/*
Copyright (c) 2011-2013, ESN Social Software AB and Jonas Tarnstrom
All rights reserved.
Redistribution and use in source and binary forms, with or without
modification, are permitted provided that the following conditions are met:
* Redistributions of source code must retain the above copyright
notice, this list of conditions and the following disclaimer.
* Redistributions in binary form must reproduce the above copyright
notice, this list of conditions and the following disclaimer in the
documentation and/or other materials provided with the distribution.
* Neither the name of the ESN Social Software AB nor the
names of its contributors may be used to endorse or promote products
derived from this software without specific prior written permission.
THIS SOFTWARE IS PROVIDED BY THE COPYRIGHT HOLDERS AND CONTRIBUTORS "AS IS" AND
ANY EXPRESS OR IMPLIED WARRANTIES, INCLUDING, BUT NOT LIMITED TO, THE IMPLIED
WARRANTIES OF MERCHANTABILITY AND FITNESS FOR A PARTICULAR PURPOSE ARE
DISCLAIMED. IN NO EVENT SHALL ESN SOCIAL SOFTWARE AB OR JONAS TARNSTROM BE
LIABLE FOR ANY DIRECT, INDIRECT, INCIDENTAL, SPECIAL, EXEMPLARY, OR
CONSEQUENTIAL DAMAGES (INCLUDING, BUT NOT LIMITED TO, PROCUREMENT OF SUBSTITUTE
GOODS OR SERVICES; LOSS OF USE, DATA, OR PROFITS; OR BUSINESS INTERRUPTION)
HOWEVER CAUSED AND ON ANY THEORY OF LIABILITY, WHETHER IN CONTRACT, STRICT
LIABILITY, OR TORT (INCLUDING NEGLIGENCE OR OTHERWISE) ARISING IN ANY WAY OUT OF
THE USE OF THIS SOFTWARE, EVEN IF ADVISED OF THE POSSIBILITY OF SUCH DAMAGE.
Portions of code from MODP_ASCII - Ascii transformations (upper/lower, etc)
https://github.com/client9/stringencoders
Copyright (c) 2007 Nick Galbreath -- nickg [at] modp [dot] com. All rights
reserved.
Numeric decoder derived from TCL library
https://www.opensource.apple.com/source/tcl/tcl-14/tcl/license.terms
* Copyright (c) 1988-1993 The Regents of the University of California.
* Copyright (c) 1994 Sun Microsystems, Inc.
*/
// Licence at LICENSES/ULTRAJSON_LICENSE
#define PY_SSIZE_T_CLEAN
#include <Python.h>
#define NO_IMPORT_ARRAY
#define PY_ARRAY_UNIQUE_SYMBOL UJSON_NUMPY
#include "datetime.h"
#include "pandas/datetime/pd_datetime.h"
#include "pandas/vendored/ujson/lib/ultrajson.h"
#include <numpy/arrayobject.h>
#include <numpy/arrayscalars.h>
#include <numpy/ndarraytypes.h>
#include <numpy/npy_math.h>
npy_int64 get_nat(void) { return NPY_MIN_INT64; }
typedef char *(*PFN_PyTypeToUTF8)(JSOBJ obj, JSONTypeContext *ti,
size_t *_outLen);
int object_is_decimal_type(PyObject *obj);
int object_is_dataframe_type(PyObject *obj);
int object_is_series_type(PyObject *obj);
int object_is_index_type(PyObject *obj);
int object_is_nat_type(PyObject *obj);
int object_is_na_type(PyObject *obj);
typedef struct __NpyArrContext {
PyObject *array;
char *dataptr;
