# -*- coding: utf-8 -*-
import time
from functools import wraps
import pandas as pd
from pandas.core.base import PandasObject
from pandas_ta.candles import *
from pandas_ta.momentum import *
from pandas_ta.overlap import *
from pandas_ta.performance import *
from pandas_ta.statistics import *
from pandas_ta.trend import *
from pandas_ta.volatility import *
from pandas_ta.volume import *
from pandas_ta.utils import *
version = ".".join(("0", "1", "66b"))
def finalize(method):
@wraps(method)
def _wrapper(*class_methods, **method_kwargs):
cm = class_methods[0]
result = method(cm, **method_kwargs)
cm._add_prefix_suffix(result, **method_kwargs)
cm._append(result, **method_kwargs)
return result
return _wrapper
class BasePandasObject(PandasObject):
"""Simple PandasObject Extension
Ensures the DataFrame is not empty and has columns. It would be a
sad Panda otherwise.
Args:
df (pd.DataFrame): Extends Pandas DataFrame
"""
def __init__(self, df, **kwargs):
if df.empty: return
if len(df.columns) > 0:
self._df = df
else:
raise AttributeError(f" [X] No columns!")
def __call__(self, kind, *args, **kwargs):
raise NotImplementedError()
@pd.api.extensions.register_dataframe_accessor('ta')
class AnalysisIndicators(BasePandasObject):
"""AnalysisIndicators is a class that extends the Pandas DataFrame via
Pandas @pd.api.extensions.register_dataframe_accessor('name') decorator.
This Pandas Extension is named 'ta' for Technical Analysis that allows us
to apply technical indicators by extension. Even though 'ta' is a
Pandas DataFrame Extension, you can still call the Indicators
individually. Use help() if needed.
By default the 'ta' extension uses lower case column names: open, high,
low, close, and volume. You can override the defaults by providing the
it's replacement name when calling the indicator. For example, to call the
indicator hl2().
With 'default' columns: open, high, low, close, and volume.
>>> df.ta.hl2()
>>> df.ta(kind='hl2')
With DataFrame columns: Open, High, Low, Close, and Volume.
>>> df.ta.hl2(high='High', low='Low')
>>> df.ta(kind='hl2', high='High', low='Low')
If you do not want to use a DataFrame Extension, just call it normally.
>>> sma10 = ta.sma(df['Close']) # Default length=10
>>> sma50 = ta.sma(df['Close'], length=50)
>>> ichimoku, span = ta.ichimoku(df['High'], df['Low'], df['Close'])
Args:
kind (str, optional): Default: None. Kind is the 'name' of the indicator.
It converts kind to lowercase before calling.
timed (bool, optional): Default: False. Curious about the execution
speed?
kwargs: Extension specific modifiers.
append (bool, optional): Default: False. When True, it appends the
resultant column(s) to the DataFrame.
Returns:
Most Indicators will return a Pandas Series. Others like MACD, BBANDS,
KC, et al will return a Pandas DataFrame. Ichimoku on the other hand
will return two DataFrames, the Ichimoku DataFrame for the known period
and a Span DataFrame for the future of the Span values.
Let's get started!
1. Loading the 'ta' module:
>>> import pandas as pd
>>> import ta as ta
2. Load some data:
>>> df = pd.read_csv('AAPL.csv', index_col='date', parse_dates=True)
3. Help!
3a. General Help:
>>> help(df.ta)
>>> df.ta()
3b. Indicator Help:
>>> help(ta.apo)
3c. Indicator Extension Help:
>>> help(df.ta.apo)
4. Ways of calling an indicator.
4a. Calling just the MACD indicator without 'ta' DataFrame extension.
>>> ta.apo(df['close'])
4b. Calling just the MACD indicator with 'ta' DataFrame extension.
>>> df.ta.apo()
4c. Calling using kind.
>>> df.ta(kind='apo')
5. Working with kwargs
5a. Append the result to the working df.
>>> df.ta.apo(append=True)
5b. Timing an indicator.
