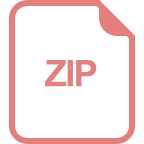
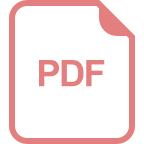
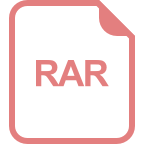
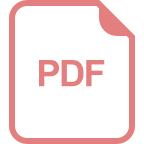
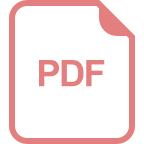
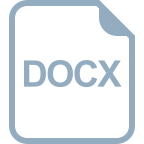
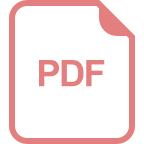
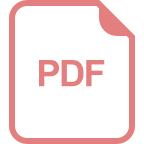
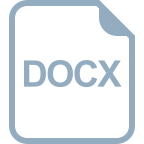
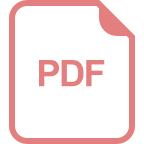
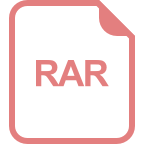
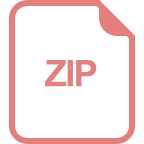
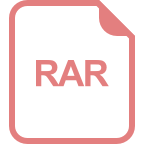
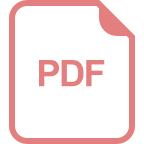
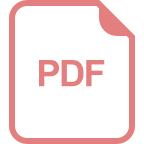
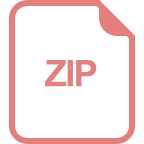
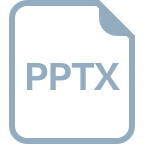
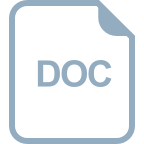
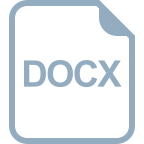
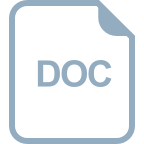
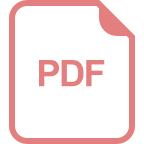
