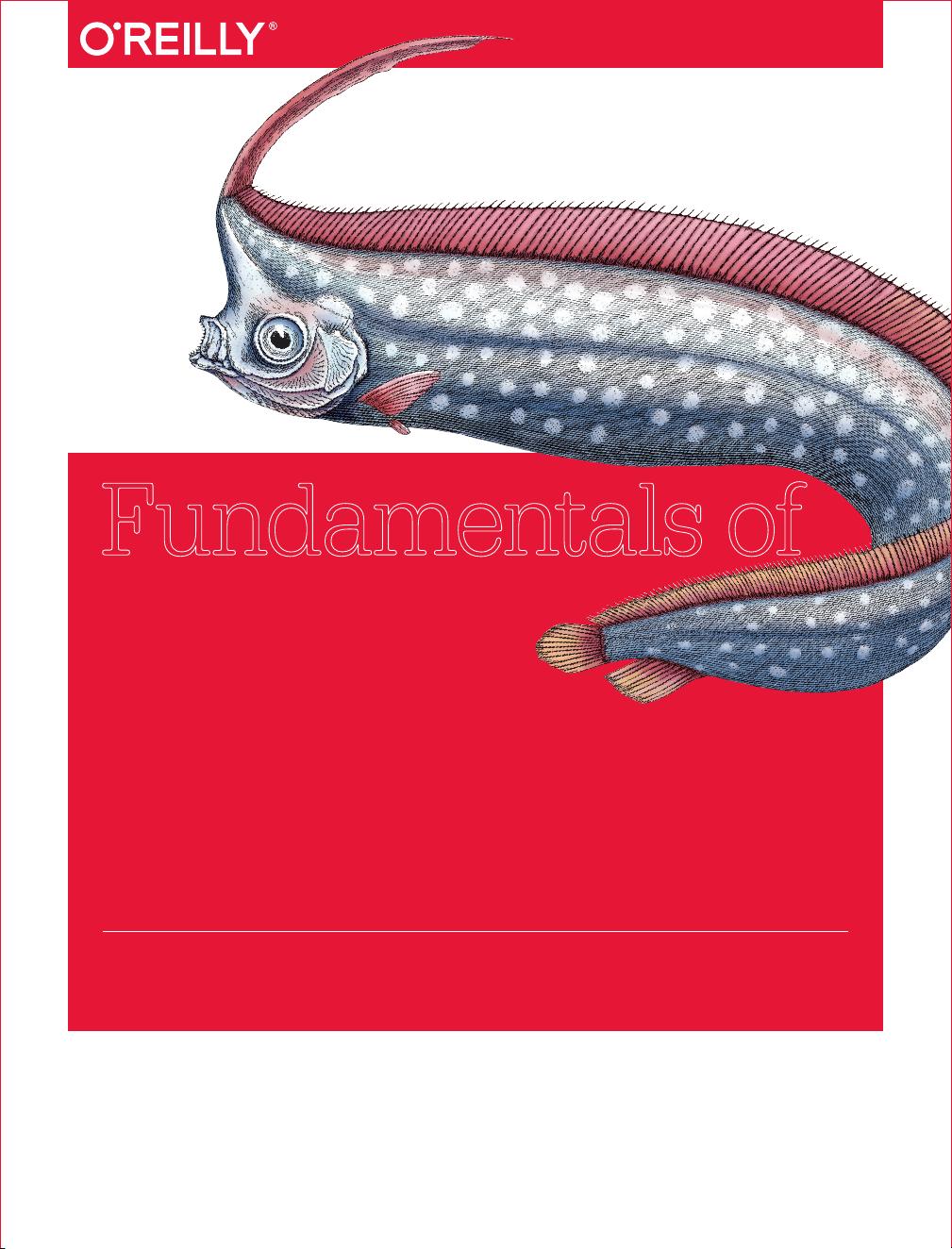
Fundamentals of
Deep
Learning
DESIGNING NEXT-GENERATION
MACHINE INTELLIGENCE ALGORITHMS
Nikhil Buduma
with contributions by Nicholas Locascio
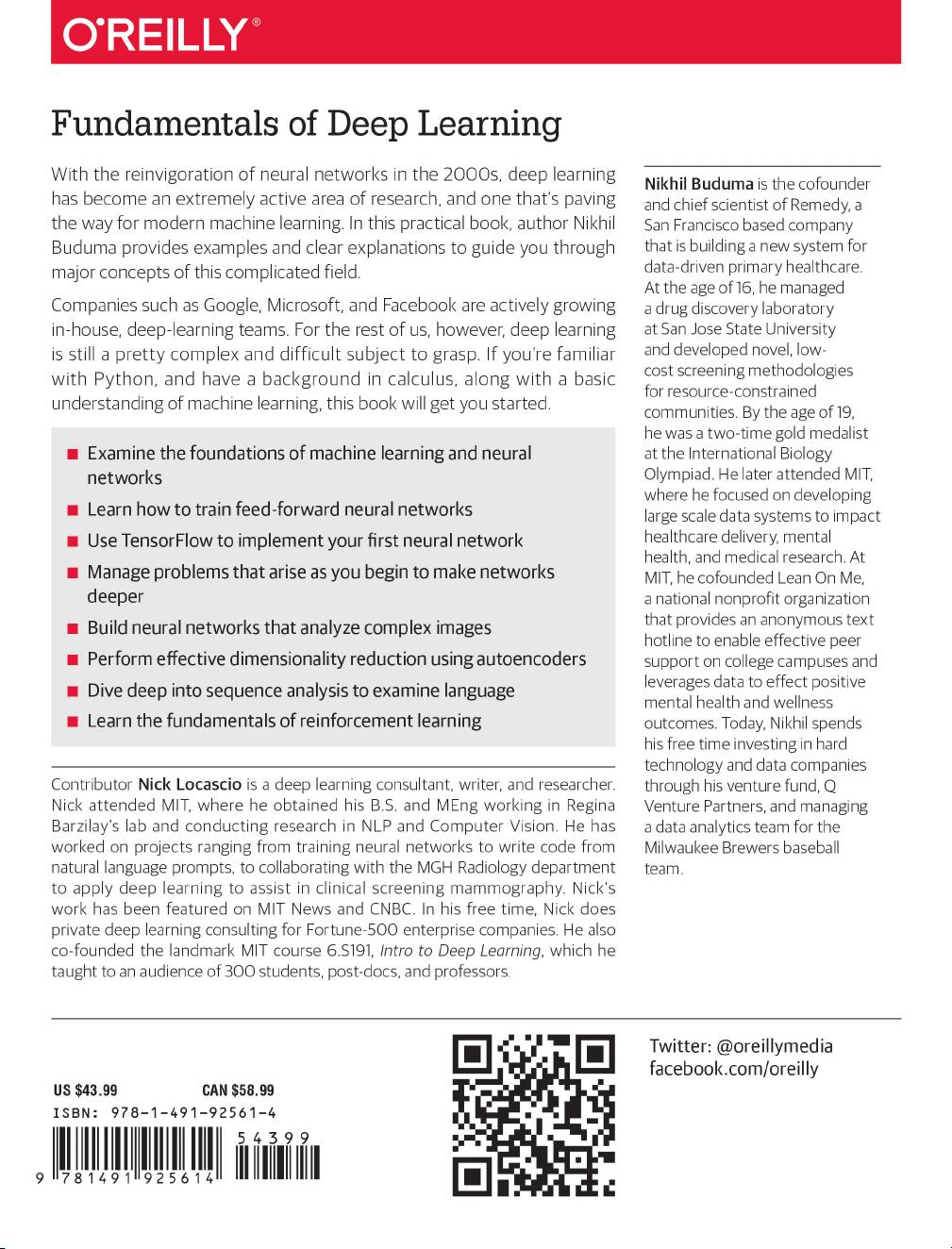
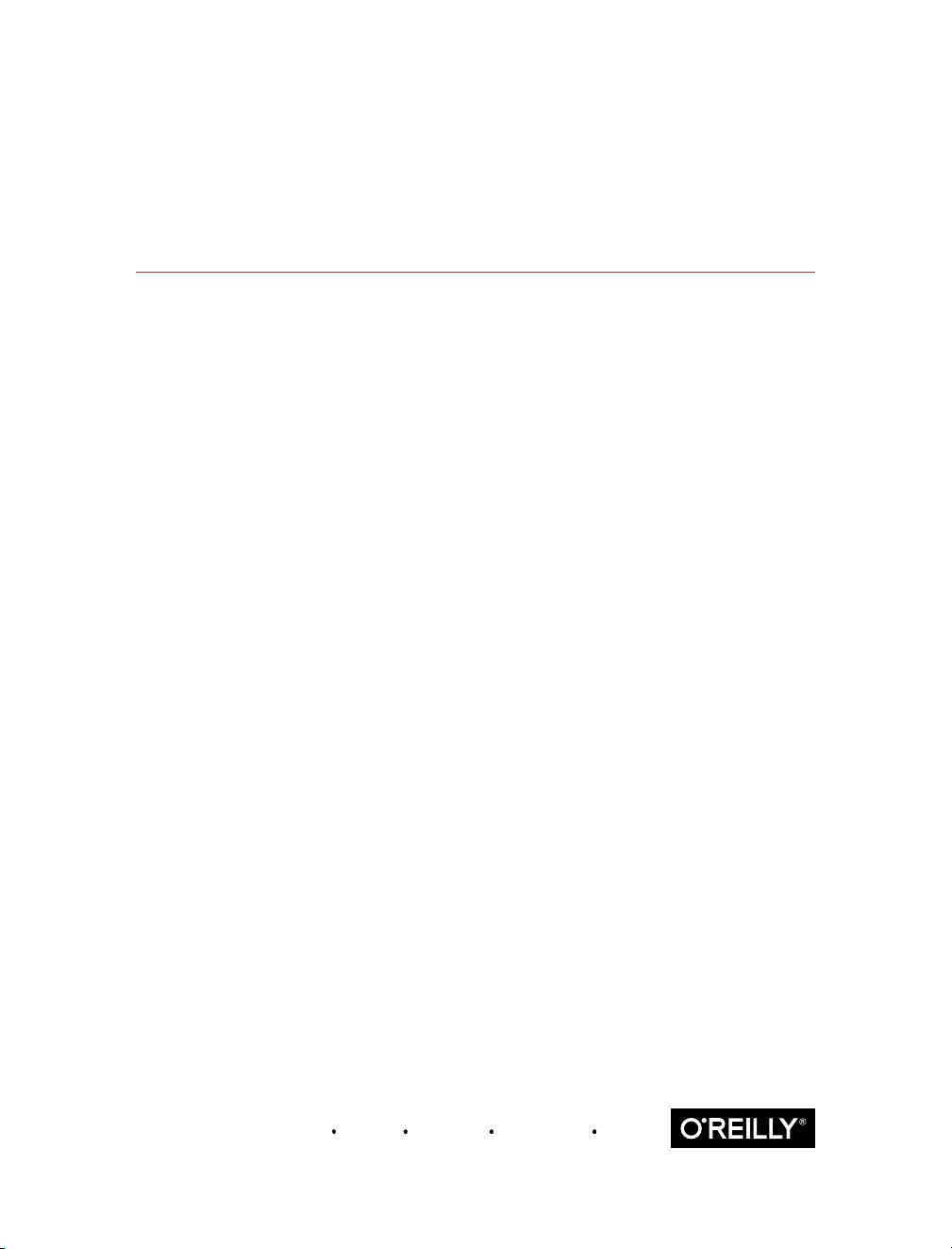
Nikhil Buduma
Fundamentals of Deep Learning
Designing Next-Generation Machine
Intelligence Algorithms
with contributions by Nicholas Locascio
Boston Farnham Sebastopol TokyoBeijing Boston Farnham Sebastopol TokyoBeijing
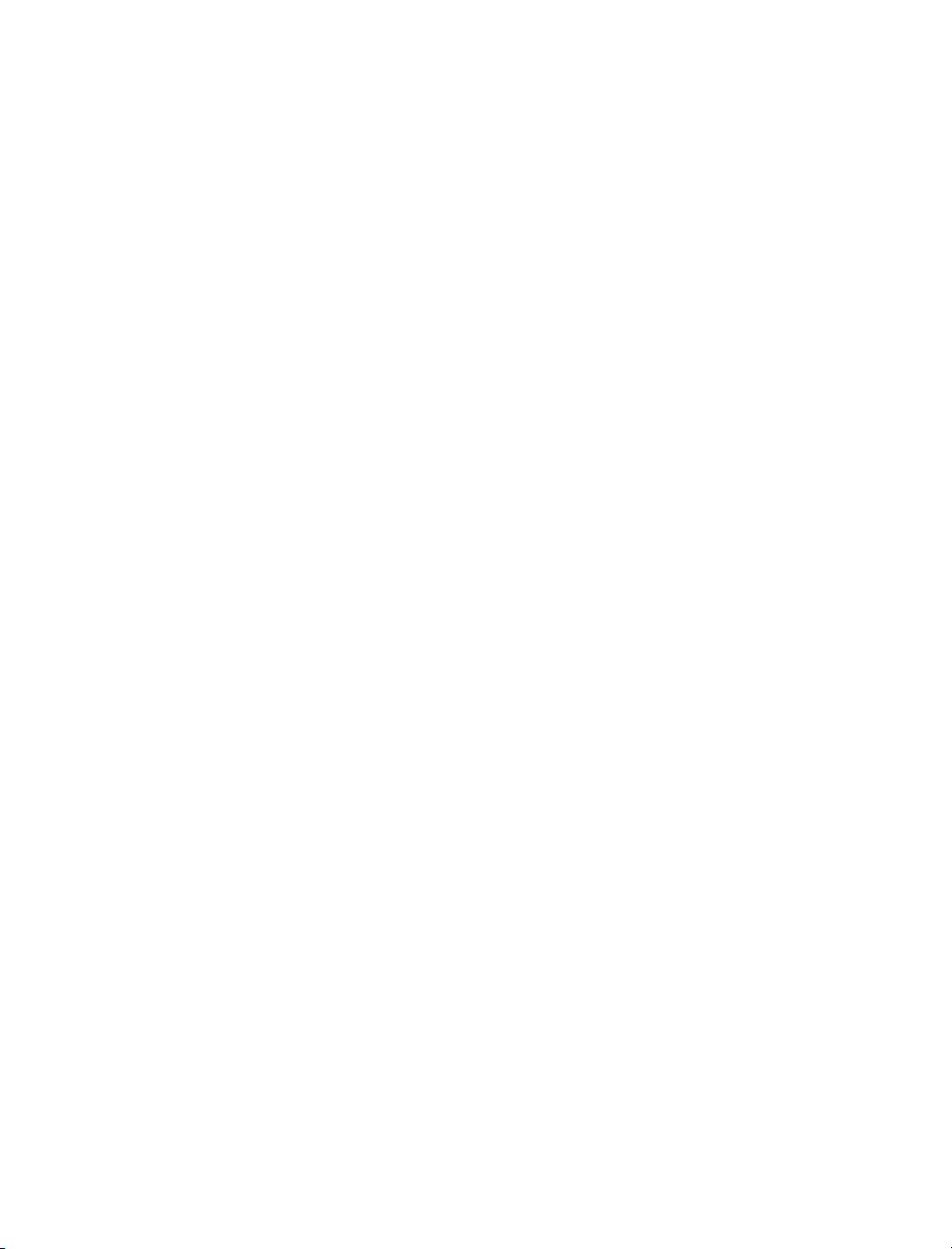
978-1-491-92561-4
[TI]
Fundamentals of Deep Learning