>>> apo = df.ta(kind='apo', timed=True)
>>> print(apo.timed)
"""
_adjusted = None
def __call__(self, kind=None, alias=None, timed=False, **kwargs):
try:
if isinstance(kind, str):
kind = kind.lower()
fn = getattr(self, kind)
if timed:
stime = time.time()
# Run the indicator
indicator = fn(**kwargs)
if timed:
time_diff = time.time() - stime
ms = time_diff * 1000
indicator.timed = f"{ms:2.3f} ms ({time_diff:2.3f} s)"
# print(f"execution time: {indicator.timed}")
self._df.timed = indicator.timed
# Add an alias if passed
if alias:
indicator.alias = f"{alias}"
return indicator
else:
self.help()
except:
self.help()
@property
def adjusted(self) -> str:
"""property: df.ta.adjusted"""
return self._adjusted
@adjusted.setter
def adjusted(self, value:str) -> None:
"""property: df.ta.adjusted = 'adj_close'"""
if value is not None and isinstance(value, str):
self._adjusted = value
else:
self._adjusted = None
@property
def datetime_ordered(self) -> bool:
"""Returns True if the index is a datetime and ordered."""
return is_datetime_ordered(self._df)
@property
def reverse(self) -> pd.DataFrame:
"""Reverses the DataFrame. Simply: df.iloc[::-1]"""
return self._df.iloc[::-1]
@property
def version(self) -> str:
"""Returns the version."""
return version
def _append(self, result=None, **kwargs):
"""Appends a Pandas Series or DataFrame columns to self._df."""
if 'append' in kwargs and kwargs['append']:
df = self._df
if df is None or result is None: return
else:
if isinstance(result, pd.DataFrame):
for i, column in enumerate(result.columns):
df[column] = result.iloc[:,i]
else:
df[result.name] = result
def _add_prefix_suffix(self, result=None, **kwargs):
