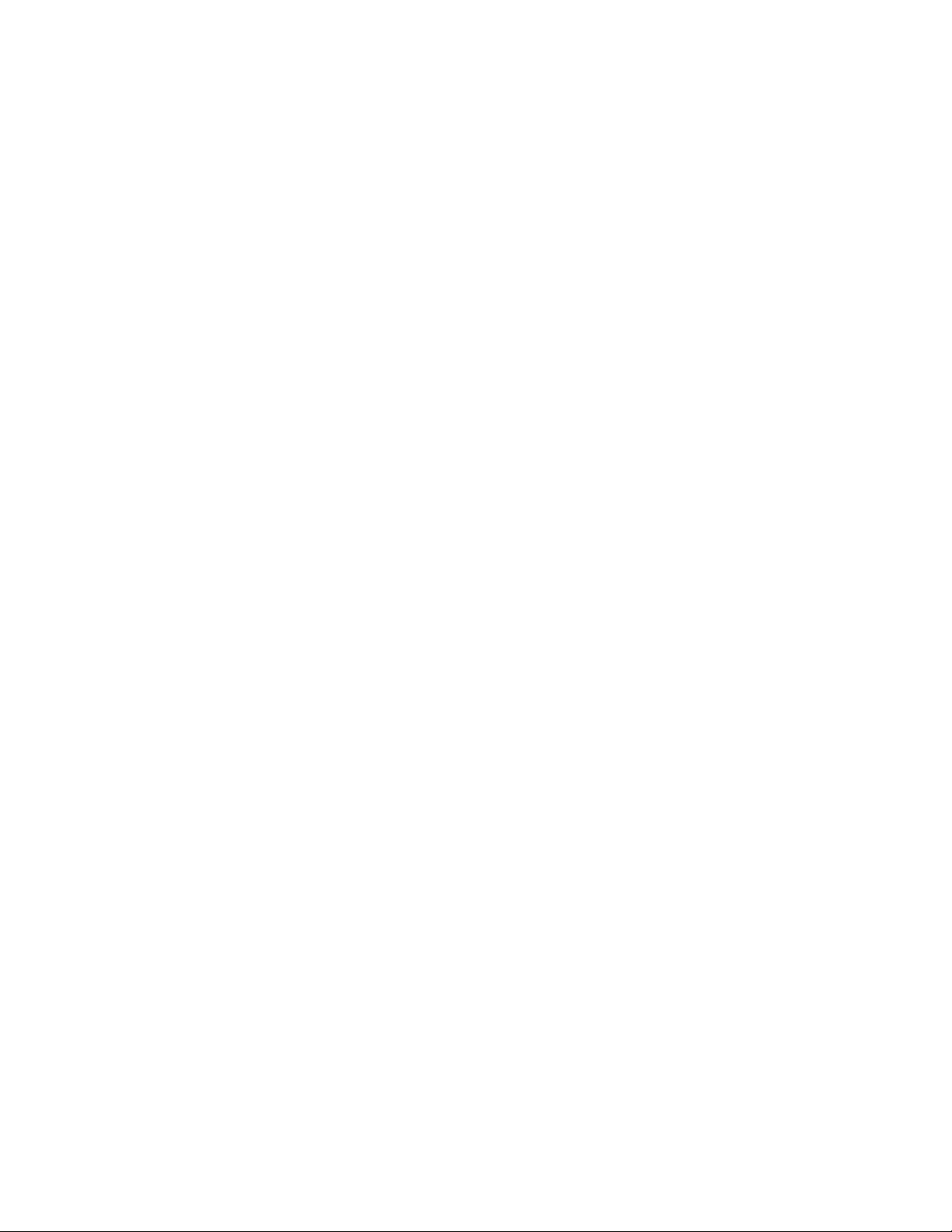
Mean Shift: A Robust Approach
Toward Feature Space Analysis
Dorin Comaniciu, Member, IEEE, and Peter Meer, Senior Member, IEEE
AbstractÐA general nonparametric technique is proposed for the analysis of a complex multimodal feature space and to delineate
arbitrarily shaped clusters in it. The basic computational module of the technique is an old pattern recognition procedure, the mean
shift. We prove for discrete data the convergence of a recursive mean shift procedure to the nearest stationary point of the underlying
density function and, thus, its utility in detecting the modes of the density. The relation of the mean shift procedure to the Nadaraya-
Watson estimator from kernel regression and the robust M-estimators of location is also established. Algorithms for two low-level vision
tasks, discontinuity preserving smoothing and image segmentation, are described as applications. In these algorithms, the only user
set parameter is the resolution of the analysis and either gray level or color images are accepted as input. Extensive experimental
results illustrate their excellent performance.
