# Copyright (c) OpenMMLab. All rights reserved.
import copy
import os.path as osp
from typing import Callable, Dict, List, Optional, Sequence, Union
import mmengine
import mmengine.fileio as fileio
import numpy as np
from mmengine.dataset import BaseDataset, Compose
from mmdet.registry import DATASETS
@DATASETS.register_module()
class BaseSegDataset(BaseDataset):
"""Custom dataset for semantic segmentation. An example of file structure
is as followed.
.. code-block:: none
├── data
│ ├── my_dataset
│ │ ├── img_dir
│ │ │ ├── train
│ │ │ │ ├── xxx{img_suffix}
│ │ │ │ ├── yyy{img_suffix}
│ │ │ │ ├── zzz{img_suffix}
│ │ │ ├── val
│ │ ├── ann_dir
│ │ │ ├── train
│ │ │ │ ├── xxx{seg_map_suffix}
│ │ │ │ ├── yyy{seg_map_suffix}
│ │ │ │ ├── zzz{seg_map_suffix}
│ │ │ ├── val
The img/gt_semantic_seg pair of BaseSegDataset should be of the same
except suffix. A valid img/gt_semantic_seg filename pair should be like
``xxx{img_suffix}`` and ``xxx{seg_map_suffix}`` (extension is also included
in the suffix). If split is given, then ``xxx`` is specified in txt file.
Otherwise, all files in ``img_dir/``and ``ann_dir`` will be loaded.
Please refer to ``docs/en/tutorials/new_dataset.md`` for more details.
Args:
ann_file (str): Annotation file path. Defaults to ''.
metainfo (dict, optional): Meta information for dataset, such as
specify classes to load. Defaults to None.
data_root (str, optional): The root directory for ``data_prefix`` and
``ann_file``. Defaults to None.
data_prefix (dict, optional): Prefix for training data. Defaults to
dict(img_path=None, seg_map_path=None).
img_suffix (str): Suffix of images. Default: '.jpg'
seg_map_suffix (str): Suffix of segmentation maps. Default: '.png'
filter_cfg (dict, optional): Config for filter data. Defaults to None.
indices (int or Sequence[int], optional): Support using first few
data in annotation file to facilitate training/testing on a smaller
dataset. Defaults to None which means using all ``data_infos``.
serialize_data (bool, optional): Whether to hold memory using
serialized objects, when enabled, data loader workers can use
shared RAM from master process instead of making a copy. Defaults
to True.
pipeline (list, optional): Processing pipeline. Defaults to [].
test_mode (bool, optional): ``test_mode=True`` means in test phase.
Defaults to False.
lazy_init (bool, optional): Whether to load annotation during
instantiation. In some cases, such as visualization, only the meta
information of the dataset is needed, which is not necessary to
load annotation file. ``Basedataset`` can skip load annotations to
save time by set ``lazy_init=True``. Defaults to False.
use_label_map (bool, optional): Whether to use label map.
Defaults to False.
max_refetch (int, optional): If ``Basedataset.prepare_data`` get a
None img. The maximum extra number of cycles to get a valid
image. Defaults to 1000.
backend_args (dict, Optional): Arguments to instantiate a file backend.
See https://mmengine.readthedocs.io/en/latest/api/fileio.htm
for details. Defaults to None.
Notes: mmcv>=2.0.0rc4 required.
"""
METAINFO: dict = dict()
def __init__(self,
ann_file: str = '',
img_suffix='.jpg',
seg_map_suffix='.png',
metainfo: Optional[dict] = None,
data_root: Optional[str] = None,
data_prefix: dict = dict(img_path='', seg_map_path=''),
filter_cfg: Optional[dict] = None,
indices: Optional[Union[int, Sequence[int]]] = None,
serialize_data: bool = True,
pipeline: List[Union[dict, Callable]] = [],
test_mode: bool = False,
lazy_init: bool = False,
use_label_map: bool = False,
max_refetch: int = 1000,
backend_args: Optional[dict] = None) -> None:
self.img_suffix = img_suffix
self.seg_map_suffix = seg_map_suffix
self.backend_args = backend_args.copy() if backend_args else None
self.data_root = data_root
self.data_prefix = copy.copy(data_prefix)
self.ann_file = ann_file
self.filter_cfg = copy.deepcopy(filter_cfg)
self._indices = indices
self.serialize_data = serialize_data
self.test_mode = test_mode
self.max_refetch = max_refetch
self.data_list: List[dict] = []
self.data_bytes: np.ndarray
# Set meta information.
self._metainfo = self._load_metainfo(copy.deepcopy(metainfo))
# Get label map for custom classes
new_classes = self._metainfo.get('classes', None)
self.label_map = self.get_label_map(
new_classes) if use_label_map else None
self._metainfo.update(dict(label_map=self.label_map))
# Update palette based on label map or generate palette
# if it is not defined
updated_palette = self._update_palette()
self._metainfo.update(dict(palette=updated_palette))
# Join paths.
if self.data_root is not None:
self._join_prefix()
# Build pipeline.
self.pipeline = Compose(pipeline)
# Full initialize the dataset.
if not lazy_init:
self.full_init()
if test_mode:
assert self._metainfo.get('classes') is not None, \
'dataset metainfo `classes` should be specified when testing'
@classmethod
def get_label_map(cls,
new_classes: Optional[Sequence] = None
) -> Union[Dict, None]:
"""Require label mapping.
The ``label_map`` is a dictionary, its keys are the old label ids and
its values are the new label ids, and is used for changing pixel
labels in load_annotations. If and only if old classes in cls.METAINFO
is not equal to new classes in self._metainfo and nether of them is not
None, `label_map` is not None.
Args:
new_classes (list, tuple, optional): The new classes name from
metainfo. Default to None.
Returns:
dict, optional: The mapping from old classes in cls.METAINFO to
new classes in self._metainfo
"""
old_classes = cls.METAINFO.get('classes', None)
if (new_classes is not None and old_classes is not None
and list(new_classes) != list(old_classes)):
label_map = {}
if not set(new_classes).issubset(cls.METAINFO['classes']):
raise ValueError(
f'new classes {new_classes} is not a '
f'subset of classes {old_classes} in METAINFO.')
for i, c in enumerate(old_classes):
if c not in new_classes:
# 0 is background
label_map[i] = 0
else:
label_map[i] = new_classes.index(c)
return label_map
else:
return None
def _update_palette(self) -> list:
"""Update palette after loading metainfo.
If length of palette is equal to classes, just return the palette.
If palette is not defined, it will randomly generate a palette.
If cla
没有合适的资源?快使用搜索试试~ 我知道了~
matlab 新手说明 包含说明和注释
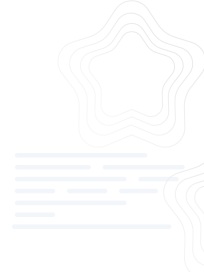
共4个文件
py:3个
zip:1个