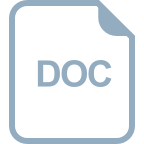
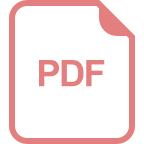
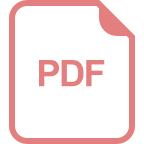
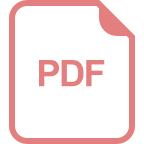
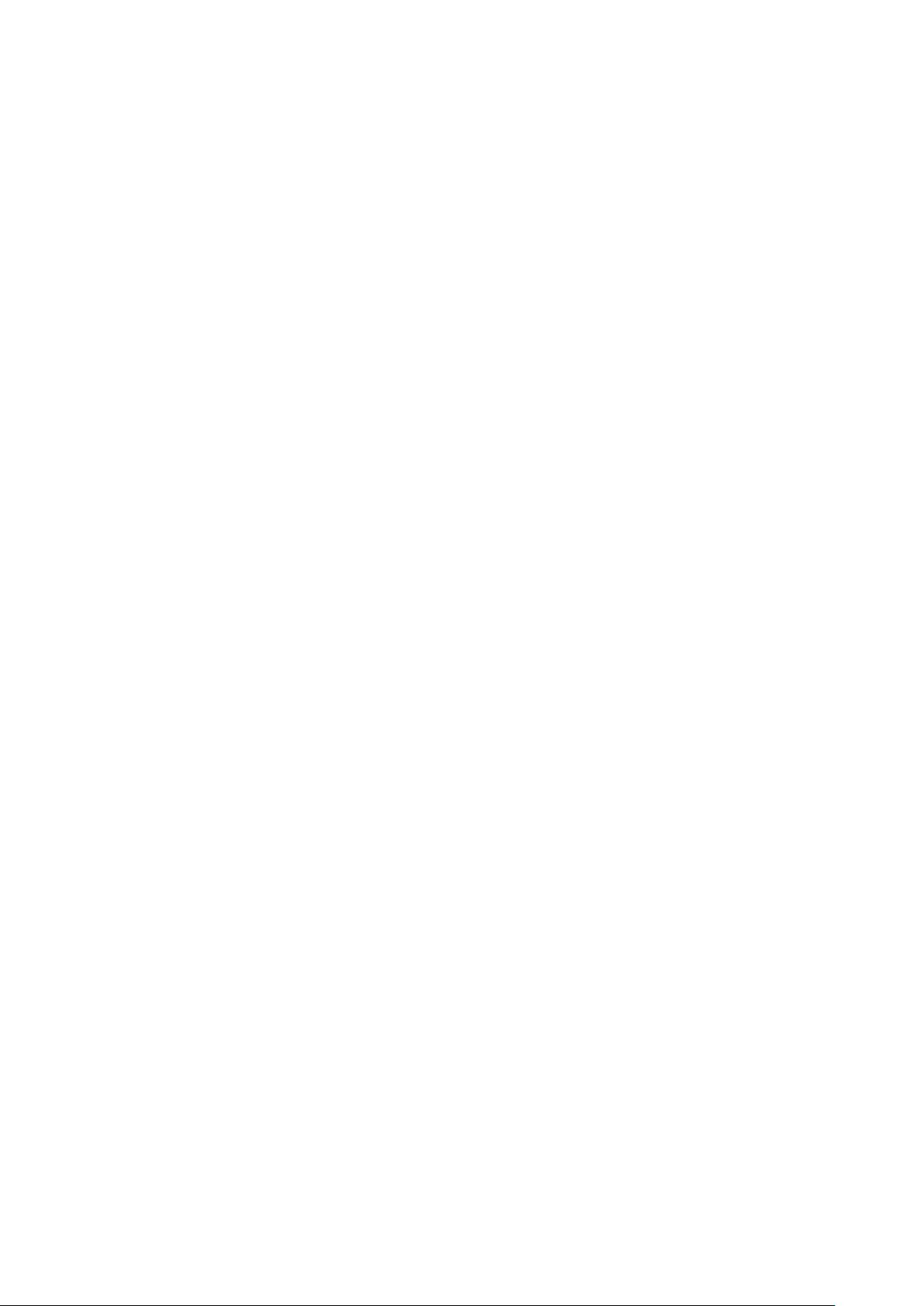
摘要
高光谱遥感技术利用数百个连续细分的波段对目标区域同时成像,形成包含
丰富空间光谱信息的三维高光谱图像。如何对高光谱图像的内容进行精确识别是
目前重要的研究课题。无监督高光谱图像聚类旨在没有类别标记信息的情况下,根
据像素间的内在相似性实现像素的归类划分。传统的高光谱图像聚类方法没有考
虑到像素点间的几何空间特征,把高光谱数据当成无序的集合来进行聚类。当高光
谱数据空间-光谱结构简单且不受像素噪声干扰时,传统的聚类方法通常可以获得
良好的聚类精度。然而,由于高光谱图像的规模较大、高光谱数据的固有结构复杂
以及容易受到噪声污染等特性,无监督高光谱图像聚类仍是一项具有挑战性的任
务。基于此背景,本文充分考虑高光谱图像的结构化信息,开展了基于图表示的高
光谱图像聚类方法研究。本文研究内容如下:
(1)为了充分利用高光谱图像的空谱结构化信息,本文提出一种基于双图融
合的子空间聚类模型(Dual Graph Fusion Subspace Clustering, DGFSC),以有效地
将像素划分为不同的类。DGFSC 在基本的子空间聚类模型中整合了图卷积的概念,
以充分挖掘和利用潜在的结构化信息。DGFSC 利用超像素分割技术获得的超像素
构造超像素级特征,用于构造具有空间信息的像素相似图以及包含波段信息的波
段相似图。DGFSC 基于这两个相似图的对偶图卷积,从图表示的数据中学习到了
更鲁棒的亲和矩阵。该模型通过联合考虑空间信息和结构化信息有效的提高了聚
类性能,大大减少了子空间聚类模型的计算复杂度。
(2)针对传统聚类算法空谱信息利用率低以及计算复杂度高的问题,本文进
