没有合适的资源?快使用搜索试试~ 我知道了~
温馨提示
本文通过将多尺度语义分割模型、情感感知模型以及街景感知模型相结合来探究城市的活力特点及其决定因素,提出了融合街景影像、POI数据、路网数据及土地使用数据的研究架构。实验结果显示城市主要区域的整体城市活力较低,在视觉感知方面,城阳区、崂山区有相对优势;在情绪感知和综合维度方面,则市北区、市南区表现出色。同时研究指出了目前在交通通达度和设施便利程度上还有很大的改善空间。 适用人群:地理信息系统、城市规划专家和决策支持专业人员。 使用场景及目标:适用于对城市不同功能区进行评估,挖掘各个区块的特点并针对发现的问题提出解决方案,以此促进健康稳定的城市发展规划。 本研究采用多种模型组合的方法提供了一种全面而精确评价城市品质的新途径,强调了在经济开发时兼顾街区环境美学化与人性化的必要性。
资源推荐
资源详情
资源评论
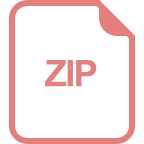
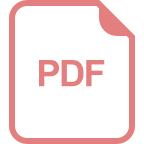
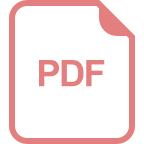
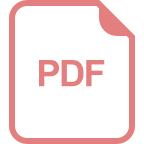
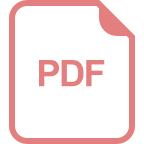
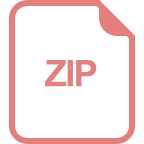
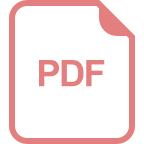
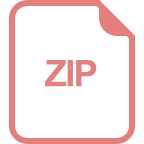
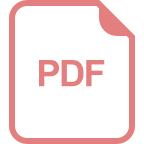
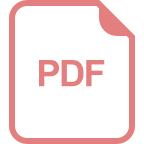
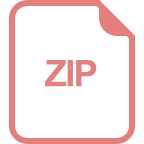
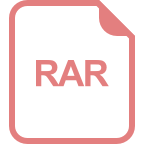
