# tensorflow-qnd
[](https://badge.fury.io/py/tensorflow-qnd)
[](setup.py)
[](https://app.wercker.com/project/byKey/28ed86fae4933770c92881a466e81929)
[](https://unlicense.org)
Quick and Dirty TensorFlow command framework
tensorflow-qnd is a TensorFlow framework to create commands to train and
evaluate models and make inference with them.
The framework is built on top of
[TF Learn](https://github.com/tensorflow/tensorflow/tree/master/tensorflow/contrib/learn/python/learn).
Especially if you are working on research projects using TensorFlow, you can
remove most of boilerplate code with this framework.
All you need to do is to define a model constructor `model_fn` and input
producer(s) `input_fn` to feed a dataset to the model.
## Features
- Creation of commands
- To train and evaluate models
- To infer labels or regression values with trained models
- Configuration of command line arguments to set hyperparameters of models etc.
- [Distributed TensorFlow](https://www.tensorflow.org/how_tos/distributed/)
- Just set an optional argument `distributed ` of `def_train_and_evaluate()`
as `True` (i.e. `def_train_and_evaluate(distributed=True)`) to enable it.
- Supports only data parallel training
- Only for training and evaluation but not for inference
## Installation
Python 3.5+ and TensorFlow 0.12+ are required.
```
pip3 install --user --upgrade tensorflow-qnd
```
## Usage
1. Add command line arguments with `add_flag` and `add_required_flag` functions.
2. Define a `train_and_evaluate` or `infer` function with
`def_train_and_evaluate` or `def_infer` function
3. Pass `model_fn` (model constructor) and `input_fn` (input producer) functions
to that defined function.
4. Run the script with appropriate command line arguments.
For more information, see [documentation](https://raviqqe.github.io/tensorflow-qnd/qnd).
## Examples
`train.py` (command script):
```python
import logging
import os
import qnd
import mnist
train_and_evaluate = qnd.def_train_and_evaluate(
distributed=("distributed" in os.environ))
model = mnist.def_model()
def main():
logging.getLogger().setLevel(logging.INFO)
train_and_evaluate(model, mnist.read_file)
if __name__ == "__main__":
main()
```
`mnist.py` (module):
```python
import qnd
import tensorflow as tf
def read_file(filename_queue):
_, serialized = tf.TFRecordReader().read(filename_queue)
scalar_feature = lambda dtype: tf.FixedLenFeature([], dtype)
features = tf.parse_single_example(serialized, {
"image_raw": scalar_feature(tf.string),
"label": scalar_feature(tf.int64),
})
image = tf.decode_raw(features["image_raw"], tf.uint8)
image.set_shape([28**2])
return tf.to_float(image) / 255 - 0.5, features["label"]
def minimize(loss):
return tf.train.AdamOptimizer().minimize(
loss,
tf.contrib.framework.get_global_step())
def def_model():
qnd.add_flag("hidden_layer_size", type=int, default=64,
help="Hidden layer size")
def model(image, number=None, mode=None):
h = tf.contrib.layers.fully_connected(image,
qnd.FLAGS.hidden_layer_size)
h = tf.contrib.layers.fully_connected(h, 10, activation_fn=None)
predictions = tf.argmax(h, axis=1)
if mode == tf.contrib.learn.ModeKeys.INFER:
return predictions
loss = tf.reduce_mean(
tf.nn.sparse_softmax_cross_entropy_with_logits(h, number))
return predictions, loss, minimize(loss), {
"accuracy": tf.contrib.metrics.streaming_accuracy(predictions,
number)[1],
}
return model
```
With the code above, you can create a command with the following interface.