一步提出了一种基于光谱-空间超像素锚图的聚类方法(Spectral-Spatial Superpixel
Anchor Graph-based Clustering,S
AGC)。 S
AGC首先利用超像素分割得到的局部同
质区域,用于去除高光谱图像中的噪声像素。基于均匀区域的空间分布,使用图卷
积的方法将波段的结构信息嵌入到基于锚图的聚类框架中,获得了更好的聚类性
能。此外,S
AGC提出了一种有效的锚点选择策略,以缓解随机选择或 k-means 聚
类方法选择锚点的不稳定性。与一般模型不同,S
AGC通过同时考虑空间结构化信
息以及锚点挑选策略,在较短时间内获得了更好的聚类精度。
本文在不同高光谱图像数据集上进行了实验验证,实验结果表明本文提出的
DGFSC 和S
AGC方法能够利用更深层次的空间-光谱结构化信息获得了较高精度。
关键词:高光谱图像聚类;子空间聚类;图卷积;超像素分割;基于锚图的聚
类
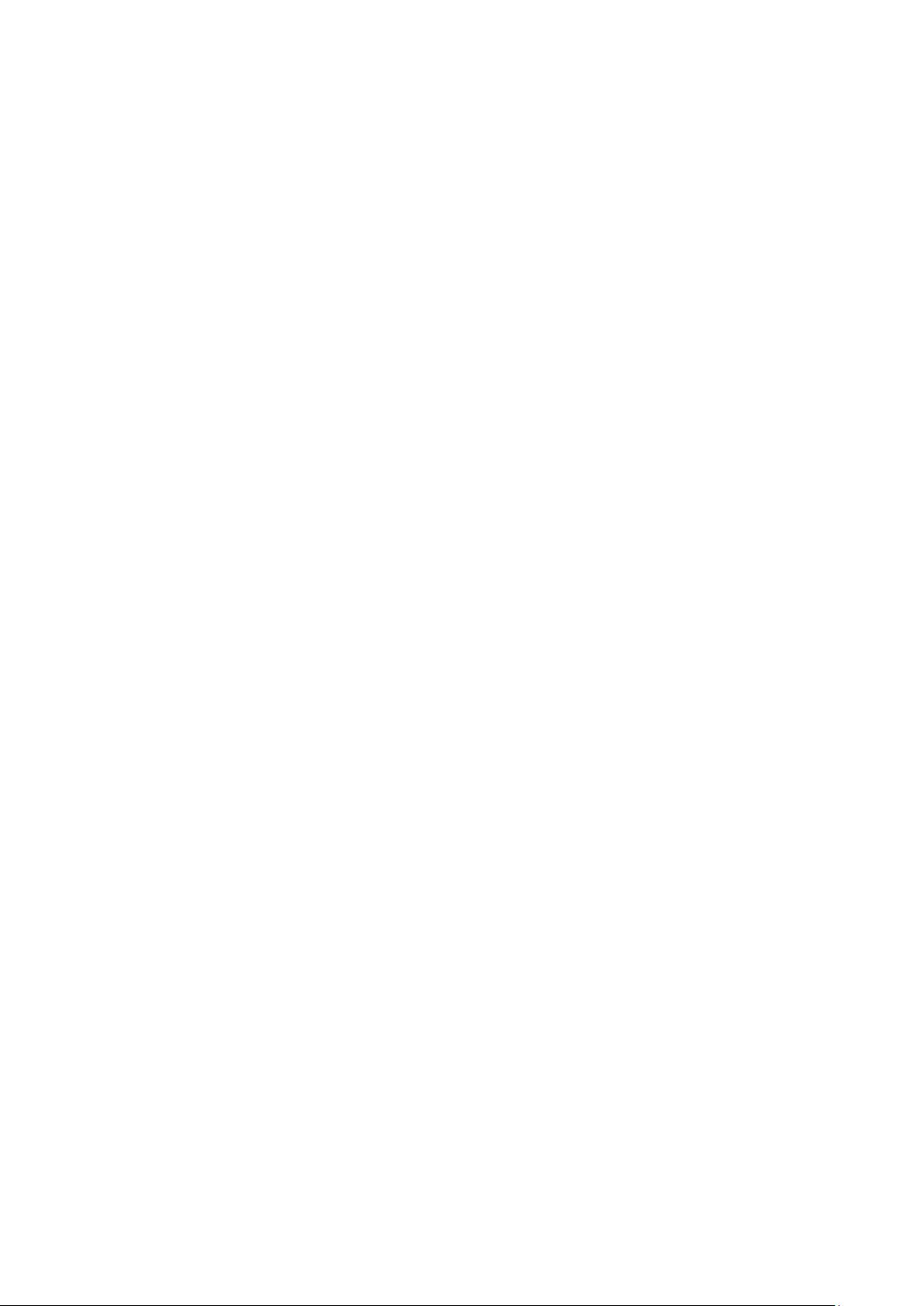
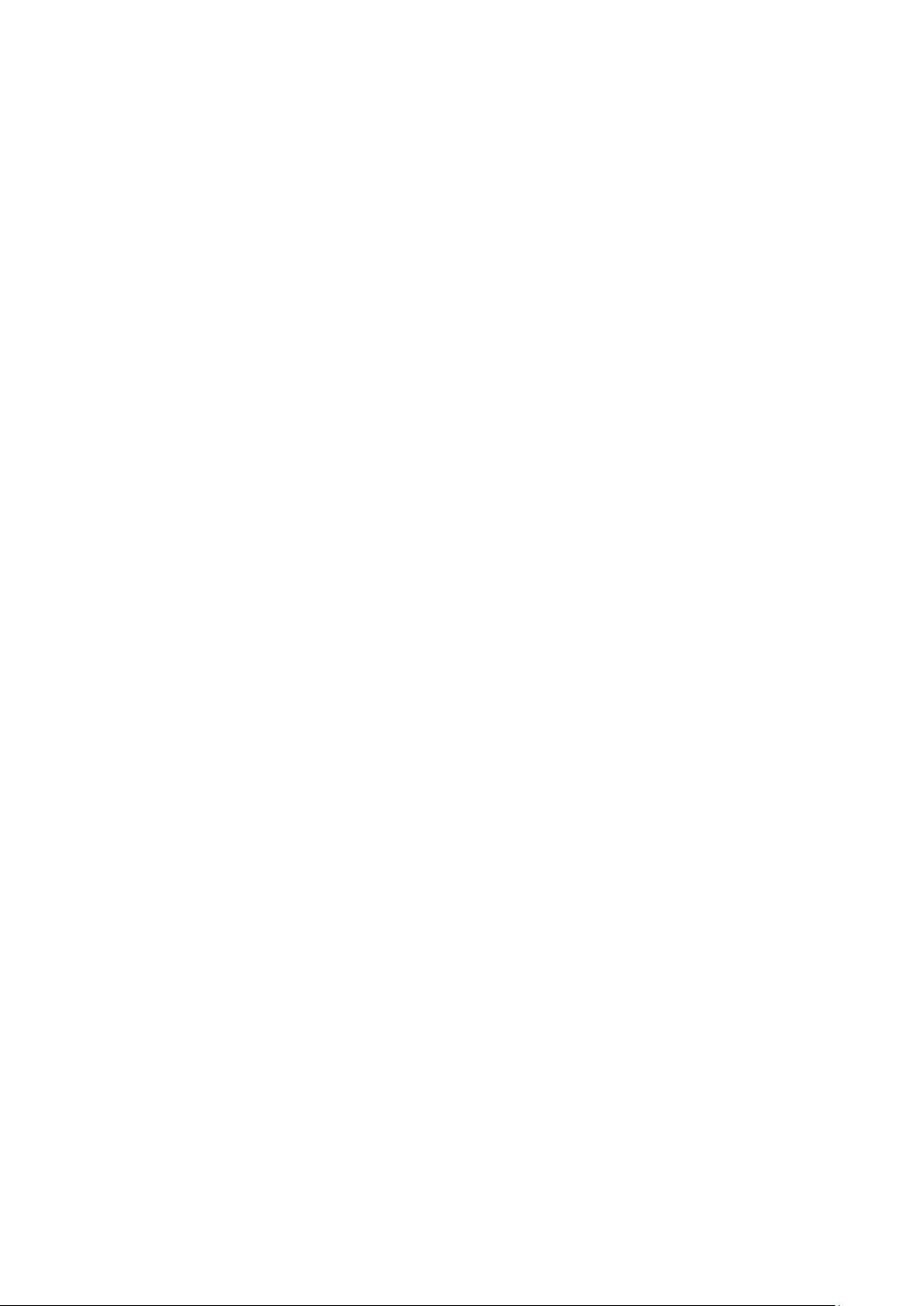
Abstract
Hyperspectral images containing numerous spatial pixels and hundreds of
continuous spectral bands are obtained with spectrometers. Accurately identifying the
content of hyperspectral images is currently an important research topic. Unsupervised
hyperspectral image clustering aims to classify and partition pixels based on their inherent