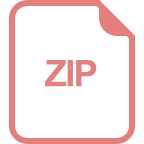
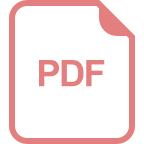
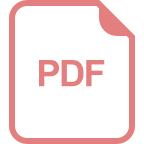
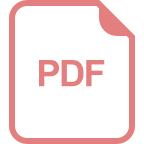
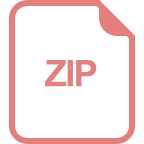
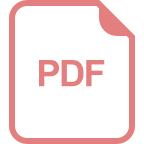
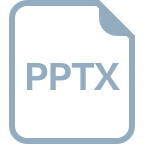
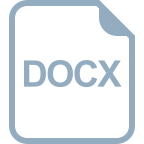
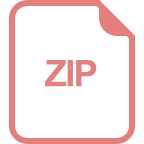
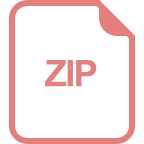
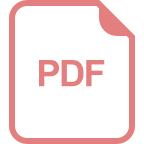
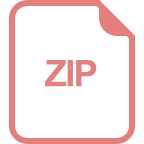
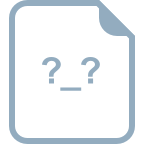
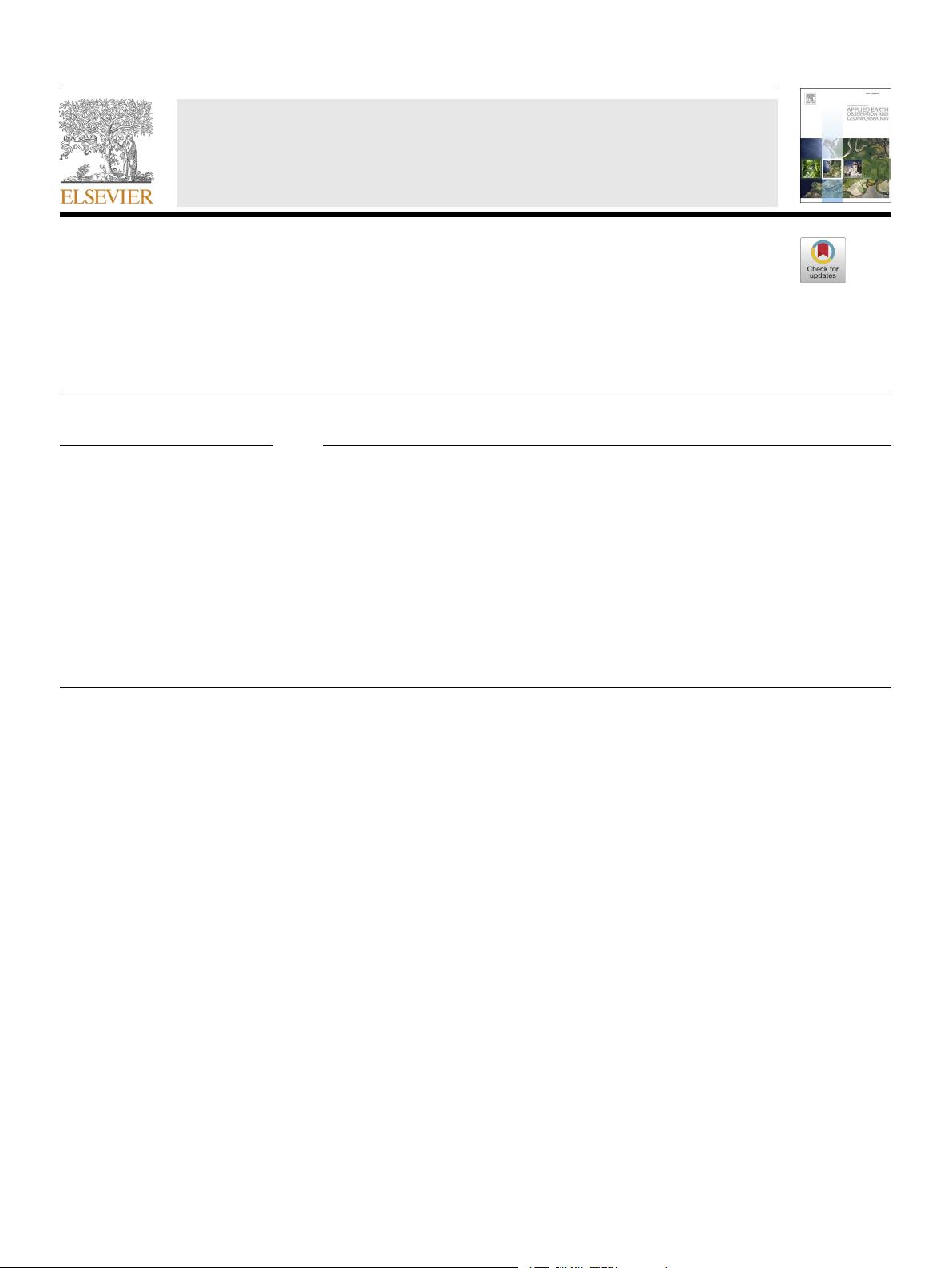
International Journal of Applied Earth Observation and Geoinformation 123 (2023) 103476
Available online 4 September 2023
1569-8432/© 2023 The Author. Published by Elsevier B.V. This is an open access article under the CC BY license (http://creativecommons.org/licenses/by/4.0/).