Index TermsÐMean shift, clustering, image segmentation, image smoothing, feature space, low-level vision.
æ
1INTRODUCTION
L
OW-LEVEL computer vision tasks are misleadingly diffi-
cult. Incorrect results can be easily obtained since the
employed techniques often rely upon the user correctly
guessing the values for the tuning parameters. To improve
performance, the execution of low-level tasks should be task
driven, i.e., supported by independent high-level informa-
tion. This approach, however, requires that, first, the low-
level stage provides a reliable enough representation of the
input and that the feature extraction process be controlled
only by very few tuning parameters corresponding to
intuitive measures in the input domain.
Feature space-based analysis of images is a paradigm
which can achieve the above-stated goals. A feature space is
a mapping of the input obtained through the processing of
the data in small subsets at a time. For each subset, a
parametric representation of the feature of interest is
obtained and the result is mapped into a point in the
multidimensional space of the parameter. After the entire
input is processed, significant features correspond to denser
regions in the feature space, i.e., to clusters, and the goal of
the analysis is the delineation of these clusters.
The nature of the feature space is application dependent.
The subsets employed in the mapping can range from
individual pixels, as in the color space representation of an
image, to a set of quasi-randomly chosen data points, as in
the probabilistic Hough transform. Both the advantage and
the disadvantage of the feature space paradigm arise from
the global nature of the derived representation of the input.
On one hand, all the evidence for the presence of a
significant feature is pooled together, providing excellent
tolerance to a noise level which may render local decisions
unreliable. On the other hand, features with lesser support
in the feature space may not be detected in spite of being
salient for the task to be executed. This disadvantage,
however, can be largely avoided by either augmenting the
feature space with additional (spatial) parameters from the
input domain or by robust postprocessing of the input
domain guided by the results of the feature space analysis.
Analysis of the feature space is application independent.
While there are a plethora of published clustering techni-
ques, most of them are not adequate to analyze feature
spaces derived from real data. Methods which rely upon
a priori knowledge of the number of clusters present
(including those which use optimization of a global
criterion to find this number), as well as methods which
implicitly assume the same shape (most often elliptical) for
all the clusters in the space, are not able to handle the
complexity of a real feature space. For a recent survey of
such methods, see [29, Section 8].
In Fig. 1, a typical example is shown. The color image in
Fig. 1a is mapped into the three-dimensional L*u*v* color
space (to be discussed in Section 4). There is a continuous
transition between the clusters arising from the dominant
colors and a decomposition of the space into elliptical tiles
will introduce severe artifacts. Enforcing a Gaussian
mixture model over such data is doomed to fail, e.g., [49],
and even the use of a robust approach with contaminated
Gaussian densities [67] cannot be satisfactory for such
complex cases. Note also that the mixture models require
the number of clusters as a parameter, which raises its own
challenges. For example, the method described in [45]
proposes several different ways to determine this number.
Arbitrarily structured feature spaces can be analyzed
only by nonparametric methods since these methods do not
have embedded assumptions. Numerous nonparametric
clustering methods were described in the literature and
they can be classified into two large classes: hierarchical
clustering and density estimation. Hierarchical clustering
techniques either aggregate or divide the data based on
IEEE TRANSACTIONS ON PATTERN ANALYSIS AND MACHINE INTELLIGENCE, VOL. 24, NO. 5, MAY 2002 603
. D. Comaniciu is with the Imaging and Visualization Department, Siemens
Corporate Research, 755 College Road East, Princeton, NJ 08540.
E-mail: comanici@scr.siemens.com.
. P. Meer is with the Electrical and Computer Engineering Department,
Rutgers University, 94 Brett Road, Piscataway, NJ 08854-8058.
E-mail: meer@caip.rutgers.edu.
Manuscript received 17 Jan. 2001; revised 16 July 2001; accepted 21 Nov.
2001.
Recommended for acceptance by V. Solo.
For information on obtaining reprints of this article, please send e-mail to:
tpami@computer.org, and reference IEEECS Log Number 113483.
0162-8828/02/$17.00 ß 2002 IEEE
- 1
- 2
- 3
- 4
- 5
- 6
前往页