TensorFlow Plot (tfplot)
========================
[][pypi_tfplot]
[][documentation]
[](https://travis-ci.org/wookayin/tensorflow-plot)
A [TensorFlow][tensorflow] utility for providing matplotlib-based **plot** operations
â [TensorBoard][tensorboard] â¤ï¸ [Matplotlib][matplotlib].
<p align="center">
<i> ð§ Under Construction â API might change!</i>
</p>
It allows us to draw **_any_** [matplotlib][matplotlib] plots or figures into images,
as a part of TensorFlow computation graph.
Especially, we can easily any plot and see the result image
as an image summary in [TensorBoard][tensorboard].
<p align="center">
<img src="./assets/tensorboard-plot-summary.png" width="70%" />
</p>
Quick Overview
--------------
There are two main ways of using `tfplot`: (i) Use as TF op, and (ii) Manually add summary protos.
### Usage: Decorator
You can directly declare a Tensor factory by using [`tfplot.autowrap`][tfplot-autowrap] as a decorator.
In the body of the wrapped function you can add any logic for drawing plots. Example:
```python
@tfplot.autowrap(figsize=(2, 2))
def plot_scatter(x: np.ndarray, y: np.ndarray, *, ax, color='red'):
ax.scatter(x, y, color=color)
x = tf.constant([1, 2, 3], dtype=tf.float32) # tf.Tensor
y = tf.constant([1, 4, 9], dtype=tf.float32) # tf.Tensor
plot_op = plot_scatter(x, y) # tf.Tensor shape=(?, ?, 4) dtype=uint8
```
### Usage: Wrap as TF ops
We can [wrap][tfplot-autowrap] **any** pure python function for plotting as a Tensorflow op, such as:
- (i) A python function that creates and return a matplotlib `Figure` (see below)
- (ii) A python function that has `fig` or `ax` keyword parameters (will be auto-injected);
e.g. [`seaborn.heatmap`](http://seaborn.pydata.org/generated/seaborn.heatmap.html)
- (iii) A method instance of [matplotlib `Axes`](https://matplotlib.org/api/axes_api.html);
e.g. [`Axes.scatter`](https://matplotlib.org/api/_as_gen/matplotlib.axes.Axes.scatter.html#matplotlib.axes.Axes.scatter)
Example of (i): You can define a python function that takes `numpy.ndarray` values as input (as an argument of Tensor input),
and draw a plot as a return value of `matplotlib.figure.Figure`.
The resulting TensorFlow plot op will be a RGBA image tensor of shape `[height, width, 4]` containing the resulting plot.
```python
def figure_heatmap(heatmap, cmap='jet'):
# draw a heatmap with a colorbar
fig, ax = tfplot.subplots(figsize=(4, 3)) # DON'T USE plt.subplots() !!!!
im = ax.imshow(heatmap, cmap=cmap)
fig.colorbar(im)
return fig
heatmap_tensor = ... # tf.Tensor shape=(16, 16) dtype=float32
# (a) wrap function as a Tensor factory
plot_op = tfplot.autowrap(figure_heatmap)(heatmap_tensor) # tf.Tensor shape=(?, ?, 4) dtype=uint8
# (b) direct invocation similar to tf.py_func
plot_op = tfplot.plot(figure_heatmap, [heatmap_tensor], cmap='jet')
# (c) or just directly add an image summary with the plot
tfplot.summary.plot("heatmap_summary", figure_heatmap, [heatmap_tensor])
```
Example of (ii):
```python tfplot
import tfplot
import seaborn.apionly as sns
tf_heatmap = tfplot.autowrap(sns.heatmap, figsize=(4, 4), batch=True) # function: Tensor -> Tensor
plot_op = tf_heatmap(attention_maps) # tf.Tensor shape=(?, 400, 400, 4) dtype=uint8
tf.summary.image("attention_maps", plot_op)
```
Please take a look at the [the showcase][examples-showcase] or [examples directory][examples-dir] for more examples and use cases.
[The full documentation][documentation] including API docs can be found at [readthedocs][documentation].
### Usage: Manually add summary protos
```python
import tensorboard as tb
fig, ax = ...
# Get RGB image manually or by executing plot ops.
embedding_plot = sess.run(plot_op) # ndarray [H, W, 3] uint8
embedding_plot = tfplot.figure_to_array(fig) # ndarray [H, W, 3] uint8
summary_pb = tb.summary.image_pb('plot_embedding', [embedding_plot])
summary_writer.write_add_summary(summary_pb, global_step=global_step)
```
Installation
------------
```
pip install tensorflow-plot
```
To grab the latest development version:
```
pip install git+https://github.com/wookayin/tensorflow-plot.git@master
```
Note
----
### Some comments on Speed
* Matplotlib operations can be **very** slow as Matplotlib runs in python rather than native code,
so please watch out for runtime speed.
There is still a room for improvement, which will be addressed in the near future.
* Moreover, it might be also a good idea to draw plots from the main code (rather than having a TF op) and add them as image summaries.
Please use this library at your best discernment.
### Thread-safety issue
Please use **object-oriented** matplotlib APIs (e.g. `Figure`, `AxesSubplot`)
instead of [pyplot] APIs (i.e. `matplotlib.pyplot` or `plt.XXX()`)
when creating and drawing plots.