Deep exploration of street view features for identifying urban vitality: A
case study of Qingdao city
Zepeng Ma
College of Geodesy and Geomatics, Shandong University of Science and Technology, Qingdao 266590, China
ARTICLE INFO
Keywords:
Street view image
Deep learning algorithms
Semantic segmentation
SHAP method
Social perception
Urban vitality
ABSTRACT
Urban vitality has signicant practical implications for urban management and planning. In this study, we
propose a comprehensive research framework that combines street scene images, point of interest (POI) data,
road network data, and residential land data, and employs deep learning algorithms to explore the characteristics
and inuencing factors of urban vitality from a social perception perspective. By designing multi-scale semantic
segmentation models, emotion perception models, and street perception models, we deeply explore the street
features of the city. At the block level, we use weighted calculation methods to quantify urban vitality by
combining POI data and residential land data, accurately characterizing the city. Finally, we analyze the driving
factors of urban vitality using random forest and SHAP methods. The research results show that Chengyang
District and Laoshan District have advantages in visual perception, while Shibei District and Shinan District
exhibit advantages in urban vitality. The overall urban vitality in the main urban area of Qingdao City is low,
with high scores in emotion perception and visual perception, but low scores in transportation accessibility and
facility convenience. Visual perception factors play a signicant role in urban vitality, highlighting the impor-
tance of urban street beautication and humanized design in economic development and environmental con-
struction. The analytical results of this study contribute to optimizing urban spatial features and provide
references for urban planning and construction.
1. Introduction
The competitiveness of cities is closely related to national strength
(Zhang, 2020). In the 21st century, China has rapidly entered the track
of urbanization, bringing not only opportunities but also challenges. In
recent years, “urban issues” have become increasingly serious, such as
inadequate urban planning, poor urban openness and inclusiveness, lack
of cooperation and communication between cities, and the emergence of
empty or ghost cities in various regions (Zhao and Zhao, 2022). The
main reason for these problems is the uneven expansion of cities (Dang,
2023). In order to ensure high-quality and stable development of cities,
it is crucial to enhance urban vitality (Zhang, 2018).
The denition and research focus of urban vitality have been
continuously evolving. Early studies on urban vitality mainly focused on
the economic and market vitality of cities (Della Spina, 2019), consid-
ering urban vitality as the self-development ability of cities in terms of
production relations, as well as prosperity and stability in economic,
environmental, and social aspects. However, with a shift in research
thinking, scholars have started to understand urban vitality from
different perspectives and dimensions. For instance, Sulis et al., (2018)
pointed out that urban vitality is related to people’s behaviors in a
certain place. Liang et al., (2022) and Zhu et al., (2020) argued that
urban vitality can be represented through human activities and the
interactive features generated within the city.
A comprehensive measurement of urban vitality is a focal issue in
urban studies, yet there is currently a lack of a xed indicator system
that can fully and accurately reect the level of urban vitality. Previous
studies have proposed different indicators and evaluation methods. For
example, Gülden Demet and Giritlio
˘
glu, (2008) used the number of
residences, population, commercial areas, and the price per square
meter of land as indicators of urban vitality. Nia, (2021) argued that
urban aesthetics design has a direct impact on the level of urban vitality.
Fuentes et al., (2020) evaluated the vitality of communities in Santiago,
Chile, based on the diversity of urban form, architecture, and spatial
characteristics. These evaluation methods are subjective to a certain
extent and cannot comprehensively and accurately measure urban
vitality.