by Nikhil Buduma and Nicholas Lacascio
Copyright © 2017 Nikhil Buduma. All rights reserved.
Printed in the United States of America.
Published by O’Reilly Media, Inc., 1005 Gravenstein Highway North, Sebastopol, CA 95472.
O’Reilly books may be purchased for educational, business, or sales promotional use. Online editions are
also available for most titles (http://oreilly.com/safari). For more information, contact our corporate/insti‐
tutional sales department: 800-998-9938 or corporate@oreilly.com.
Editors: Mike Loukides and Shannon Cutt
Production Editor: Shiny Kalapurakkel
Copyeditor: Sonia Saruba
Proofreader: Amanda Kersey
Indexer: Wendy Catalano
Interior Designer: David Futato
Cover Designer: Karen Montgomery
Illustrator: Rebecca Demarest
June 2017:
First Edition
Revision History for the First Edition
2017-05-25: First Release
The O’Reilly logo is a registered trademark of O’Reilly Media, Inc. Fundamentals of Deep Learning, the
cover image, and related trade dress are trademarks of O’Reilly Media, Inc.
While the publisher and the authors have used good faith efforts to ensure that the information and
instructions contained in this work are accurate, the publisher and the authors disclaim all responsibility
for errors or omissions, including without limitation responsibility for damages resulting from the use of
or reliance on this work. Use of the information and instructions contained in this work is at your own
risk. If any code samples or other technology this work contains or describes is subject to open source
licenses or the intellectual property rights of others, it is your responsibility to ensure that your use
thereof complies with such licenses and/or rights.
