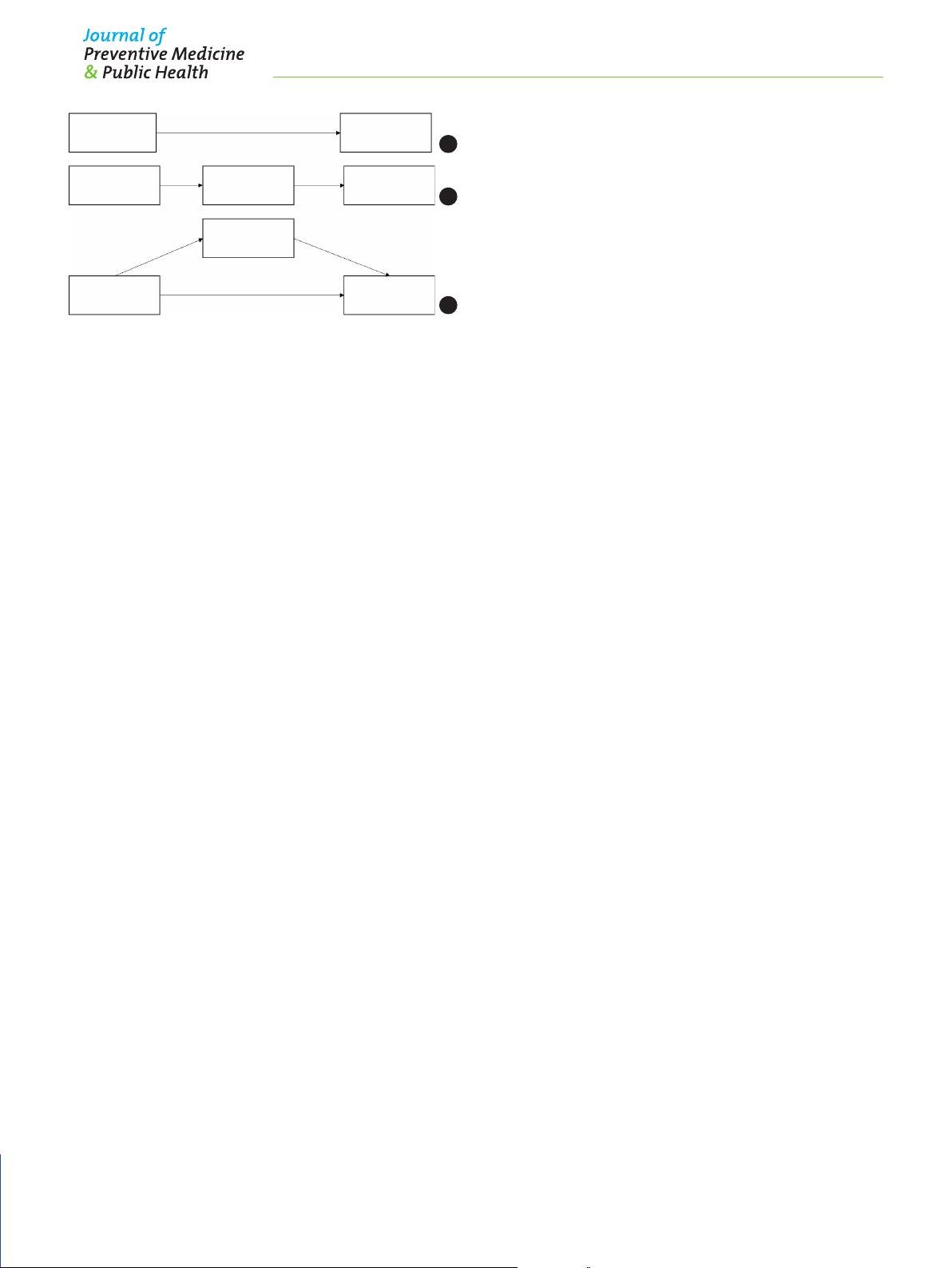
167
Mediation Analysis and Examples
Journal of
Preventive Medicine
& Public Health
166
Copyright © 2021 The Korean Society for Preventive Medicine
cal reports focused on evaluating the simple association be-
tween E and Y as in Figure 1A. However, as in Figure 1B, it is
shown that an E aects a mediator (M), which in turn aects
an Y. The M fully mediates the eect from the E to the Y. How-
ever, situations were identified where the M does not fully
mediate the eect of E on the Y, which led to the concept of
partial mediation, as depicted in Figure 1C. As shown in Figure
1C, the eect of an E can be exerted directly on an Y (direct ef-
fect, path c’) or take a detour via a M (indirect eect, paths a
and b). Initially, the criteria to be regarded as a M were that E
should have a statistically signicant association with M, and
that M should also have a statistically significant association
with Y. The initial criteria also included the condition that the
mediation analysis could be performed only if there was a sta-
tistically signicant association between E and Y; this signi-
cant relationship between E and Y should be no longer signi-
cant after controlling for the previous paths from E to M and M
to Y. However, the latter two conditions were further criticized
due to the existence of inconsistent and partial mediation, and
were therefore omitted from the essential conditions needed
for mediation analysis.
In contrast to a moderator or confounder, a M is interpreted
as involving a causal pathway between E and Y. A detailed def-
inition of a M is provided in the work of Robins and Greenland
[7]. The seminal work on this concept of a M or intervening
variable was based on Judd and Kenny [8,9] and Baron and
Kenny [10]’s article utilizing the regression method.
In Judd and Kenny[8,9]’s dierence of coecients approach,
mediation analysis can be conceptualized as utilizing two re-
gressions, as follows. First, we run a simple regression analysis
with E on Y without M to estimate path c’.
Y
=
B
0
+B · E+e
(1)
Second, we carry out a multivariable regression with E and
M to predict Y.
Y
=
B
0
+B
1
· E+B
2
· M+e
(2)
In this case, as the coecient B reects the total eect (TE),
the direct eect from the E to Y c’ shown in Figure 1C, corre-
sponds to B
1
in equation 2. The dierence method calculates
the indirect eect by subtracting the direct eect (c’) from the
TE, as follows:
B
indirect
=
B−B
1
(3)
This is a simple and widely used approach to screen for the
possible presence of a M. However, the logistic regression
method has been criticized for lacking a causal interpretation.
The dierence method has been used to check for mediation,
but non-signicant ndings using this method do not exclude
the chance of possible mediation [11].
The other approach is the product method, which was intro-
duced by Sobel and used by Baron and Kenny [10]. In this meth-
od, again, a multivariable regression is conducted with E and
M to predict Y.
Y
=
B
0
+B
1
· E+B
2
· M+e
(2)
However, the next step is to regress M on X and can be writ-
ten as
M
=
B
0
+B · X+e
(4)
In equation 3, B reects path a in Figure 1C, and B
2
in equa-
tion 2 reects b in Figure 1C. The coecient of the indirect ef-
fect, B
indirect
, is calculated by multiplying the 2 coecients, B
2
and B.
B
indirect
=
B·B
2
(5)
Generally, when there is no interaction between an E and a
M, these two methods coincide, except for logistic regression.
In particular, for rare Ys (approximately under 10%) with no
confounding factors, these 2 estimates will, from a practical
standpoint, reect the natural indirect eect (NIE), which will
be discussed in the causal mediation section. The difference
Figure 1. A conceptual diagram of mediation analysis (A) tra-
ditional epidemiological assessment, (B) full mediation, and
(C) partial mediation.
Exposure
Exposure Mediator
Mediator
c
c’
a
a
b
b
Outcome
Outcome
Outcome
Exposure
A
B
C