In recent years, scholars have closely associated urban vitality with
human behavior. They use data collection and analysis of people’s be-
haviors to understand the level of urban vitality. For example, Delcl
`
os-
Ali
´
o et al., (2019) analyzed and validated Jacobs’s theory of urban vi-
tality based on GIS data and data tracked on smartphones. Botta and
Guti
´
errez-Roig, (2021) argued that a vibrant urban environment refers
to areas in a city with high levels of human activity and interaction, and
Contents lists available at ScienceDirect
International Journal of Applied Earth
Observation and Geoinformation
journal homepage: www.elsevier.com/locate/jag
https://doi.org/10.1016/j.jag.2023.103476
Received 2 June 2023; Received in revised form 25 August 2023; Accepted 29 August 2023
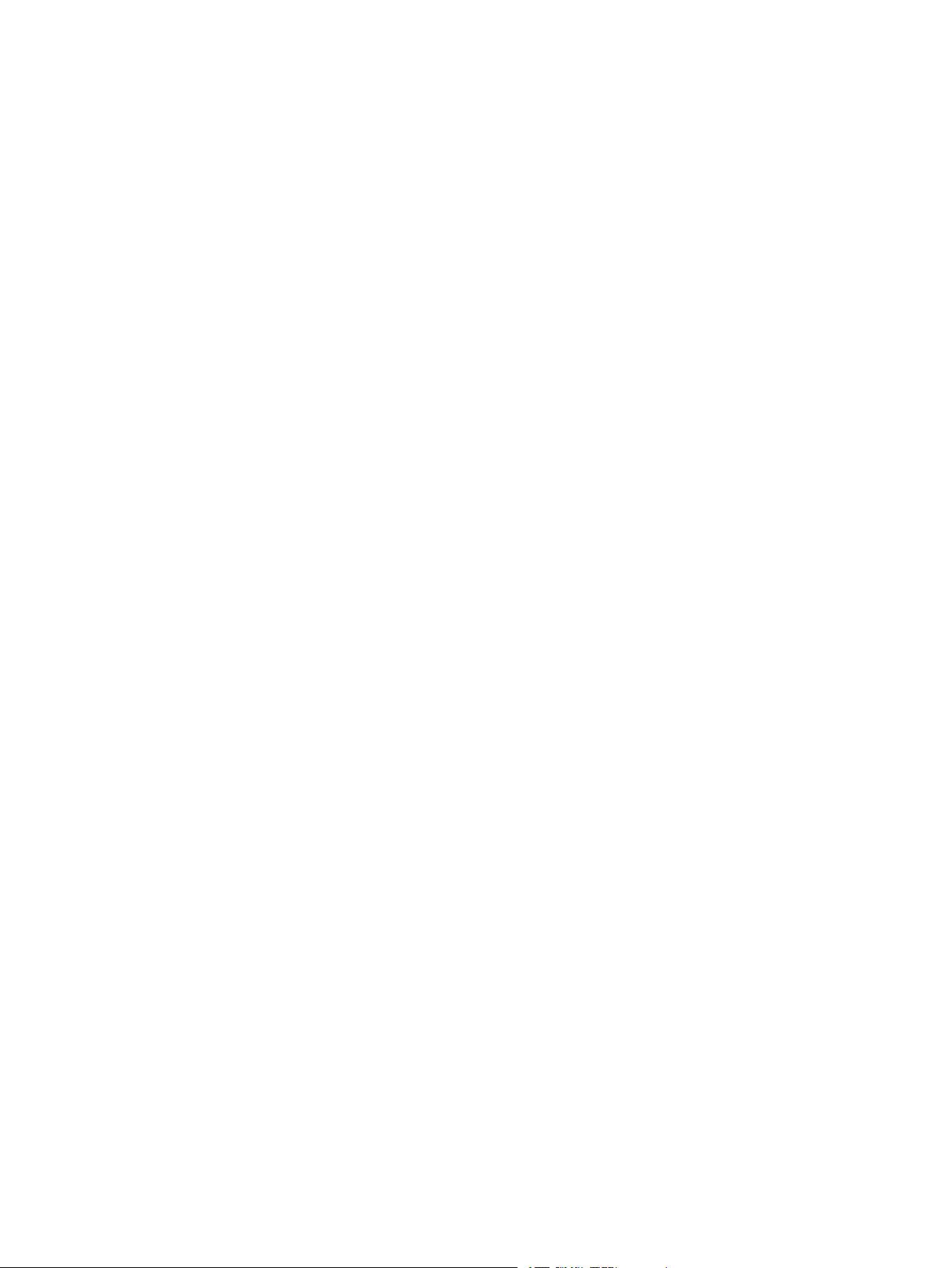
International Journal of Applied Earth Observation and Geoinformation 123 (2023) 103476
2
they demonstrated that combining mobile phone data with crowd-
sourced urban features can lead to a better understanding of urban ac-
tivity. Mao and Zhong, (2020) explored the level of urban vitality and its
inuencing factors based on POI (Points of Interest) data and location
services data, emphasizing the subjectivity of individuals in urban vi-
tality. Yin et al., (2019) used mobile app location data to describe the
ow of people across towns, exploring the distribution patterns of urban
vitality. This approach is closer to the essence of urban vitality and has
better objectivity. However, the difculties in obtaining mobile
signaling and location data, as well as the high data costs, greatly limit
research on urban vitality.
