# tensorflow-ml
    
[](https://github.com/siddhantpathakk/tensorflow-ml/actions/workflows/python-publish.yml)
## Introduction
TensorFlow ML is a project that aims to provide an abstract implementation of commonly used machine learning algorithms using TensorFlow, without relying on external libraries like scikit-learn. This project is designed to offer a comprehensive and flexible set of machine learning models that can be used for various tasks, such as classification, regression, clustering, and more.
The TensorFlow ML project is useful for several reasons and can overcome the needs of scikit-learn in various scenarios:
1. **TensorFlow Backend:** By leveraging TensorFlow as its backend, TensorFlow ML can take advantage of its efficient computation and optimization capabilities. TensorFlow is well-known for its ability to work with large-scale datasets and efficiently utilize hardware accelerators like GPUs and TPUs, making it suitable for handling complex machine learning models and tasks.
2. **Flexibility and Customizability:** With TensorFlow ML, users have the flexibility to customize and fine-tune machine learning models according to their specific needs. TensorFlow's symbolic representation and automatic differentiation capabilities enable easy modification of models, loss functions, and optimization algorithms. This level of customization may not always be readily available in pre-implemented models of scikit-learn.
3. **Continuous Development and Updates:** As TensorFlow ML is an open-source project, it can benefit from a large community of contributors and developers. This means that the project is continually evolving, and new algorithms and improvements can be added regularly. This is especially advantageous for staying up-to-date with the latest advancements in machine learning.
4. **Unified Framework:** By using TensorFlow ML, developers and researchers can work within a single framework for both traditional machine learning and deep learning tasks. This avoids the need to switch between different libraries and provides a more cohesive environment for developing and deploying machine learning models.
5. **Community and Support:** TensorFlow has a large and active community of users and contributors. This means that users of TensorFlow ML can benefit from the collective knowledge and support of this community, which can be invaluable for troubleshooting, sharing best practices, and fostering collaborative development.
## Status
This project is currently under development. The following table shows the status of the implemented machine learning models:
| Model | Type | Code | Test |
| ---------------------- | -------------- | ---- | ---- |
| Linear Regression | Regression | Done | Pass |
| Logistic Regression | Classification | Done | Pass |
| NB - GNB/BNB | Classification | Done | Pass |
| Random Forest/GBT/CART | Classification | Done | Pass |
| Lasso Regression | Regression | Done | Pass |
| Polynomial Reg | Regression | Done | Pass |
| Ridge Regression | Regression | Done | Pass |
The following table shows the status of working status on different operating systems:
| Operating System | Status |
| ---------------- | ----------- |
| Linux | Pass |
| MacOS | In Progress |
| Windows | TBC |
## Installing
You can install TensorFlow ML from PyPI using `pip`. Follow these steps:
```bash
pip install tensorflow-ml
```
Alternatively, to use TensorFlow ML from GitHub directly, follow these steps:
1. Clone the GitHub repository: `git clone https://github.com/siddhantpathakk/tensorflow-ml.git`
2. Install the required dependencies by running: `pip install -r requirements.txt`
After the installation is complete, you can import the implemented machine learning models in your Python scripts and start using TensorFlow ML for your machine learning tasks.
## Examples
Here are some examples of how to use TensorFlow ML to create machine learning models:
**REGRESSION:**
* Linear Regression (Regular, Polynomial, Ridge, Lasso)
```python
from tensorflow_ml.regression.linear import LinearRegression
# Try other regression models if you feel like!
# from tensorflow_ml.regression.polynomial import PolynomialRegression
# from tensorflow_ml.regression.ridge import RidgeRegression
# from tensorflow_ml.regression.lasso import LassoRegression
lr = LinearRegression()
# Set the hyper-parameters such as learning-rate, number of epochs etc
lr.set_params(_params)
# Train the model
lr.fit(x, y)
# Get the MSE
lr.evaluate(x, y)
# Get the predictions
lr.predict(x)
# Get the R-squared value
lr.score(x, y)
# Get the coefficients of the trained model
lr.get_coeff()
```
**CLASSIFICATION:**
* Logistic Regression
```python
from tensorflow_ml.classification.logistic_regression import LogisticRegression
logistic_regression = LogisticRegression()
# Set the hyper-parameters such as learning-rate, number of epochs etc
logistic_regression.set_params(params)
# Train the model
logistic_regression.fit(X_train, y_train, random_seed=42, X_val=X_val, y_val=y_val)
# Evaluate the model on the test set
accuracy, cross_entropy_loss = logistic_regression.score(X_test, y_test)
```
* Naive Bayes Classifier (Bernoulli NB, Gaussian NB)
```python
from tensorflow_ml.classification.naive_bayes.bernoulli import BernoulliNaiveBayes
# Set some additional hyper-parameters
bnb = BernoulliNaiveBayes(smoothing=1.0) # Set the desired smoothing parameter
# Train the model
bnb.fit(training_features, training_labels)
# Get the accuracy of the model on test set
accuracy = bnb.evaluate(testing_features, testing_labels)
```
* Random Forests, Gradient Boosted Trees
```python
from tensorflow_ml.classification.decision_tree import DecisionTree
model = DecisionTree(model = "gbt", verbose = True) # Also can use 'rf' for Random Forests, 'cart' for Classification and Regression Tree
# If regression:
model = DecisionTree(model = "gbt", verbose = True, _task = 'regression')
# Get the parameters pre-defined by the model
model.get_params()
# Load the dataset and convert it to TFDS
model.load_dataset(data, label)
# Train the model
model.fit(_metrics = ['mse', 'accuracy'])
# Evaluate the model and view metrics and loss
model.evaluate()
# Make predictions on test/train split of data
model.predict(length=5, split="test")
```
## Participate in Contributing to this Repo
We welcome contributions from the open-source community to enhance the TensorFlow ML library. If you are interested in contributing, follow these steps:
1. Fork the project on GitHub: [https://github.com/siddhantpathakk/tensorflow-ml](https://github.com/your_username/tensorflow-ml)
2. Create a new branch for your feature/bugfix: `git checkout -b feature-name`
3. Implement your changes and improvements.
4. Write tests to ensure the functionality of the added models.
5. Commit your changes and push them to your forked repository.
6. Create a pull request to the main repository, describing your changes in detail.
Your contributions will be reviewed, and once approved, they will be merged into the main project. Let's work together to make TensorFlow ML a powerful and versatile machine learning library!
In summary, TensorFlow ML is a powerful and v