"""Add prefix and/or suffix to the result columns"""
if result is None: return
else:
prefix = suffix = ""
# delimiter = kwargs.pop("delimiter", "_")
if "prefix" in kwargs:
prefix = f"{kwargs['prefix']}_"
if "suffix" in kwargs:
suffix = f"_{kwargs['suffix']}"
if isinstance(result, pd.Series):
result.name = prefix + result.name + suffix
else:
result.columns = [prefix + column + suffix for column in result.columns]
def _get_column(self, series, default):
"""Attempts to get the correct series or 'column' and return it."""
df = self._df
if df is None: return
# Explicitly passing a pd.Series to override default.
if isinstance(series, pd.Series):
return series
# Apply default if no series nor a default.
elif series is None or default is None:
return df[self.adjusted] if self.adjusted is not None else df[default]
# Ok. So it's a str.
elif isinstance(series, str):
# Return the df column since it's in there.
if series in df.columns:
return df[series]
else:
# Attempt to match the 'series' because it was likely misspelled.
matches = df.columns.str.match(series, case=False)
match = [i for i, x in enumerate(matches) if x]
# If found, awesome. Retur
没有合适的资源?快使用搜索试试~ 我知道了~
pandas_ta-0.1.66b.tar.gz
需积分: 1 0 下载量 60 浏览量
2024-03-17
13:29:22
上传
评论
收藏 53KB GZ 举报
温馨提示
Python库是一组预先编写的代码模块,旨在帮助开发者实现特定的编程任务,无需从零开始编写代码。这些库可以包括各种功能,如数学运算、文件操作、数据分析和网络编程等。Python社区提供了大量的第三方库,如NumPy、Pandas和Requests,极大地丰富了Python的应用领域,从数据科学到Web开发。Python库的丰富性是Python成为最受欢迎的编程语言之一的关键原因之一。这些库不仅为初学者提供了快速入门的途径,而且为经验丰富的开发者提供了强大的工具,以高效率、高质量地完成复杂任务。例如,Matplotlib和Seaborn库在数据可视化领域内非常受欢迎,它们提供了广泛的工具和技术,可以创建高度定制化的图表和图形,帮助数据科学家和分析师在数据探索和结果展示中更有效地传达信息。
资源推荐
资源详情
资源评论
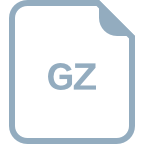