Street view images, as a new type of data source, has advantages of
wide coverage, providing street-level landscape information, and low
data collection costs. Street view imagery contains a wealth of visual
information about urban spaces, including static urban built environ-
ments and dynamic pedestrians or vehicles on streets, which offers new
perspectives and dimensions for urban research (Zhang, et al., 2019;
Voordeckers et al., 2021). Previous studies have utilized street view
imagery data in various research areas, such as investigating the impact
of greenery on mental health, exploring comfortable urban travel ex-
periences, and extracting semantic scenes. With the continuous expan-
sion of street view imagery data sources and advancements in the eld of
articial intelligence, it brings both new opportunities and challenges
for research in geographic spatial-related domains (Gao, 2020).
Deep convolutional neural networks (CNNs) have emerged as a
pivotal and effective tool in the advancement of computer vision,
playing an increasingly vital role in image recognition and classication
(Berman et al., 2014). Furthermore, they offer new opportunities for
modeling and perceiving geographic locations (Wang et al., 2020). In
order to quantitatively analyze the semantic aspects of urban scenes and
extract their deeper connotations, researchers have started employing
deep learning methods for scene classication, semantic segmentation,
and object detection in street-view imagery(Kruse et al., 2021; Yu and
Wang, 2016; Yin et al., 2015; Liang et al., 2017; Markevych et al., 2017).
However, challenges such as category confusion continue to plague
scene classication and semantic segmentation techniques in the pro-
cessing of street-view images (Tian et al., 2019a; Tian et al., 2019b). The
introduction of complex semantic scene factors has necessitated higher
demands for scene classication and semantic segmentation tasks
(Zhang, 2018).
This study presents a comprehensive research framework to over-
come the limitations of previous studies. Specically, we employ ne-
scale street view imagery and deep dive into urban street features
from the perspective of social perception by utilizing a designed multi-
scale semantic segmentation model, emotional perception model, and
street perception model. Subsequently, at the plot scale, we quantify
urban vitality through weighted calculations, combining point of in-
terest data and residential land data, to accurately depict urban char-
acteristics. Lastly, we analyze the factors driving urban vitality using
random forest and SHAP methods. This work makes the following
contributions: (1) It designs three deep learning models that fully exploit
feature information in street view data; (2) It provides a method to
measure urban vitality features at a ne scale; (3) By quantifying human
perception of locations and uncovering regional characteristics, it pro-
motes tailored approaches to balance urban development; (4) It eluci-
dates the non-linear relationship between urban street view features and
urban vitality. This work enables a more comprehensive and accurate
evaluation of urban vitality levels, providing effective guidance for
urban development. It is of great signicance in addressing current
urban issues and promoting healthy urban growth.