程序员Chino的日记
- 粉丝: 3742
- 资源: 5万+
最新资源
- 2016中国社交媒体影响报告.pdf
- cq08m5kszc3d.pdf
- 2016中国数字营销行动报告.pdf
- 201601 - 凯络十大趋势报告 - 2016.pdf
- App Annie 全球移动应用市场 2015 年回顾.pdf
- Dive_Mobile-OMD.pdf
- IDC:2016全球数据中心预测.pdf
- go1.23.4.linux-amd64
- 山东春季高考C语言练习题目
- 基于单片机八人数字抢答器仿真、程序、设计报告 (1)抢答器同时供 8 名选手比赛,分别用8个按钮 s1-s8表示 (5)选手在设定的时间内进行抢答,抢答有效,定时器停止工作,蜂鸣器报警,数码管显示选手
- go1.23.4.src
- 水泵控制程序,跟随压力加减机,定时轮,故障自动投入,水泵相互备用 1.模式为0,先停泵,然后启动水泵 2.模式为1, 先启泵,然后在停泵 3.故障自动切水泵 4.当切泵时,启动运行时间最短的泵 5.当
- MATLAB界面版本- BP神经网络的火焰识别.zip
- 基于JAVA实现的五子棋人机对弈游戏
- MATLAB界面版本- GUI的水果识别.zip
- 5560m5nd6n7z.pdf
资源上传下载、课程学习等过程中有任何疑问或建议,欢迎提出宝贵意见哦~我们会及时处理!
点击此处反馈


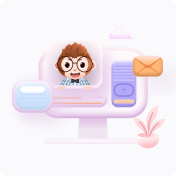