npy_intp curdim; // current dimension in array's order
npy_intp stridedim; // dimension we are striding over
int inc; // stride dimension increment (+/- 1)
npy_intp dim;
npy_intp stride;
npy_intp ndim;
npy_intp index[NPY_MAXDIMS];
int type_num;
char **rowLabels;
char **columnLabels;
} NpyArrContext;
typedef struct __PdBlockContext {
Py_ssize_t colIdx;
Py_ssize_t ncols;
int transpose;
NpyArrContext **npyCtxts; // NpyArrContext for each column
} PdBlockContext;
typedef struct __TypeContext {
JSPFN_ITERBEGIN iterBegin;
JSPFN_ITEREND iterEnd;
JSPFN_ITERNEXT iterNext;
JSPFN_ITERGETNAME iterGetName;
JSPFN_ITERGETVALUE iterGetValue;
PFN_PyTypeToUTF8 PyTypeToUTF8;
PyObject *newObj;
PyObject *dictObj;
Py_ssize_t index;
Py_ssize_t size;
PyObject *itemValue;
PyObject *itemName;
PyObject *attrList;
PyObject *iterator;
double doubleValue;
JSINT64 longValue;
char *cStr;
NpyArrContext *npyarr;
PdBlockContext *pdblock;
int transpose;
char **rowLabels;
char **columnLabels;
npy_intp rowLabelsLen;
npy_intp columnLabelsLen;
} TypeContext;
typedef struct __PyObjectEncoder {
JSONObjectEncoder enc;
// pass through the NpyArrContext when encoding multi-dimensional arrays
NpyArrContext *npyCtxtPassthru;
// pass through the PdBlockContext when encoding blocks
PdBlockContext *blkCtxtPassthru;
// pass-through to encode numpy data directly
int npyType;
void *npyValue;
int datetimeIso;
NPY_DATETIMEUNIT datetimeUnit;
NPY_DATETIMEUNIT valueUnit;
// output format style for pandas data types
int outputFormat;
int originalOutputFormat;
PyObject *defaultHandler;
} PyObjectEncoder;
#define GET_TC(__ptrtc) ((TypeContext *)((__ptrtc)->prv))
enum PANDAS_FORMAT { SPLIT, RECORDS, INDEX, COLUMNS, VALUES };
static int PdBlock_iterNext(JSOBJ, JSONTypeContext *);
static TypeContext *createTypeContext(void) {
TypeContext *pc = PyObject_Malloc(sizeof(TypeContext));
if (!pc) {
PyErr_NoMemory();
return NULL;
}
pc->newObj = NULL;
pc->dictObj = NULL;
pc->itemValue = NULL;
pc->itemName = NULL;
pc->attrList = NULL;
pc->index = 0;
pc->size = 0;
pc->longValue = 0;
pc->doubleValue = 0.0;
pc->cStr = NULL;
pc->npyarr = NULL;
pc->pdblock = NULL;
pc->rowLabels = NULL;
pc->columnLabels = NULL;
pc->transpose = 0;
pc->rowLabelsLen = 0;
pc->columnLabelsLen = 0;
return pc;
}
static PyObject *get_values(PyObject *obj) {
PyObject *values = NULL;
if (object_is_index_type(obj) || object_is_series_type(obj)) {
// The special cases to worry about are dt64tz and category[dt64tz].
// In both cases we want the UTC-localized datetime64 ndarray,
// without going through and object array of Timestamps.
if (PyObject_HasAttrString(obj, "tz")) {
PyObject *tz = PyObject_GetAttrString(obj, "tz");
if (tz != Py_None) {
// Go through object array if we have dt64tz, since tz info will
// be lost if values is used directly.
