Matlab Toolbox for Dimensionality Reduction (v0.8.1b)
=====================================================
Information
-------------------------
Author: Laurens van der Maaten
Affiliation: Delft University of Technology
Contact: lvdmaaten@gmail.com
Release date: March 21, 2013
Version: 0.8.1b
Installation
-------------------------
Copy the drtoolbox/ folder into the $MATLAB_DIR/toolbox directory (where $MATLAB_DIR indicates your Matlab installation directory). Start Matlab and select 'Set path...' from the File menu. Click the 'Add with subfolders...' button, select the folder $MATLAB_DIR/toolbox/drtoolbox in the file dialog, and press Open. Subsequently, press the Save button in order to save your changes to the Matlab search path. The toolbox is now installed.
Some of the functions in the toolbox use MEX-files. Precompiled versions of these MEX-files are distributed with this release, but the compiled version for your platform might be missing. In order to compile all MEX-files, type cd([matlabroot '/toolbox/drtoolbox']) in your Matlab prompt, and execute the function MEXALL.
Features
-------------------------
This Matlab toolbox implements 34 techniques for dimensionality reduction and metric learning. These techniques are all available through the COMPUTE_MAPPING function or through the GUI. The following techniques are available:
- Principal Component Analysis ('PCA')
- Linear Discriminant Analysis ('LDA')
- Multidimensional scaling ('MDS')
- Probabilistic PCA ('ProbPCA')
- Factor analysis ('FactorAnalysis')
- Sammon mapping ('Sammon')
- Isomap ('Isomap')
- Landmark Isomap ('LandmarkIsomap')
- Locally Linear Embedding ('LLE')
- Laplacian Eigenmaps ('Laplacian')
- Hessian LLE ('HessianLLE')
- Local Tangent Space Alignment ('LTSA')
- Diffusion maps ('DiffusionMaps')
- Kernel PCA ('KernelPCA')
- Generalized Discriminant Analysis ('KernelLDA')
- Stochastic Neighbor Embedding ('SNE')
- Symmetric Stochastic Neighbor Embedding ('SymSNE')
- t-Distributed Stochastic Neighbor Embedding ('tSNE')
- Neighborhood Preserving Embedding ('NPE')
- Locality Preserving Projection ('LPP')
- Stochastic Proximity Embedding ('SPE')
- Linear Local Tangent Space Alignment ('LLTSA')
- Conformal Eigenmaps ('CCA', implemented as an extension of LLE)
- Maximum Variance Unfolding ('MVU', implemented as an extension of LLE)
- Landmark Maximum Variance Unfolding ('LandmarkMVU')
- Fast Maximum Variance Unfolding ('FastMVU')
- Locally Linear Coordination ('LLC')
- Manifold charting ('ManifoldChart')
- Coordinated Factor Analysis ('CFA')
- Gaussian Process Latent Variable Model ('GPLVM')
- Deep autoencoders ('Autoencoder')
- Neighborhood Components Analysis ('NCA')
- Maximally Collapsing Metric Learning ('MCML')
- Large Margin Nearest Neighhbor metric learning ('LMNN')
Furthermore, the toolbox contains 6 techniques for intrinsic dimensionality estimation. These techniques are available through the function INTRINSIC_DIM. The following techniques are available:
- Eigenvalue-based estimation ('EigValue')
- Maximum Likelihood Estimator ('MLE')
- Estimator based on correlation dimension ('CorrDim')
- Estimator based on nearest neighbor evaluation ('NearNb')
- Estimator based on packing numbers ('PackingNumbers')
- Estimator based on geodesic minimum spanning tree ('GMST')
In addition to these techniques, the toolbox contains functions for prewhitening of data (the function PREWHITEN), exact and estimate out-of-sample extension (the functions OUT_OF_SAMPLE and OUT_OF_SAMPLE_EST), and a function that generates toy datasets (the function GENERATE_DATA).
The graphical user interface of the toolbox is accessible through the DRGUI function.