similarities without category label information. Traditional high-spectral image clustering
methods did not consider the geometric spatial features between pixels, treating high-
spectral data as an unordered set for clustering. When the spatial-spectral structure of
hyperspectral data is simple and not affected by pixel noise, traditional clustering methods
can usually achieve good clustering accuracy. However, due to the large scale of high-
spectral images, the complexity of the inherent structure of high-spectral data, and
susceptibility to noise pollution, unsupervised high-spectral image clustering remains a
challenging task. Against this background, this thesis fully considers the structured
information of high-spectral images and conducts research on graph-based high-spectral
image clustering methods. Specifically, the research content of this thesis is as follows:
(1) To fully utilize the spatial-spectral structured information of hyperspectral
images, we propose a spectral-spatial subspace clustering model, called dual graph fusion
subspace clustering (DGFSC), to effectively partition pixels into different clusters.
DGFSC integrates the concept of graph convolution into the basic subspace clustering
model to fully explore and utilize potential structured information. The DGFSC method
employs superpixel segmentation to obtain superpixel-level features, which are used to
construct a pixel similarity graph with spatial information and a band similarity graph
with spectral information. Based on the dual graph convolution of these two similarity
graphs, DGFSC learns a more robust affinity matrix from the graph-represented data. By
jointly considering spatial and structured information, the model effectively improves
clustering performance.