```
usage: train.py [-h] [--output_dir OUTPUT_DIR] [--train_steps TRAIN_STEPS]
[--eval_steps EVAL_STEPS]
[--min_eval_frequency MIN_EVAL_FREQUENCY]
[--num_cores NUM_CORES] [--log_device_placement]
[--save_summary_steps SAVE_SUMMARY_STEPS]
[--save_checkpoints_steps SAVE_CHECKPOINTS_STEPS]
[--keep_checkpoint_max KEEP_CHECKPOINT_MAX]
[--batch_size BATCH_SIZE]
[--batch_queue_capacity BATCH_QUEUE_CAPACITY]
[--num_batch_threads NUM_BATCH_THREADS] --train_file
TRAIN_FILE [--filename_queue_capacity FILENAME_QUEUE_CAPACITY]
--eval_file EVAL_FILE [--hidden_layer_size HIDDEN_LAYER_SIZE]
optional arguments:
-h, --help show this help message and exit
--output_dir OUTPUT_DIR
Directory where checkpoint and event files are stored
(default: output)
--train_steps TRAIN_STEPS
Maximum number of train steps (default: None)
--eval_steps EVAL_STEPS
Maximum number of eval steps (default: None)
--min_eval_frequency MIN_EVAL_FREQUENCY
Minimum evaluation frequency in number of train steps
(default: 1)
--num_cores NUM_CORES
Number of CPU cores used. 0 means use of a default
value. (default: 0)
--log_device_placement
If specified, log device placement information
(default: False)
--save_summary_steps SAVE_SUMMARY_STEPS
Number of steps every time of which summary is saved
(default: 100)
--save_checkpoints_steps SAVE_CHECKPOINTS_STEPS
Number of steps every time of which a model is saved
(default: None)
--keep_checkpoint_max KEEP_CHECKPOINT_MAX
Max number of kept checkpoint files (default: 86058)
--batch_size BATCH_SIZE
Mini-batch size (default: 64)
--batch_queue_capacity BATCH_QUEUE_CAPACITY
Batch queue capacity (default: 1024)
--num_batch_threads NUM_BATCH_THREADS
Number of threads used to create batches (default: 16)
--train_file TRAIN_FILE
File path of train data file(s). A glob is available.
(e.g. train/*.tfrecords) (default: None)
--filename_queue_capacity FILENAME_QUEUE_CAPACITY
Capacity of filename queues of train, eval and infer
data (default: 32)
--eval_file EVAL_FILE
File path of eval data file(s). A glob is available.