Usage
-------------------------
All the functions that you should call as a user of the toolbox are located in the same folder as this Readme-file. The folder contains the following files:
- compute_mapping.m This function performs the specified dimension reduction technique on the specified data set. Type HELP COMPUTE_MAPPING to get details on supported techniques and on the parameters of the techniques.
- drgui.m This function allows you to use some of the toolbox functionality via a graphical user interface.
- generate_data.m This function generates some artificial data sets such as the Swiss roll data set.
- intrinsic_dim.m This function performs intrinsic dimensionality estimation using the specified estimator on the specified data set.
- mexall.m This function compiles all the MEX-files that are required to use the toolbox. Please run immediately after installation.
- out_of_sample.m This function takes as input a dimension reduction mapping and a set of new test points, and outputs the locations of the test points in the reduced space. This function is only supported by parametric and spectral techniques.
- out_of_sample_est.m This function takes as input a training set, a reduced version of that training set, and a set of new test points, and finds an approximate locations of the test points in the reduced space. Only use this function for techniques that do not support out-of-sample-extensions.
- prewhiten.m This function whitens data, i.e., it makes it zero-mean, identity-covariance.
- reconstruct_data.m This function computes reconstructions of reduced data for linear techniques and autoencoders.
- test_toolbox.m This function runs a full test of all functionalities of the toolbox.
Here is an example on how to use the toolbox:
[X, labels] = generate_data('helix', 2000);
figure, scatter3(X(:,1), X(:,2), X(:,3), 5, labels); title('Original dataset'), drawnow
no_dims = round(intrinsic_dim(X, 'MLE'));
disp(['MLE estimate of intrinsic dimensionality: ' num2str(no_dims)]);
[mappedX, mapping] = compute_mapping(X, 'PCA', no_dims);
figure, scatter(mappedX(:,1), mappedX(:,2), 5, labels); title('Result of PCA');
[mappedX, mapping] = compute_mapping(X, 'Laplacian', no_dims, 7);
figure, scatter(mappedX(:,1), mappedX(:,2), 5, labels(mapping.conn_comp)); title('Result of Laplacian Eigenmaps'); drawnow
It will create a helix dataset, estimate the intrinsic dimensionality of the dataset, run Laplacian Eigenmaps on the dataset, and plot the results. All functions in the toolbox can work both on data matrices as on PRTools datasets (http://prtools.org). For more information on the options for dimensionality reduction, type HELP COMPUTE_MAPPING in your Matlab prompt. Information on the intrinsic dimensionality estimators can be obtained by typing the HELP INTRINSIC_DIM.
Pitfalls
-------------------------
When you run certain code, you might receive an error that a certain file is missing. This is because in some parts of the code, MEX-functions are used. I provide a number of precompiled versions of these MEX-functions in the toolbox. However, the MEX-file for your platform might be missing. To fix this, type in your Matlab:
mexall
Now you have compiled versions of the MEX-files as well. This fix also solves slow execution of the shortest path computations in Isomap.
If you encounter an error concerning CSDP while running the FastMVU-algorithm, the binary of CSDP for your platform is missing. If so, please obtain a binary distribution of CSDP from https://projects.coin-or.org/Csdp/ and place it in the drtoolbox/techniques directory. Make sure it has the right name for your platform (csdp.exe for Windows, csdpmac for Mac OS X (PowerPC), csdpmaci for Mac OS X (Intel), and csdplinux for Linux).