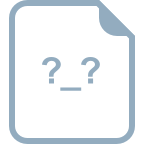
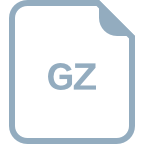
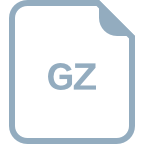
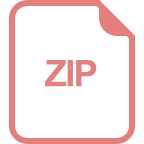
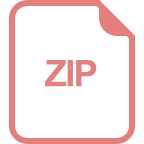
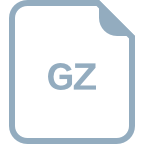
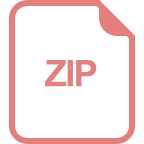
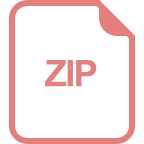
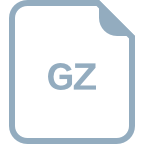
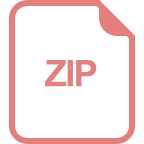
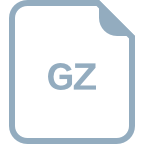
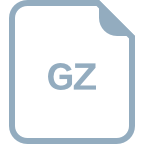
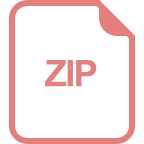
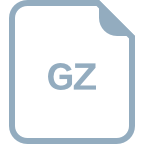
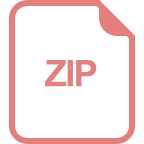
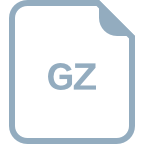
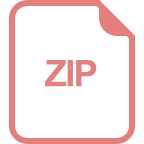
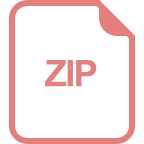
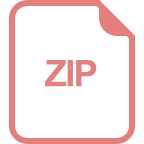
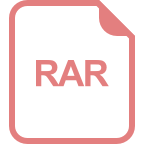
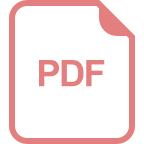
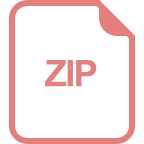
收起资源包目录

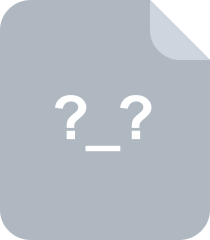
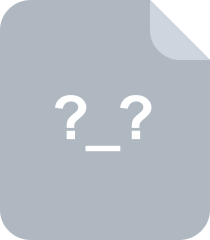
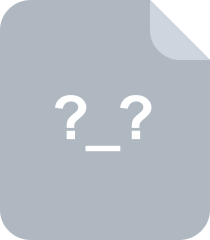
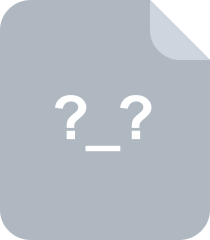
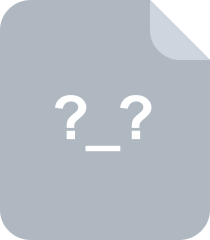
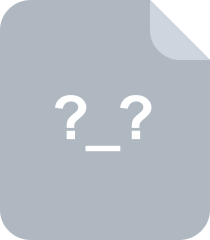
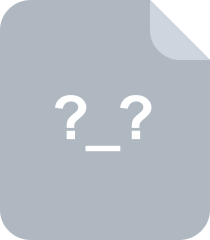
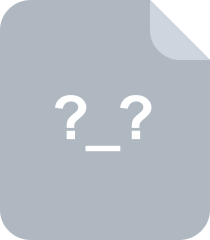
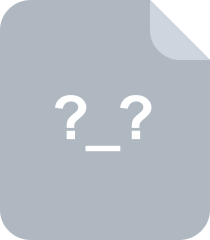
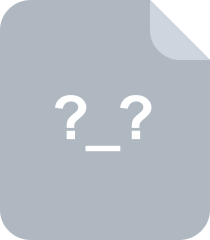
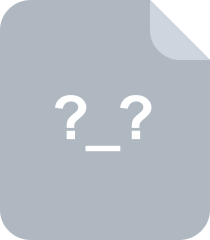
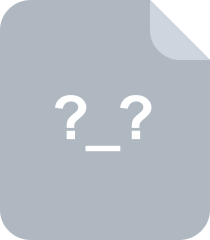
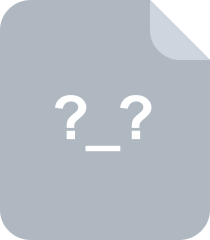
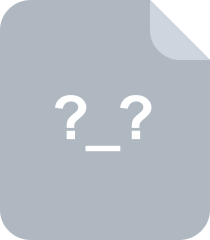
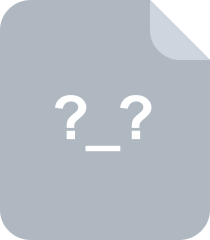
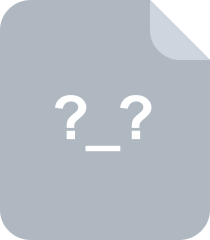
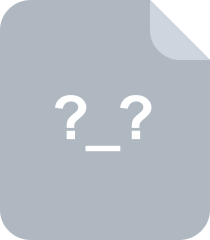
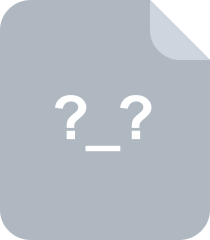