(e.g. eval/*.tfrecords) (default: None)
--hidden_layer_size HIDDEN_LAYER_SIZE
Hidden layer size (default: 64)
```
Explore [examples](examples) directory for more information and see how to run
them.
## Caveats
### Necessary update of a global step variable
As done in [examples](examples), you must get a global step variable with
`tf.contrib.framework.get_global_step()` and update (increment) it in each
training step.
### Use streaming metrics for `eval_metric_ops`
When non-streaming ones such as `tf.contrib.metrics.accuracy` are used in a
return value `eval_metric_ops` of your `model_fn` or as arguments of
`ModelFnOps`, their values will be ones of the last batch in every evaluation
step.
## Contributing
Please send issues about any bugs, feature requests or questions, or pull
requests.
## License
[The Unlicense](https://unlicense.org)
没有合适的资源?快使用搜索试试~ 我知道了~
tensorflow-qnd-0.0.16.tar.gz
0 下载量 134 浏览量
2024-03-21
12:48:51
上传
评论
收藏 13KB GZ 举报
温馨提示
Python库是一组预先编写的代码模块,旨在帮助开发者实现特定的编程任务,无需从零开始编写代码。这些库可以包括各种功能,如数学运算、文件操作、数据分析和网络编程等。Python社区提供了大量的第三方库,如NumPy、Pandas和Requests,极大地丰富了Python的应用领域,从数据科学到Web开发。Python库的丰富性是Python成为最受欢迎的编程语言之一的关键原因之一。这些库不仅为初学者提供了快速入门的途径,而且为经验丰富的开发者提供了强大的工具,以高效率、高质量地完成复杂任务。例如,Matplotlib和Seaborn库在数据可视化领域内非常受欢迎,它们提供了广泛的工具和技术,可以创建高度定制化的图表和图形,帮助数据科学家和分析师在数据探索和结果展示中更有效地传达信息。
资源推荐
资源详情
资源评论
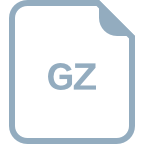
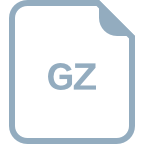
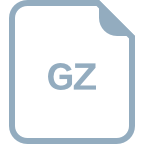
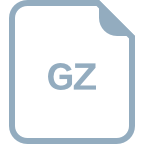
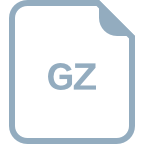
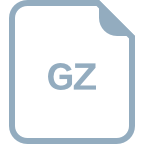
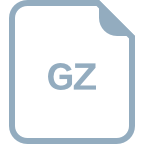
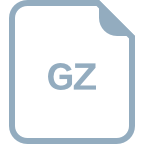
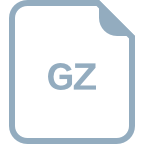
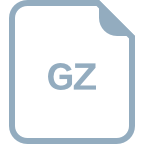
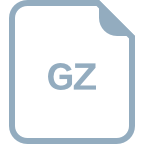
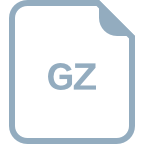
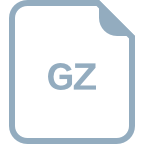
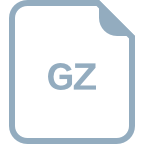
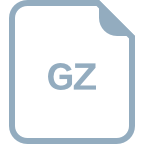
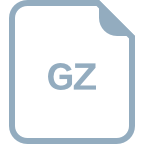
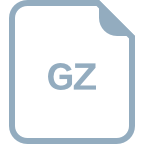