(2) To address the low utilization of spatial information and high technical
complexity of traditional clustering algorithms, a spectral-spatial superpixel anchor
graph-based clustering (S
AGC) method is proposed for hyperspectral images. S
AGC
first uses locally homogeneous regions obtained through superpixel segmentation to
remove noise pixels in hyperspectral images. Based on the spatial distribution of uniform
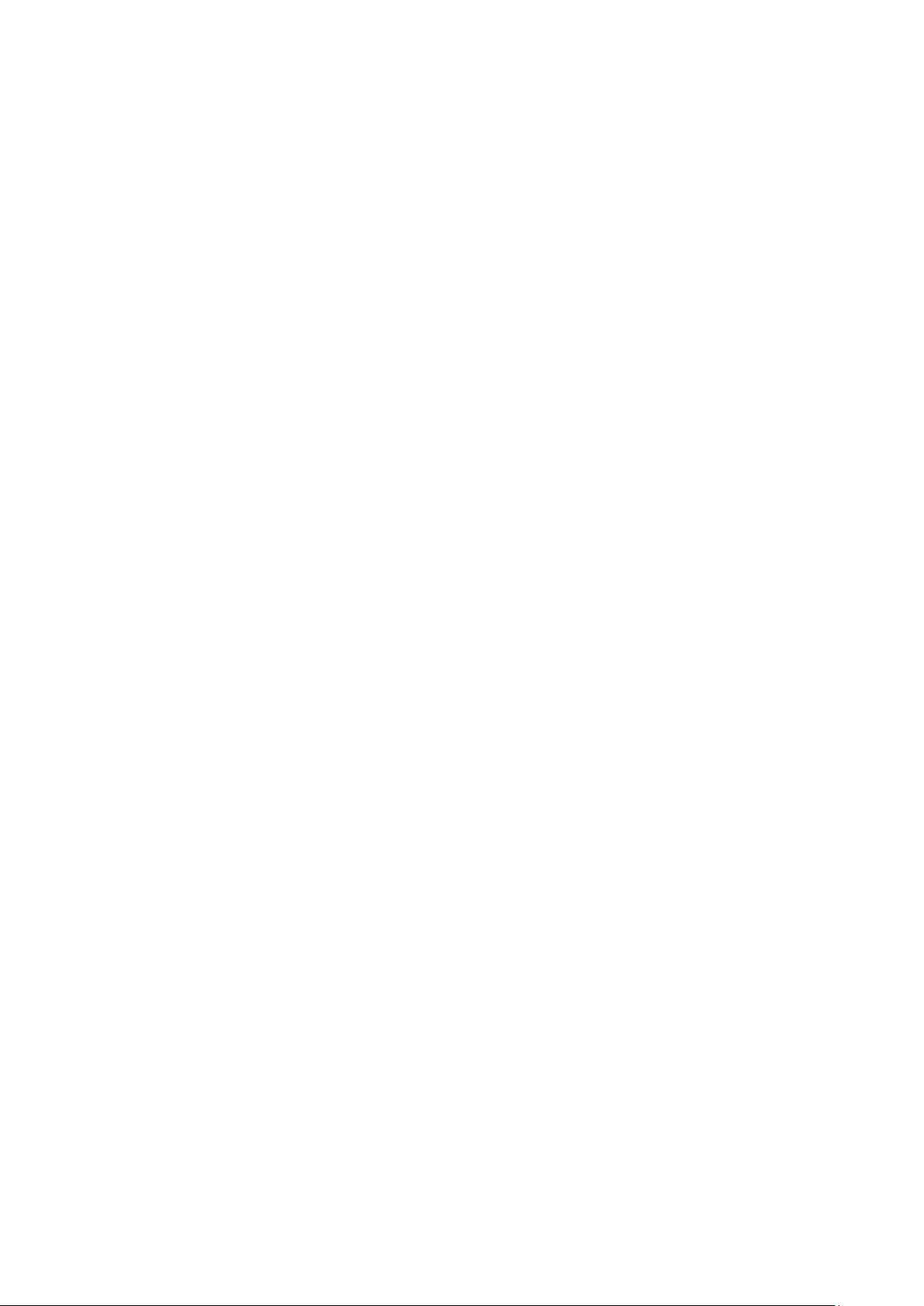
regions, the method embeds the structural information of bands into the anchor graph-
based clustering framework using graph convolution. In addition, S
AGC proposes an
effective anchor selection strategy to alleviate the instability of random selection or k-
means clustering method for anchor selection. Unlike conventional models, S
AGC
further improves clustering performance by simultaneously considering spatial structured
information and anchor selection strategy.
This experimental validation in different hyperspectral image datasets shows that the
proposed method DGFSC and S
AGC can use higher spatial-spectral structural
information to obtain high classification accuracy.