需积分: 5 0 下载量 31 浏览量
2024-10-07
09:21:35
上传
评论
收藏 17KB ZIP 举报
温馨提示
matlab
资源推荐
资源详情
资源评论
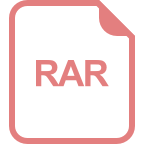
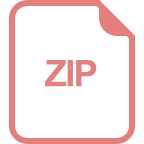
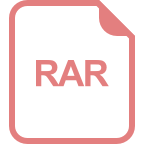
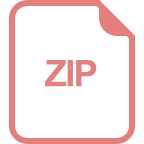
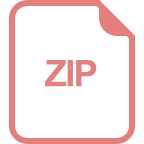
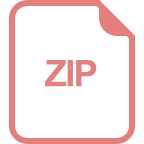
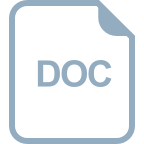
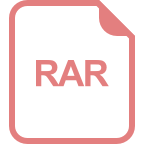
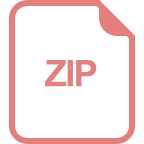
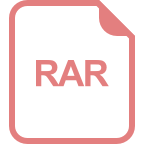
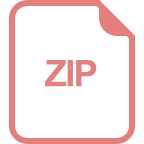
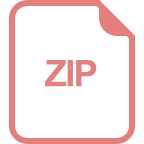
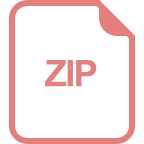
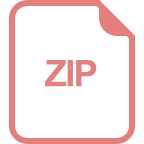
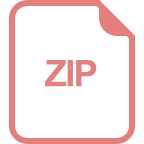
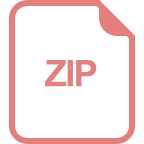
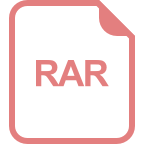
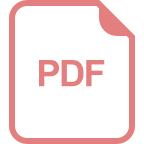
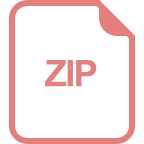
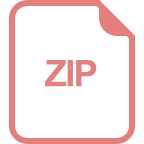
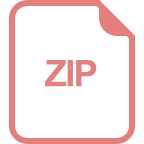
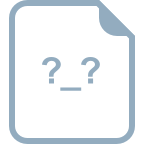
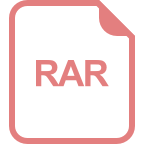
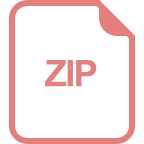
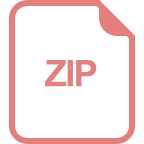
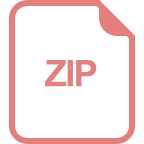
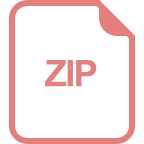
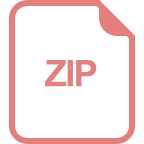
收起资源包目录