Many methods for dimensionality reduction perform spectral analyses of sparse matrices. You might think that eigenanalysis is a well-studied problem that can easily be solved. However, eigenanalysis of large matrices turns out to be tedious. The toolbox allows you to use two different methods for eigenanalysis:
- The original Matlab functions (based on Arnoldi methods)
- The JDQR functions (based on Jacobi-Davidson methods)
For problems up to 10,000 data poin
没有合适的资源?快使用搜索试试~ 我知道了~
温馨提示
基于matlab+C/C++实现的K-means+FCM+谱聚类+DBSCAN+AP+DPC聚类算法比较+源码,适合毕业设计、课程设计、项目开发。项目源码已经过严格测试,可以放心参考并在此基础上延申使用,详情见md文档~ 基于matlab+C/C++实现的K-means+FCM+谱聚类+DBSCAN+AP+DPC聚类算法比较+源码,适合毕业设计、课程设计、项目开发。项目源码已经过严格测试,可以放心参考并在此基础上延申使用,详情见md文档~ 基于matlab+C/C++实现的K-means+FCM+谱聚类+DBSCAN+AP+DPC聚类算法比较+源码,适合毕业设计、课程设计、项目开发。项目源码已经过严格测试,可以放心参考并在此基础上延申使用,详情见md文档~ 基于matlab+C/C++实现的K-means+FCM+谱聚类+DBSCAN+AP+DPC聚类算法比较+源码,适合毕业设计、课程设计、项目开发。项目源码已经过严格测试,可以放心参考并在此基础上延申使用,详情见md文档~
资源推荐
资源详情
资源评论
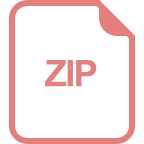
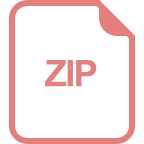
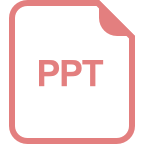