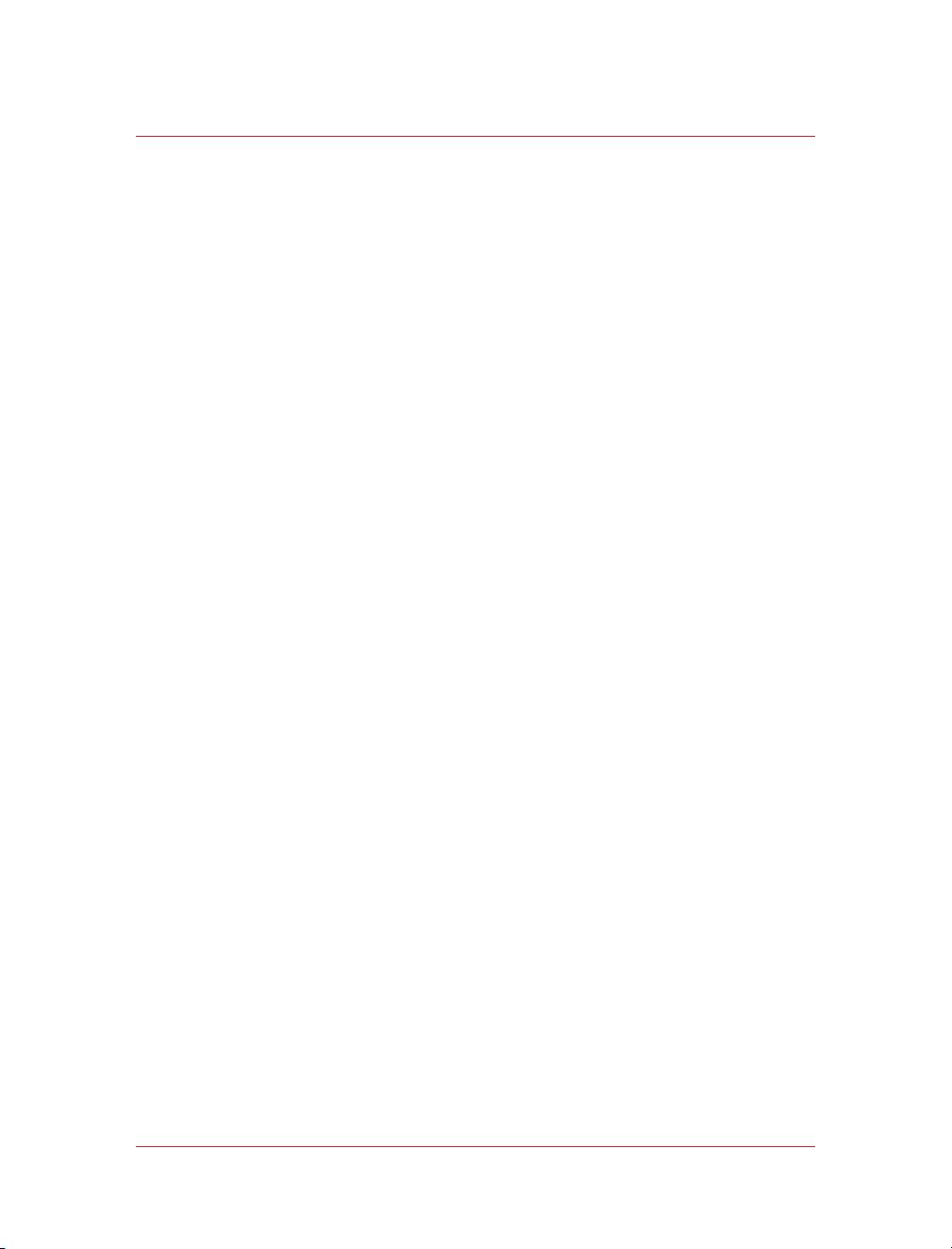
Table of Contents
Preface. . . . . . . . . . . . . . . . . . . . . . . . . . . . . . . . . . . . . . . . . . . . . . . . . . . . . . . . . . . . . . . . . . . . . . . ix
1. The Neural Network. . . . . . . . . . . . . . . . . . . . . . . . . . . . . . . . . . . . . . . . . . . . . . . . . . . . . . . . . 1
Building Intelligent Machines 1
The Limits of Traditional Computer Programs 2
The Mechanics of Machine Learning 3
The Neuron 7
Expressing Linear Perceptrons as Neurons 8
Feed-Forward Neural Networks 9
Linear Neurons and Their Limitations 12
Sigmoid, Tanh, and ReLU Neurons 13
Softmax Output Layers 15
Looking Forward 15
2. Training Feed-Forward Neural Networks. . . . . . . . . . . . . . . . . . . . . . . . . . . . . . . . . . . . . . . 17
The Fast-Food Problem 17
Gradient Descent 19
The Delta Rule and Learning Rates 21
Gradient Descent with Sigmoidal Neurons 22
The Backpropagation Algorithm 23
Stochastic and Minibatch Gradient Descent 25
Test Sets, Validation Sets, and Overfitting 27
Preventing Overfitting in Deep Neural Networks 34
Summary 37
3. Implementing Neural Networks in TensorFlow. . . . . . . . . . . . . . . . . . . . . . . . . . . . . . . . . 39
What Is TensorFlow? 39
How Does TensorFlow Compare to Alternatives? 40
iii