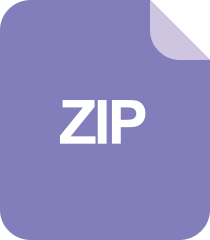
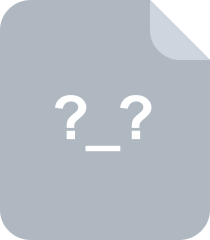
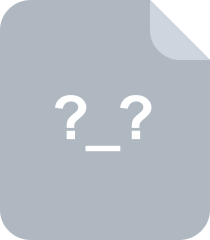
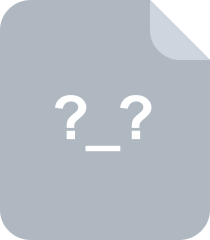
共 4 条
- 1
资源评论
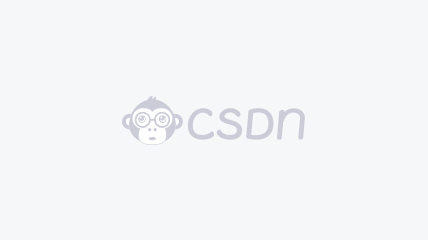

聚财猫猫
- 粉丝: 248
- 资源: 221
上传资源 快速赚钱
我的内容管理 展开
我的资源 快来上传第一个资源
我的收益
登录查看自己的收益我的积分 登录查看自己的积分
我的C币 登录后查看C币余额
我的收藏
我的下载
下载帮助

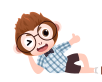
最新资源
资源上传下载、课程学习等过程中有任何疑问或建议,欢迎提出宝贵意见哦~我们会及时处理!
点击此处反馈


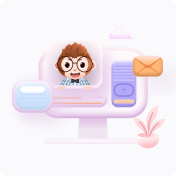
安全验证
文档复制为VIP权益,开通VIP直接复制