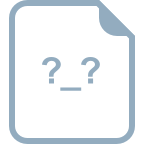
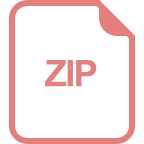
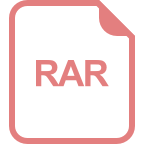
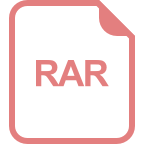
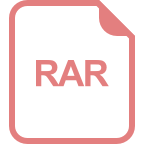
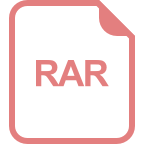
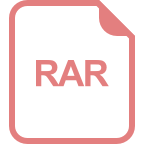
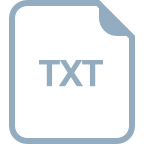
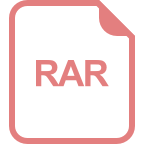
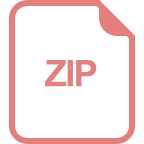
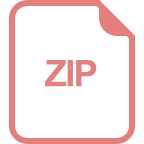
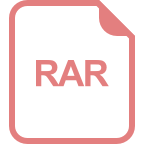
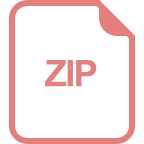
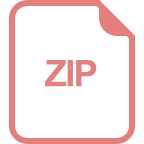
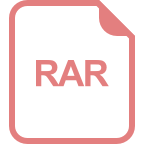
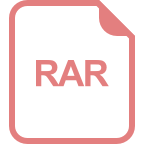
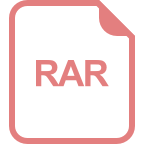
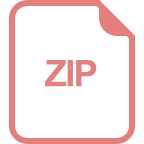
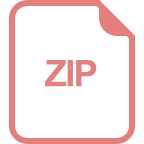
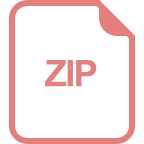
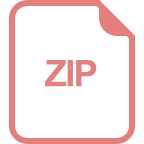
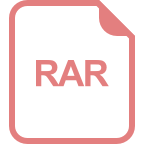
收起资源包目录

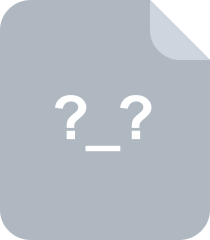
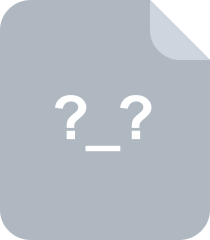
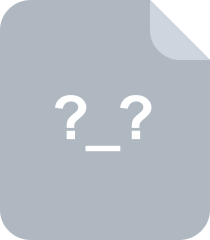
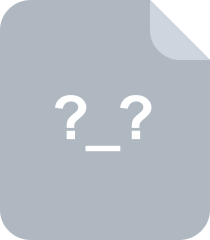