Key Words: Hyperspectral image clustering; Subspace clustering; Graph
convolution; Superpixel segmentation; Anchor graph-based clustering
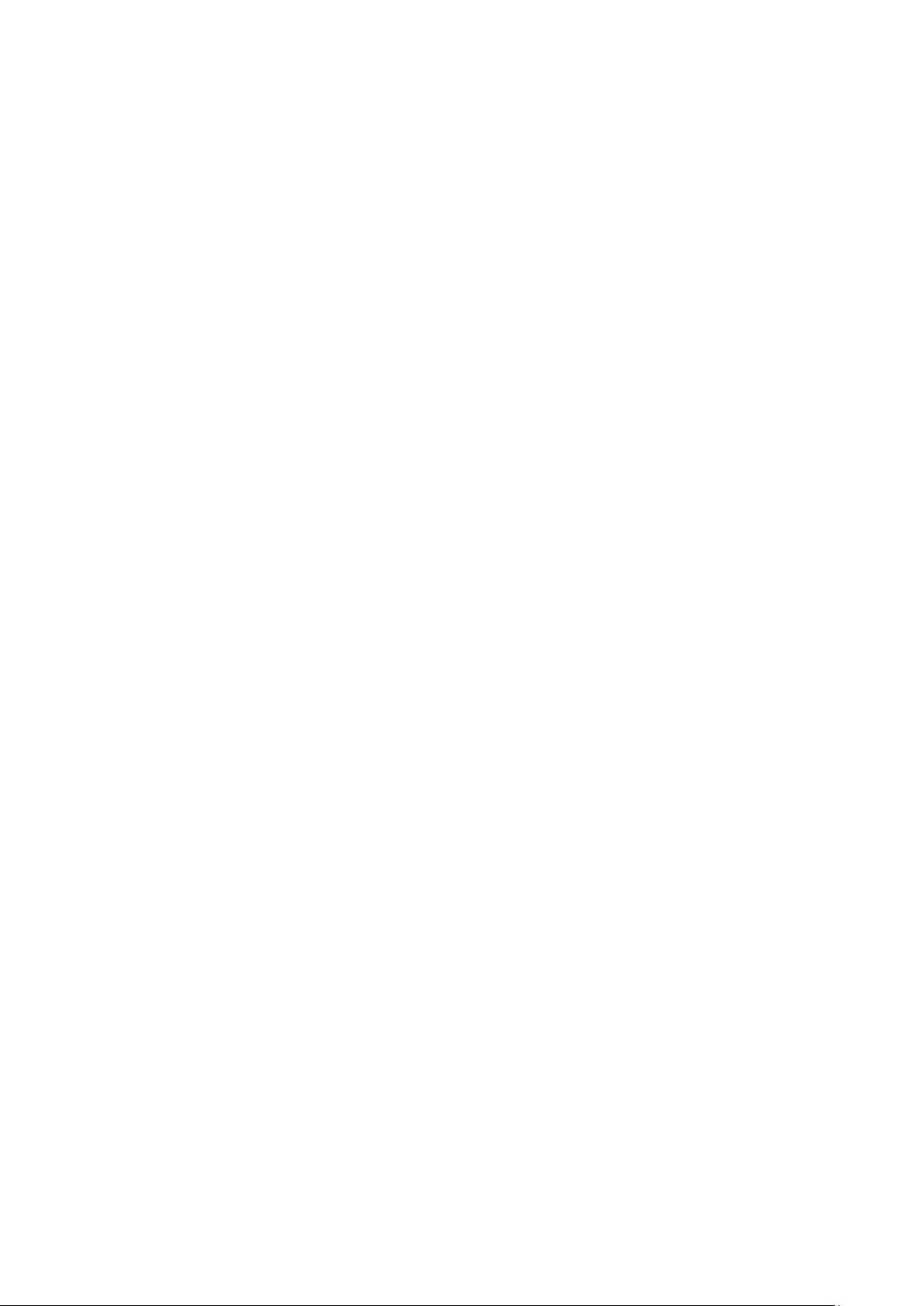
目 录
第一章 绪论 ............................................................................................................ 1
1.1 研究背景与意义 ....................................................................................... 1
1.2 高光谱图像聚类方法研究现状及其问题 ............................................... 3
1.2.1 传统高光谱图像聚类算法 ............................................................ 3
1.2.2 基于图的高光谱图像聚类算法 .................................................... 4
1.2.3 现有研究难点 ................................................................................ 5
1.3 研究内容与章节组织安排 ....................................................................... 6
1.3.1 本文研究内容 ................................................................................ 6
1.3.2 本文章节安排 ................................................................................ 7
第二章 相关理论研究 ............................................................................................ 8
2.1 高光谱图像介绍 ....................................................................................... 8
2.2 高光谱图像空谱信息提取技术理论基础 ............................................... 9
2.2.1 超像素分割 .................................................................................... 9
2.2.2 图卷积神经网络 ........................................................................... 11
2.3 高光谱图像聚类方法理论基础 ............................................................. 12
2.3.1 子空间聚类理论基础 .................................................................. 12
2.3.2 基于锚图的聚类理论基础 .......................................................... 13
2.4 本章小结 ................................................................................................. 15