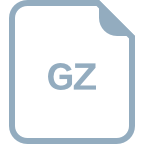
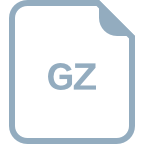
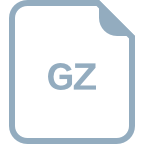
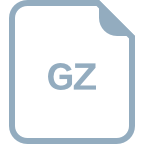
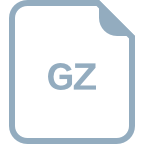
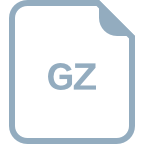
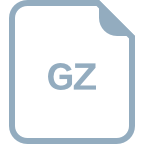
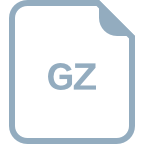
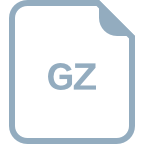
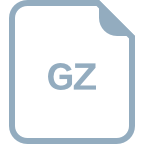
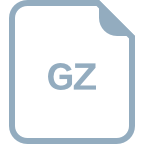
收起资源包目录


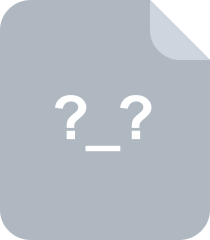
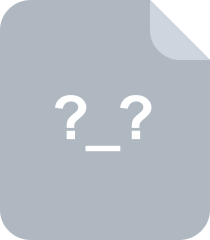
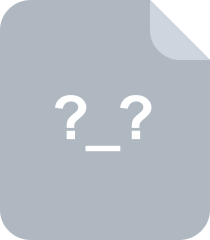
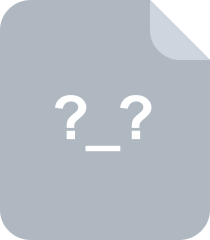
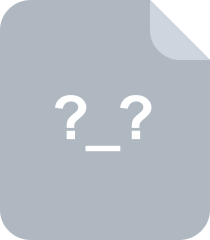

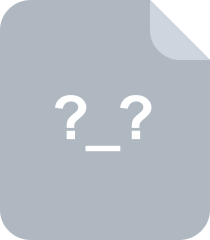
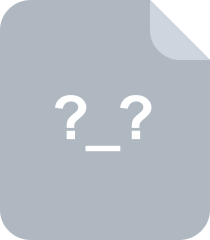
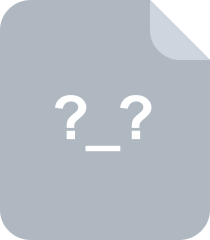
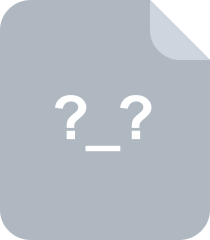
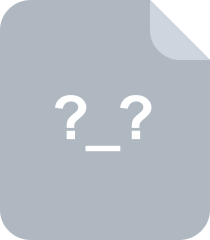
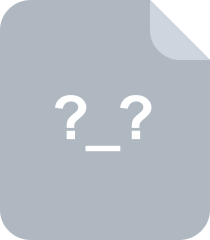
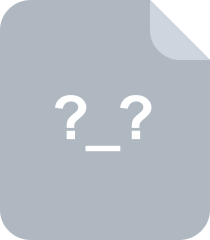
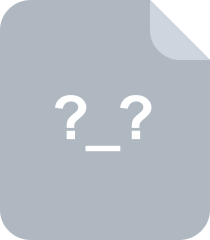
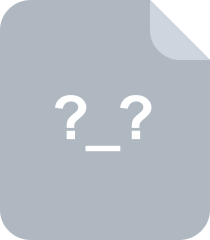
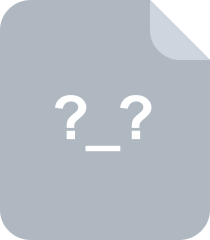
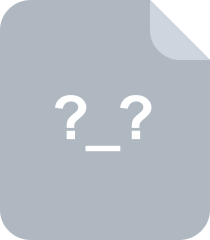
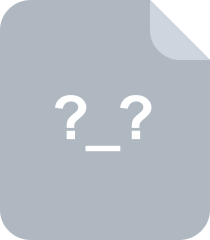
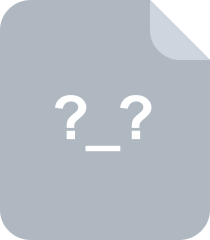
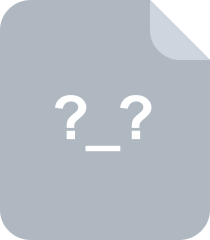
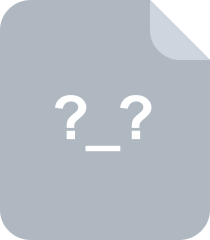
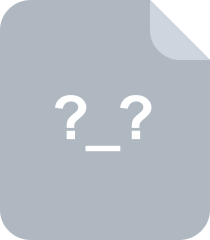
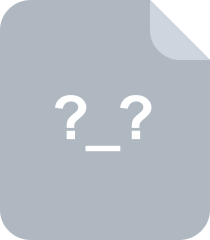
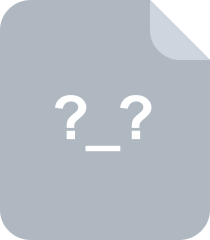
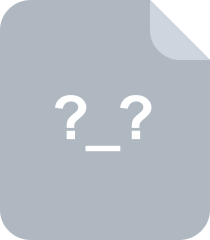
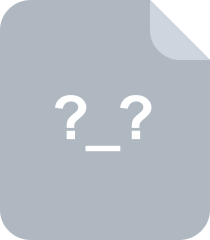

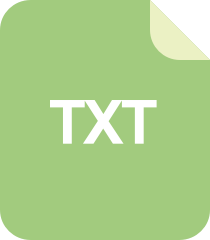
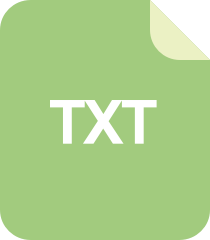
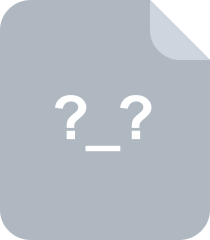
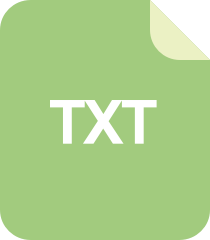
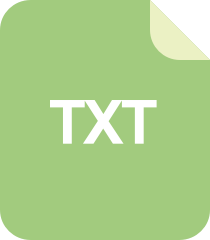
共 30 条
- 1
资源评论
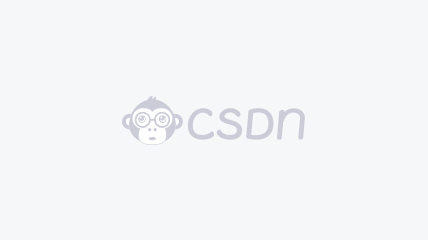

程序员Chino的日记
- 粉丝: 3712
- 资源: 5万+
上传资源 快速赚钱
我的内容管理 展开
我的资源 快来上传第一个资源
我的收益
登录查看自己的收益我的积分 登录查看自己的积分
我的C币 登录后查看C币余额
我的收藏
我的下载
下载帮助

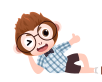
最新资源
资源上传下载、课程学习等过程中有任何疑问或建议,欢迎提出宝贵意见哦~我们会及时处理!
点击此处反馈


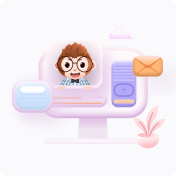
安全验证
文档复制为VIP权益,开通VIP直接复制