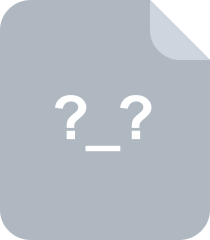
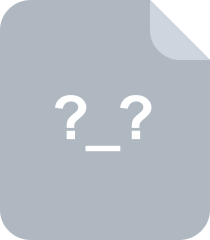
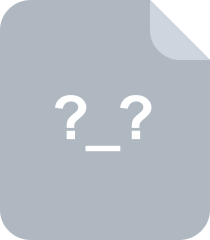
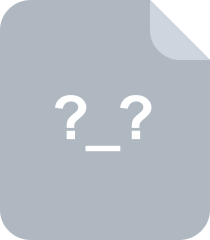
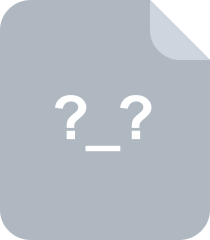
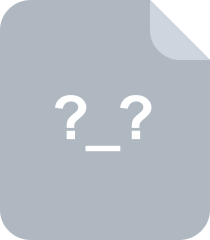
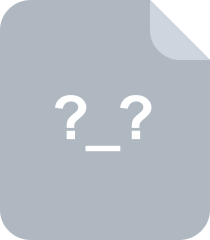
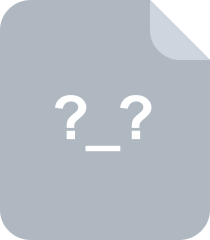
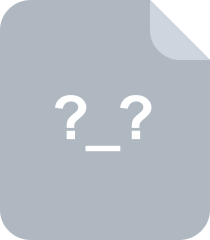
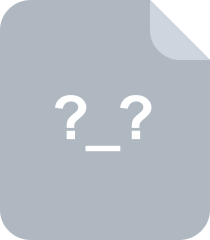
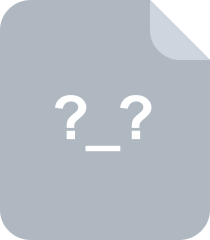
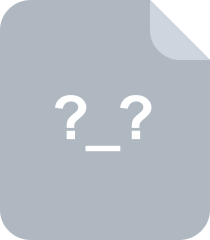
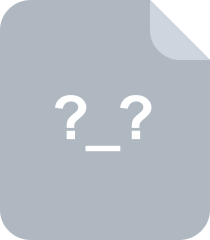
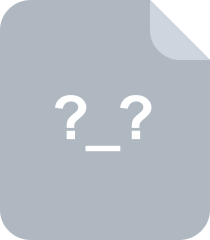
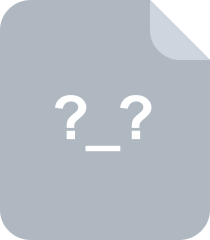
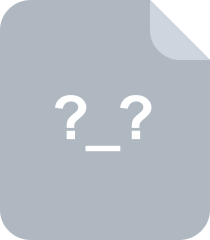
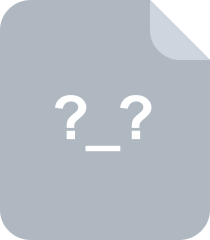
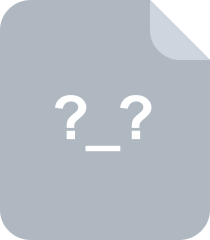
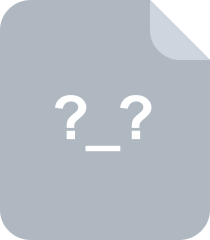
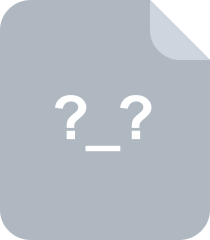
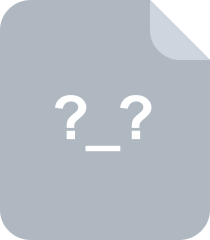
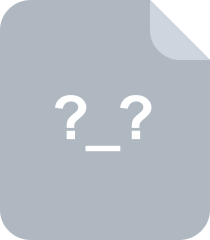
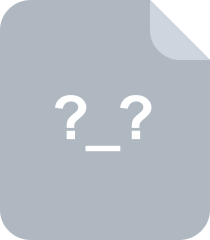
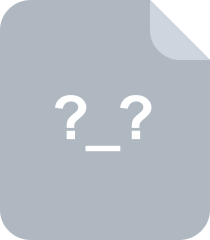
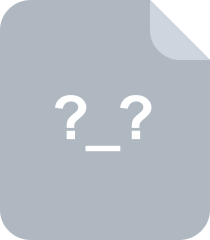
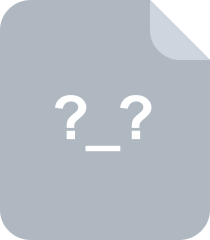
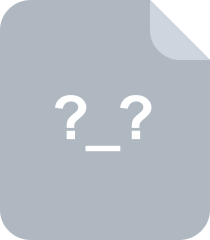