2. Study area and data
2.1. Study area
Qingdao is located in the southeastern part of Shandong Province. It
is the economic center of Shandong Province and one of the earliest
coastal cities in China. With the process of urbanization, Qingdao’s
streetscapes have exhibited more distinctive features. The main urban
area of Qingdao city is chosen as the study area to analyze the urban
vitality at the residential block level. The study area is shown in Fig. 1.
2.2. Data source and preprocessing
2.2.1. Street view data
Street view images are a novel source of data that provides users with
real-life visual representations of streets, buildings, and other urban
physical environments. In this study, Baidu Maps’ street view imagery
was selected as the data source. The street view imagery was obtained
using Python web scraping with the Baidu Street View Map API.
During the data preprocessing phase, road network data of the study
area was downloaded from OpenStreetMap and integrated into ArcMap
for road network simplication. To focus on the internal features of the
city, highways and roads where street view vehicles cannot capture
imagery were excluded. Road centerlines were extracted and compared
with available street view imagery on Baidu Maps . Road segments
without street view coverage were removed. Following the research
approach of (Yang et al., 2021), sampling points were obtained at in-
tervals of 50 m along the road centerlines to ensure comprehensive
photo coverage and balanced data size. The latitude and longitude of
each sampling point were computed, and the nearest geocoded location
for panoramic imagery was retrieved using the Baidu API. For each
location, the angle between the road and the front-facing street view was
calculated, and street view images were collected from four specic
camera angles, with a xed pitch angle of 20
◦
. Images were crawled for
sampling points at positions
α
+ 0
◦
,
α
+ 90
◦
,
α
+ 180
◦
, and
α
+ 270
◦
, as
shown in Fig. 2.
2.2.2. Semantic segmentation dataset
The semantic segmentation dataset used in this study was obtained
from the open-source Cityscapes dataset. The dataset includes a total of
3,475 nely annotated images, consisting of 2,975 training images and
500 validation images. Each image in the dataset has a resolution of
1024 × 2048 pixels.
Due to the fact that the Cityscapes dataset contains a total of 34
categories, this study focuses on 19 specic categories. Considering the
image size of the dataset, which is 1024 × 2048 pixels, in order to reduce
the GPU memory usage during model training, the image size was
resized to 768 × 768 pixels. Additionally, data augmentation techniques
were applied to the dataset. The performance of the model was evalu-
ated using the validation set for accuracy assessment. The sample data
and labels of the dataset are shown in Fig. 3.
2.2.3. MIT places pulse 2.0 dataset
The MIT Places Pulse 2.0 dataset is a crowdsourced dataset for urban
landscape perception in street view images, publicly released by the MIT
Media Lab. It consists of 110,988 images captured between 2007 and
2012, sampled from 56 major cities in 28 countries across six continents.
The global coverage of the dataset makes it an ideal choice for training
urban perception models. The dataset is formed by collecting ratings
from different participants through information collection platforms
(Fig. 4) who rated pairs of random street view images from six di-
mensions: “beautiful,” “depressing,” “lively,” “wealthy,” and “boring.”
Zhang et al., (2018a) and Zhang et al., (2018b) employed a statistical
method similar to the concept of “schedule intensity” in sports compe-
titions (Park and Newman, 2005) to calculate the perception scores for
each dimension, resulting in scores distributed within the range of [0,
10]. In our research, we sampled this dataset to specify our dataset for
predicting urban perception.