第三章 基于双图融合的高光谱图像子空间聚类方法 ...................................... 16
3.1 引言 ......................................................................................................... 16
3.2 基于双图融合子空间聚类方法 ............................................................. 17
3.2.1 总体结构 ...................................................................................... 17
3.2.2 超像素特征生成 .......................................................................... 18
3.2.3 相似图的构造 .............................................................................. 18
3.2.4 模型提出 ...................................................................................... 19
3.2.5 模型优化与求解 .......................................................................... 21
3.2.6 谱聚类 .......................................................................................... 21
3.3 实验结果与参数分析 ............................................................................. 23
3.3.1 实验数据集介绍 .......................................................................... 23
3.3.2 实验设置 ...................................................................................... 25
剩余61页未读,继续阅读
资源评论
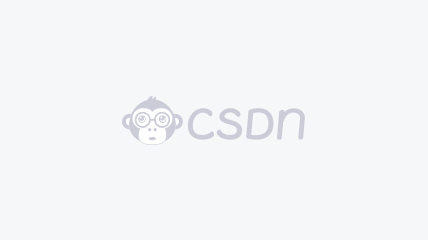

pk_xz123456
- 粉丝: 2325
- 资源: 2475
上传资源 快速赚钱
我的内容管理 展开
我的资源 快来上传第一个资源
我的收益
登录查看自己的收益我的积分 登录查看自己的积分
我的C币 登录后查看C币余额
我的收藏
我的下载
下载帮助

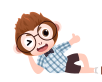
最新资源
- 下载.png112分v额且财务去去
- devecostudio-windows-4.1.0.400
- STSPIN32G4-demo板资料
- 昆虫害虫检测54-YOLO(v5至v9)、COCO、CreateML、Darknet、Paligemma、TFRecord、VOC数据集合集.rar
- jetbrains-JetBrains公司的IDE介绍及其在软件开发中的应用
- 284.基于java的旅游网站(含报告).zip
- python selenium爬虫用的浏览器和驱动96.0.4664.45
- 算法实现排序算法 Python 实现.zip
- JavaWeb资源代码文档资料.zip
- 哈希表-数据结构领域哈希表的概念、操作与应用场景
资源上传下载、课程学习等过程中有任何疑问或建议,欢迎提出宝贵意见哦~我们会及时处理!
点击此处反馈


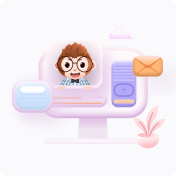
安全验证
文档复制为VIP权益,开通VIP直接复制