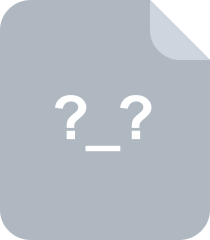
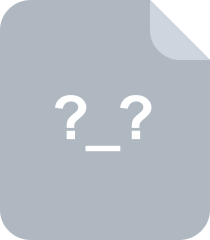
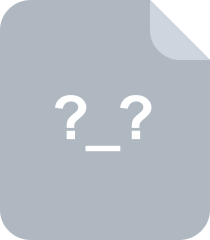
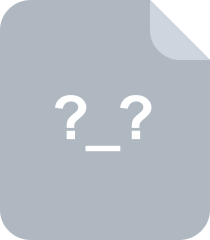
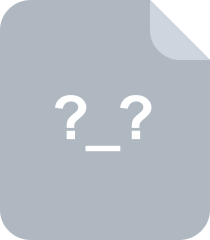
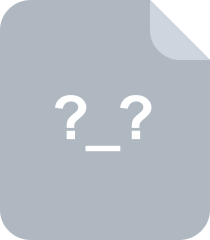
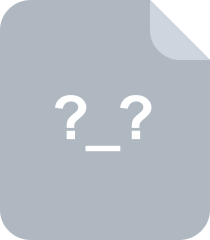
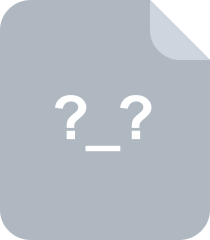
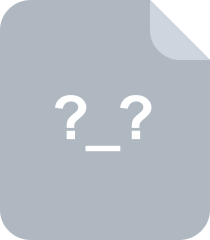
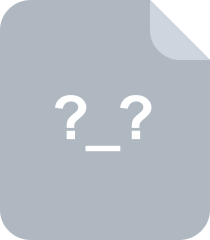
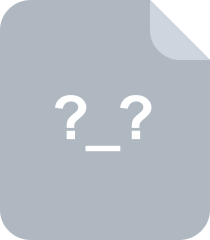
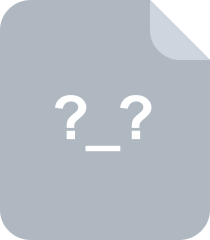
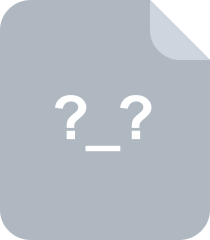
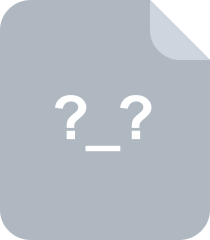
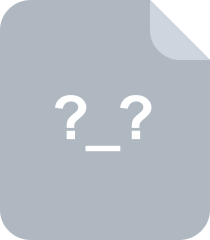
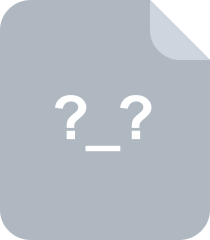
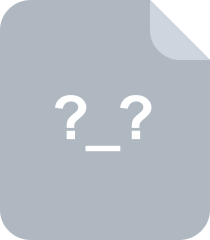
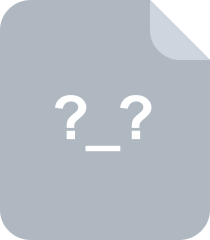
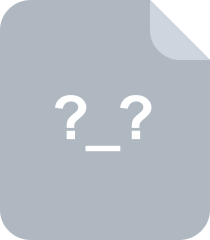
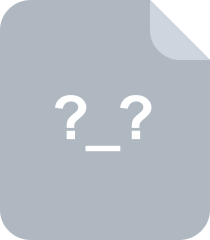
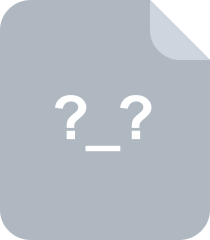
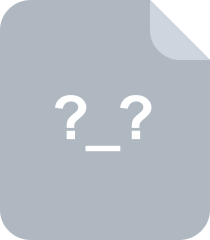
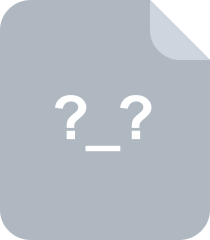
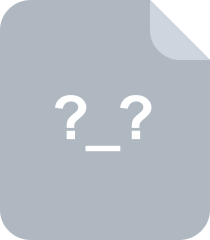
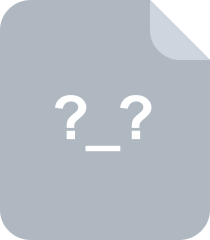
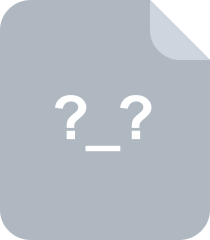