2.2.4. COCO Captions datasets
The COCO Captions dataset, also known as Microsoft COCO
Z. Ma
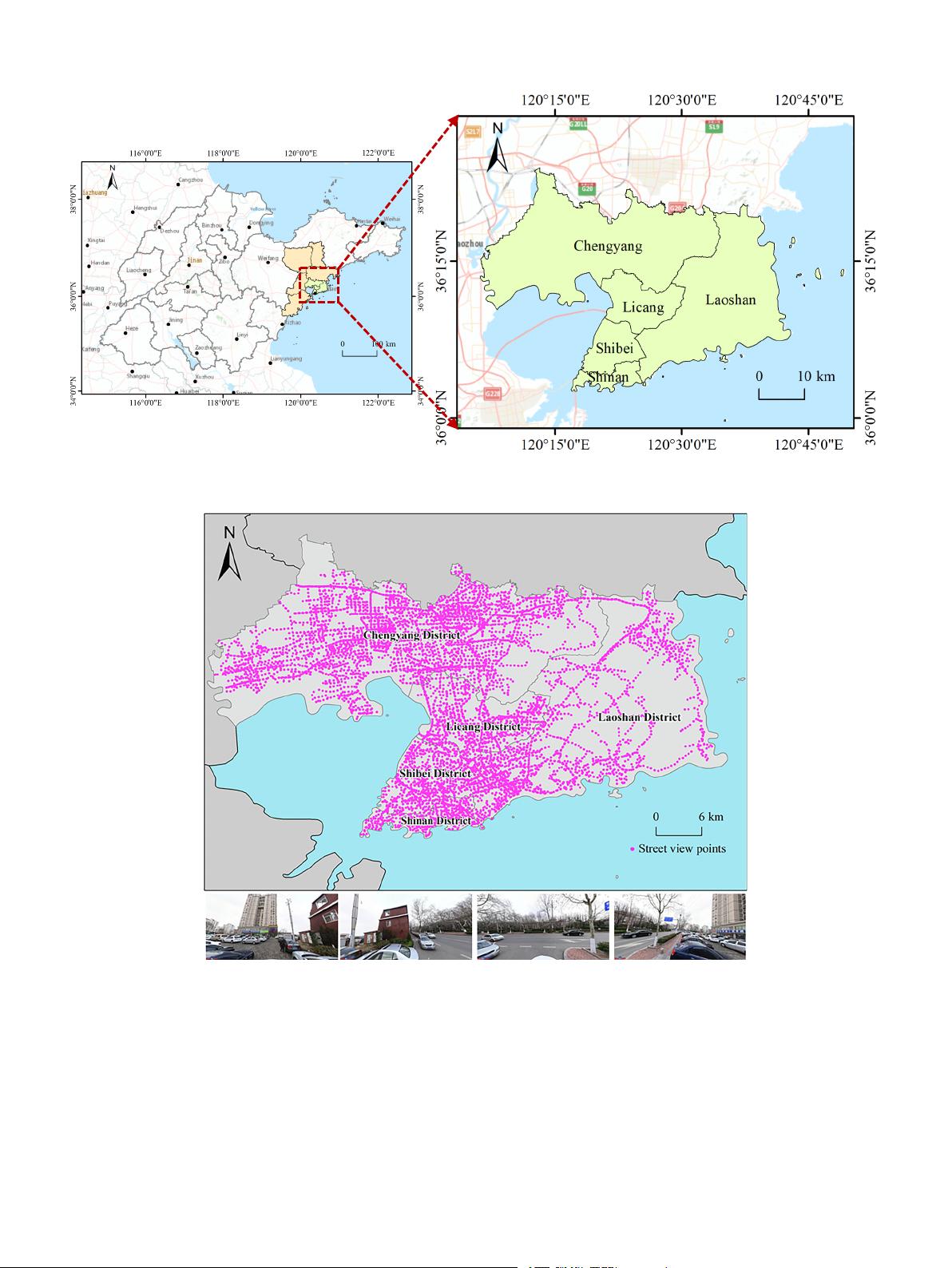
International Journal of Applied Earth Observation and Geoinformation 123 (2023) 103476
3
(Common Objects in Context) Captions dataset, is a commonly used
computer vision dataset. This dataset contains images from various real-
world scenes, along with associated caption descriptions. It encompasses
over 120,000 images and contains more than 500,000 caption de-
scriptions. The COCO Captions dataset is widely used in tasks involving
both images and text, such as image captioning and image caption
generation. The sample data and corresponding text labels of the dataset
are shown in Fig. 5.