This is because [pyplot] APIs are not *thread-safe*,
while the TensorFlow plot operations are usually executed in multi-threaded manners.
For example, avoid any use of `pyplot` (or `plt`):
```python
# DON'T DO LIKE THIS !!!
def figure_heatmap(heatmap):
fig = plt.figure() # <--- NO!
plt.imshow(heatmap)
return fig
```
and do it like:
```python
def figure_heatmap(heatmap):
fig = matplotlib.figure.Figure() # or just `fig = tfplot.Figure()`
ax = fig.add_subplot(1, 1, 1) # ax: AxesSubplot
# or, just `fig, ax = tfplot.subplots()`
ax.imshow(heatmap)
return fig # fig: Figure
```
For example, `tfplot.subplots()` is a good replacement for `plt.subplots()`
to use inside plot functions.
Alternatively, you can just take advantage of automatic injection of `fig` and/or `ax`.
[pypi_tfplot]: https://pypi.python.org/pypi/tfplot
[matplotlib]: http://matplotlib.org/
[tensorflow]: https://www.tensorflow.org/
[tensorboard]: https://www.tensorflow.org/get_started/summaries_and_tensorboard
[pyplot]: http://matplotlib.org/api/pyplot_api.html
[examples-dir]: https://github.com/wookayin/tensorflow-plot/blob/master/examples/
[examples-showcase]: https://github.com/wookayin/tensorflow-plot/blob/master/examples/showcases.ipynb
[documentation]: http://tensorflow-plot.readthedocs.io/en/latest/
[tfplot-autowrap]: https://tensorflow-plot.readthedocs.io/en/latest/api/tfplot.html#tfplot.autowrap
### TensorFlow compatibility
Currently, `tfplot` is compatible with TensorFlow 1.x series.
Support for eager execution and TF 2.0 will be coming soon!
License
-------
[MIT License](LICENSE) © Jongwook Choi

程序员Chino的日记
- 粉丝: 3822
- 资源: 5万+
最新资源
- 基于Matlab的通信边缘计算仿真与雷达跟踪算法研究:卡尔曼滤波系列及粒子群路径规划算法改进,基于Matlab的通信边缘计算仿真与雷达跟踪算法研究:卡尔曼滤波及算法改进,Matlab通信边缘计算通信仿
- C语言实现selcet服务端
- 狼群算法:实现柔性车间调度问题的MATLAB解决方案及源码学习资源,狼群算法在柔性车间调度中的优化应用:Matlab源码解析与实践指南,狼群算法求解柔性车间调度matlab版 有源码提供学习 可直接
- 【科研绘图系列】R语言绘制SCI论文图合集
- 基于改进人工鱼群法的机器人路径规划算法MATLAB复现,自适应视野与步长优化及启发选择机制应用,自适应视野与步长改进人工鱼群算法的机器人路径规划算法复现,MATLAB实现无人机、无人车、无人船应用,l
- 基于组态王的水蓄冷中央空调变频器控制系统设计组态方案,基于组态王的水蓄冷中央空调变频器控制系统设计组态方案,组态王设计变频器的水蓄冷中央空调控制系统设计组态设计 ,组态王设计; 变频器; 水蓄冷中央
- 永磁同步电机直接公式法计算:弱磁控制与最大转矩电流比(MTPA)结合应用 详细涵盖内环到外环电流环仿真步骤,参数变化影响解析,结合MATLAB实现的注意事项及独特补充内容 ,永磁同步电机公式法计算:M
- 三相逆变器下垂控制:负载突变分析与控制阶跃响应曲线图集(附结构控制图),三相逆变器下垂控制详解:负载突变分析与控制阶跃响应曲线图集(附结构图),三相逆变器之下垂控制?负载突变分析 图一控制阶跃信号为0
- python中国政府采购网爬虫
- 简历系统(基于springboot,mysql,java).zip
- the last-个人-计划备选
- Optislang电机:多目标协同优化NVH优化模型的实践与应用,基于Optislang技术的多目标优化电机NVH性能优化模型,Optislang电机 多目标优化电机NVH优化模型 ,Optislan
- 正激式小功率开关电路与开环闭环电压控制仿真对比研究:基于Matlab仿真平台的具体参数解析与效果评估,深入探究:正激式小功率开关电路Matlab仿真及其开环与闭环(电压闭环)对比仿真过程与具体参数详解
- 基于灰狼优化算法的支持向量机(GWO-SVM)教程:适合新手学习的完整代码实现,包含注释和可直接运行的数据接入,灰狼算法优化的支持向量机(GWO-SVM):适合新手学习,代码齐全,注释丰富,轻松运行
- 基于MATLAB Simulink的光伏逆变器低电压穿越仿真模型,采用boost加NPC拓扑结构与SVPWM控制,实现正负序分离及pll控制,基于MATLAB Simulink的光伏逆变器低电压穿越仿
- 交错并联Boost PFC仿真电路模型:双闭环控制方式下的输出电压与电感电流优化控制,优良波形及Simulink仿真实现,交错并联Boost PFC仿真电路模型:双闭环控制方式下的电压外环与电感电流内
资源上传下载、课程学习等过程中有任何疑问或建议,欢迎提出宝贵意见哦~我们会及时处理!
点击此处反馈


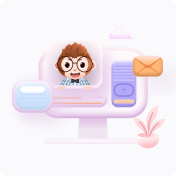