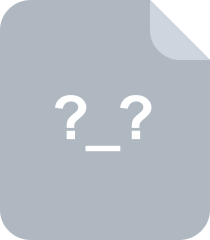
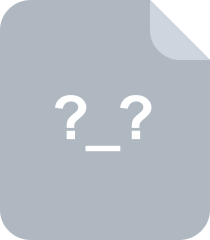
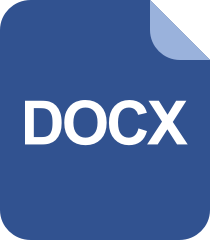
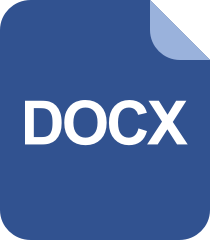
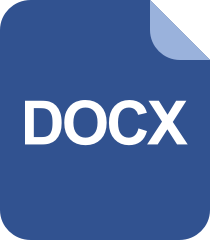
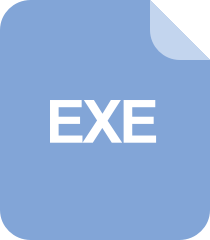
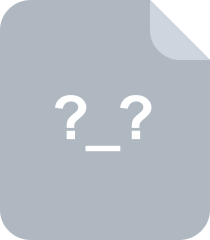
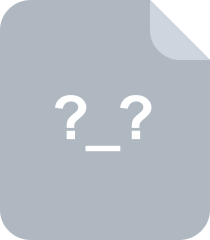
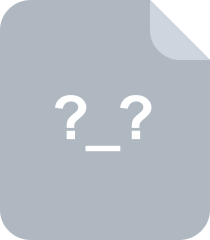
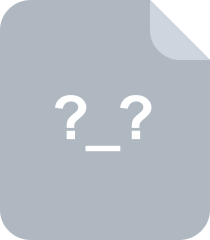
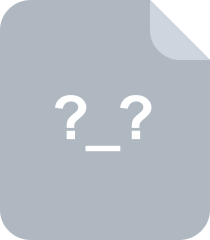
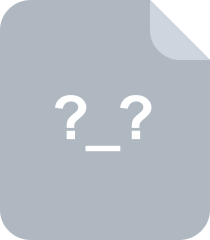
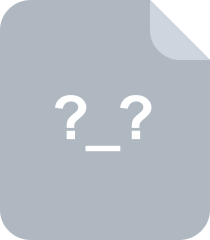
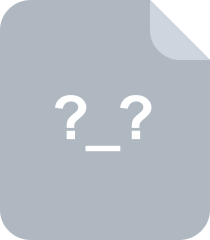
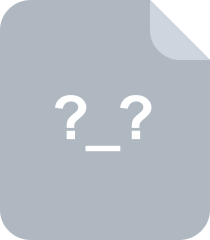
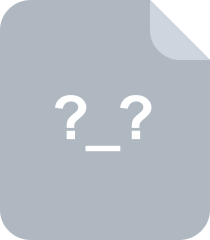
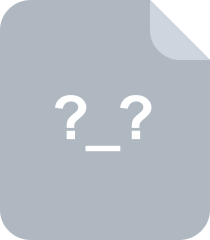
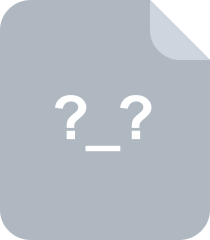
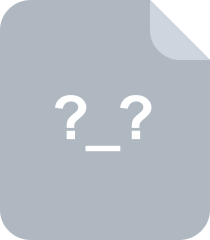
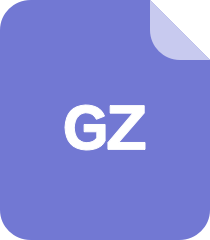
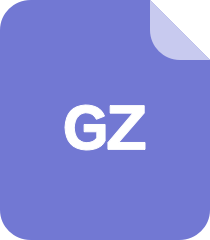
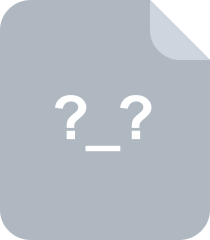
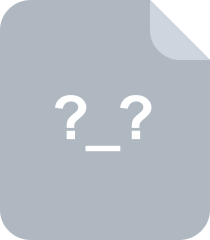
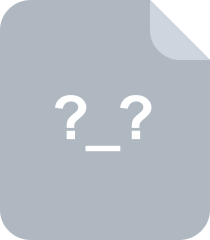
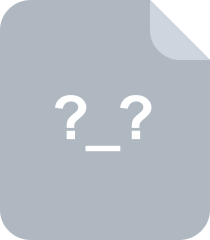
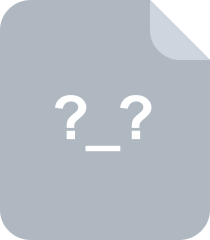
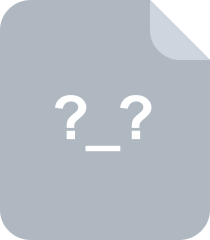