In our research, we utilized this dataset as the training dataset for our
LSTM_CNN model to predict textual information in street view images.
2.2.5. Other datasets
Residential plot data was obtained from vectorized land-use data and
served as the evaluation unit and quantication carrier in this study.
Infrastructure point-of-interest (POI) data, sourced from Amap, was
used to calculate indicators such as facility convenience dimension.
3. Methods
The framework of this study consists of two main parts: indicator
establishment and urban vitality calculation. The framework can be
Fig. 1. Range of the study area.
Fig. 2. Spatial distribution of street view sampling points.
Z. Ma
剩余13页未读,继续阅读
资源评论
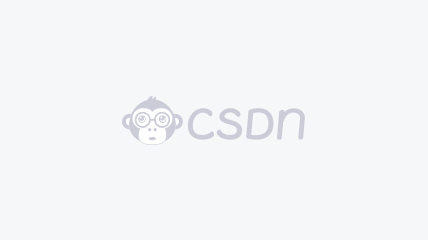

pk_xz123456
- 粉丝: 2750
- 资源: 3914
上传资源 快速赚钱
我的内容管理 展开
我的资源 快来上传第一个资源
我的收益
登录查看自己的收益我的积分 登录查看自己的积分
我的C币 登录后查看C币余额
我的收藏
我的下载
下载帮助

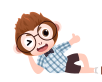
最新资源
- 铁路轨道缺陷数据集,4278张原始图片,支持YOLOV5格式的标注,可识别是否有裂缝,间隙缺陷, 图片和标注信息可参考:https://backend.blog.csdn.net/article/de
- alkatip5.9 维吾尔、哈萨克语输入法
- COMSOL孔隙渗流下的细颗粒迁移运动 对土石混合体进行了数值仿真,考虑了土石混合体孔隙变化,细颗粒侵蚀,骨架结构变形,此问题是一个多场(渗流场、变形场、应力场、损伤场)多相介质(土颗粒集合体,块石
- Python近年考试真题(免费领取!还有和我一样良心的博主了吗)
- 铁路轨道缺陷数据集,4278张原始图片,支持COCO JSON格式的标注,可识别是否有裂缝,间隙缺陷, 图片和标注信息可参考:https://backend.blog.csdn.net/articl
- 使用LSTM预测风功率 1、进行了数据清洗(数据清洗前后对比图) 2、相关性分析 3、进行了多次模型参数调优实验(神经元个数、dropout等) 4、绘制了loss曲线 5、预测了未来5步并将每步的对
- vivado2019.2平台使用verilog开发的OFDM调制解调系统,包括编译码,FFT,IFFT,CP等,包含testbench,有视频指导怎么使用
- 压制和平公益美化V3.zip
- 基于PLC的养殖场环境监测控制系统 包括梯形图 电气图 可根据要求进行修改(需要另外加) 博途v15.1版本及以上均可打开 西门子plc1200 当各个电动机运行时 实时参数也会发生相应
- CD盒全自动折弯机sw18可编辑全套技术资料100%好用.zip
- vb+access家庭理财VB6.0系统源代码
- 基于SPMSM(自建电机模型)的模型参考自适应参数辨识simulink模型(r2021b) 效果较好,可做斜坡辨识
- 数媒 作品报告 参考案例(1).zip
- 数字信号处理知识点及习题
- 弹药盒自动组装检测设备sw18可编辑全套技术资料100%好用.zip
- 电阻片自动点胶机(sw20可编辑+cad)全套技术资料100%好用.zip
资源上传下载、课程学习等过程中有任何疑问或建议,欢迎提出宝贵意见哦~我们会及时处理!
点击此处反馈


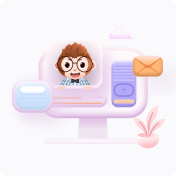
安全验证
文档复制为VIP权益,开通VIP直接复制