Py_DECREF(tz);
values = PyObject_CallMethod(obj, "__array__", NULL);
return values;
}
Py_DECREF(tz);
}
values = PyObject_GetAttrString(obj, "values");
if (values == NULL) {
// Clear so we can subsequently try another method
PyErr_Clear();
} else if (PyObject_HasAttrString(values, "__array__")) {
// We may have gotten a Categorical or Sparse array so call np.array
PyObject *array_values = PyObject_CallMethod(values, "__array__", NULL);
Py_DECREF(values);
values = array_values;
} else if (!PyArray_CheckExact(values)) {
// Didn't get a numpy array, so keep trying
Py_DECREF(values);
values = NULL;
}
}
if (values == NULL) {
PyObject *typeRepr = PyObject_Repr((PyObject *)Py_TYPE(obj));
PyObject *repr;
if (PyObject_HasAttrString(obj, "dtype")) {
PyObject *dtype = PyObject_GetAttrString(obj, "dtype");
repr = PyObject_Repr(dtype);
Py_DECREF(dtype);
} else {
repr = PyUnicode_FromString("<unknown dtype>");
}
PyErr_Format(PyExc_ValueError, "%R or %R are not JSON serializable yet",
repr, typeRepr);
Py_DECREF(repr);
Py_DECREF(typeRepr);
return NULL;
}
return values;
}
static PyObject *get_sub_attr(PyObject *obj, char *attr, char *subAttr) {
PyObject *tmp = PyObject_GetAttrString(obj, attr);
if (tmp == 0) {
return 0;
}
PyObject *ret = PyObject_GetAttrString(tmp, subAttr);
Py_DECREF(tmp);
return ret;
}
static Py_ssize_t get_attr_length(PyObject *obj, char *attr) {
PyObject *tmp = PyObject_GetAttrString(obj, attr);
if (tmp == 0) {
return 0;
}
Py_ssize_t ret = PyObject_Length(tmp);
Py_DECREF(tmp);
if (ret == -1) {
return 0;
}
return ret;
}
static npy_int64 get_long_attr(PyObject *o, const char *attr) {
// NB we are implicitly assuming that o is a Timedelta or Timestamp, or NaT
PyObject *value = PyObject_GetAttrString(o, attr);
const npy_int64 long_val =
(PyLong_Check(value) ? PyLong_AsLongLong(value) : PyLong_AsLong(value));
Py_DECREF(value);
if (obj

执着的小火车
- 粉丝: 467
- 资源: 1186
最新资源
- C#联合halcon的demo 直线 找圆 形状模板匹配及等级识别等功能 功能有找直线,找圆,形状模板匹配,二维码识别及等级识别,相机内参标定,相机外参标定,以及几何测量 另外还有某论坛的开源控件,并
- BM260、310、410系列.pdf
- P9500系列.pdf
- 西门子PID程序西门子plc模板程序西门子通讯程序案例 1200和多台G120西门子变频器Modbud RTU通讯,带西门子触摸屏,带变频器参数 Modbus通讯报文详细讲解,PID自写FB块无密
- NOI级 数学与其他-2025.01.09(K).pdf
- 毕业设计-基于python大学生就业信息管理系统(django)毕业设计与实现源码+数据库
- 电机控制器,感应异步电机的无传感器矢量控制,完整的C代码+仿真模型: 基于“电压模型+电流模型”的磁链观测器,实现转子磁场定向控制(FOC),可实现电机在低速、中高速段的高精度的转速估算;代码已经成功
- Scrum指南-中文版-2020
- 探索CDN技术:互联网内容加速的分布式解决方案
- Hadoop环境中MapReduce集群的操作命令与Web管理界面介绍
- 西门子PID程序西门子PLC 1200和多台G120西门子变频器Modbud RTU通讯,带西门子触摸屏,带变频器参数 Modbus通讯报文详细讲解,PID自写FB块无密码可以直接应用到程序,PID带
- 大数据处理中PySpark操作与实战案例:RDD创建及基本操作教程
- 毕业设计-基于python招聘数据分析可视化系统(django)毕业设计与实现源码+数据库
- GEE 案例-基于sentinel-2的主成分分析(查看不同波段的主成分结果).pdf
- SUES-大四上-计科课程-学习/考试/复习/实验资料
- 基于分布式驱动电动汽车的车辆状态估计,分别采用无迹卡尔曼,容积卡尔曼,高阶容积卡尔曼观测器等,可估计包括纵向速度,质心侧偏角,横摆角速度,以及四个车轮角速度七个状态 模型中第一个模块是四轮驱动电机
资源上传下载、课程学习等过程中有任何疑问或建议,欢迎提出宝贵意见哦~我们会及时处理!
点击此处反馈


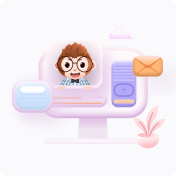