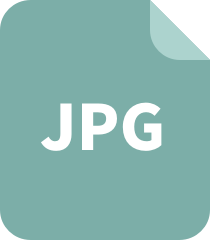
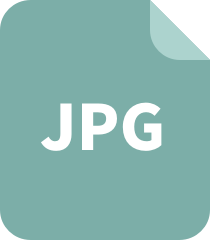
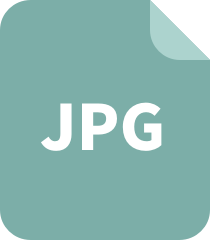
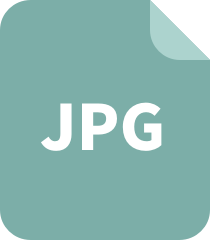
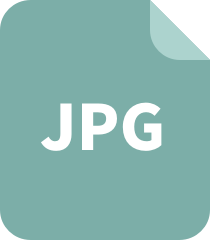
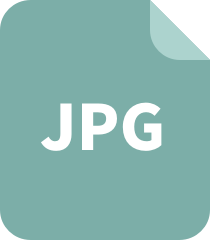
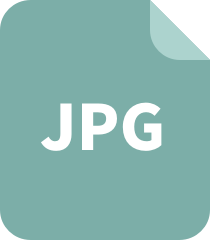
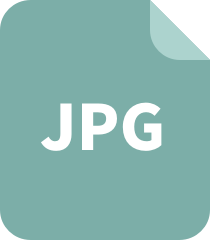
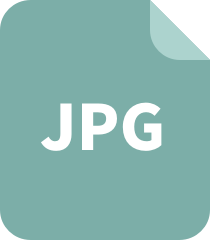
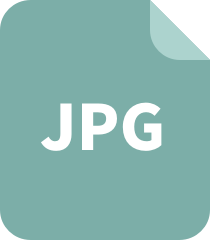
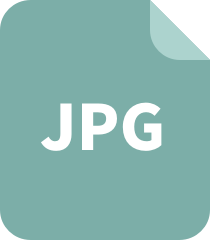
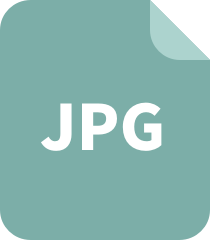
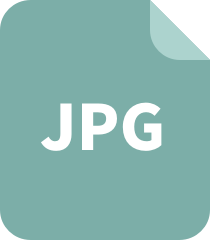
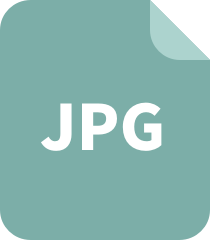
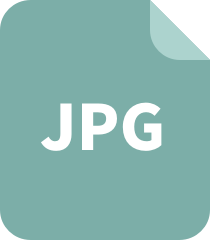
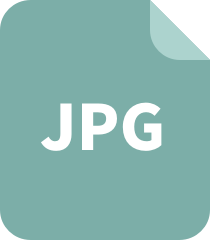
共 463 条
- 1
- 2
- 3
- 4
- 5
资源评论
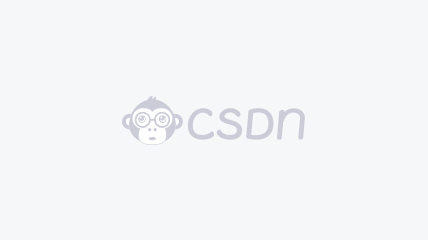
- weixin_512723492024-11-04资源有很好的参考价值,总算找到了自己需要的资源啦。


梦回阑珊
- 粉丝: 5194
- 资源: 1681
上传资源 快速赚钱
我的内容管理 展开
我的资源 快来上传第一个资源
我的收益
登录查看自己的收益我的积分 登录查看自己的积分
我的C币 登录后查看C币余额
我的收藏
我的下载
下载帮助

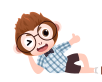
最新资源
资源上传下载、课程学习等过程中有任何疑问或建议,欢迎提出宝贵意见哦~我们会及时处理!
点击此处反馈


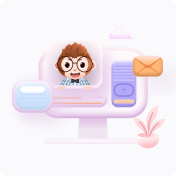
安全验证
文档复制为VIP权益,开通VIP直接复制