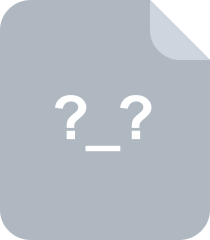
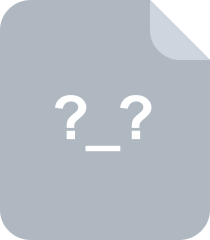
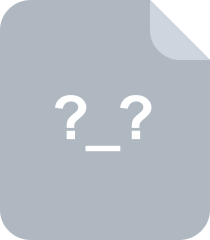
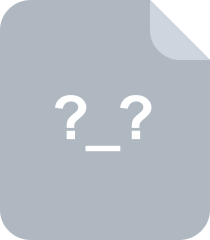
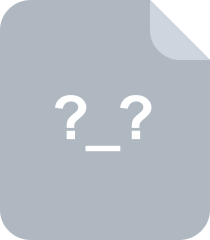
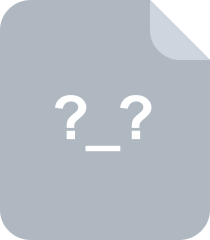
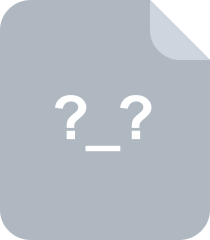
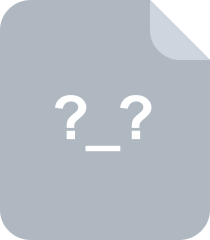
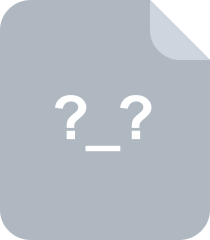
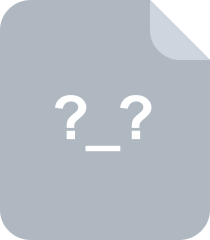
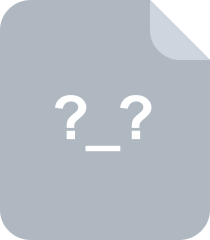
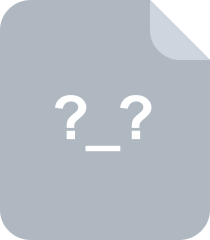
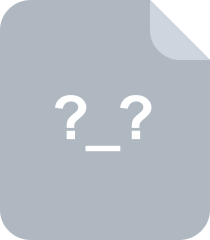
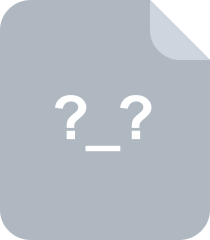
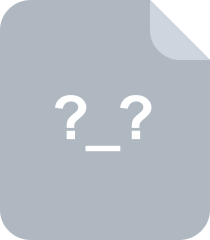
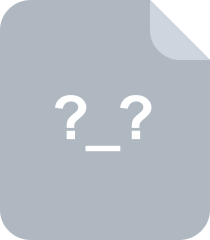
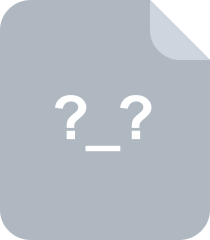
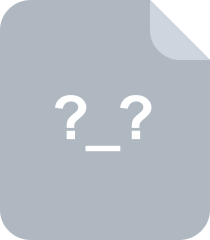
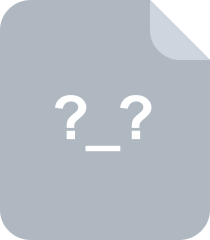
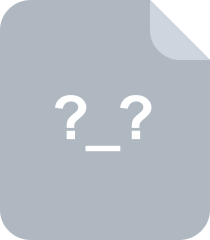
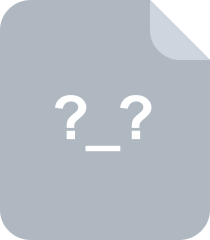
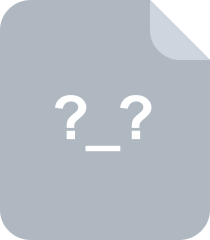
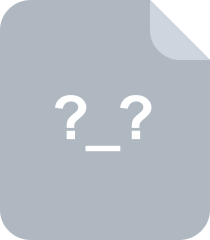
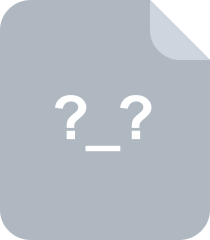
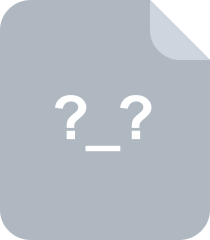
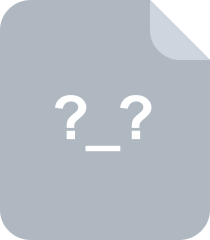
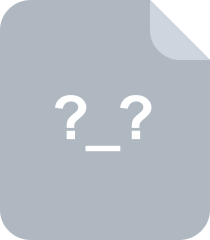
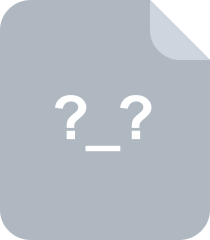
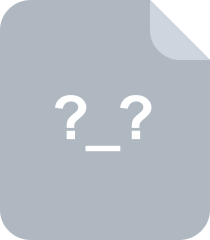
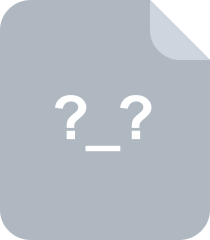
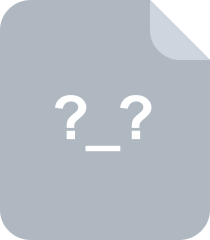
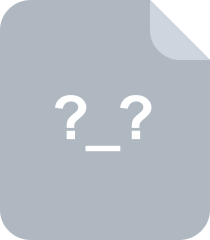
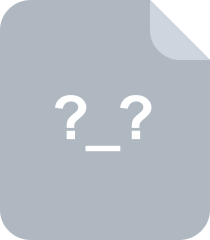
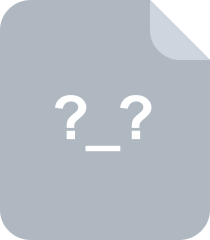
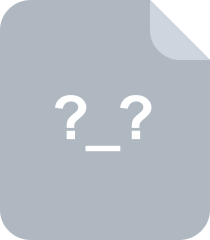
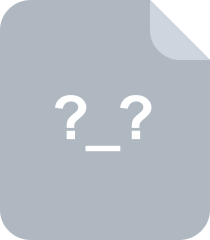
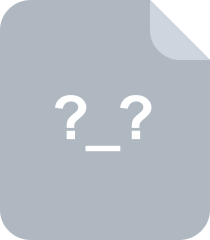
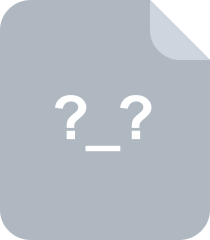
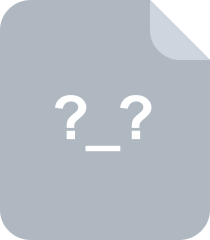
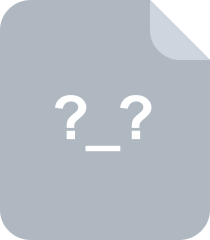
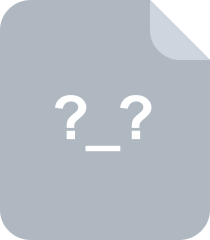
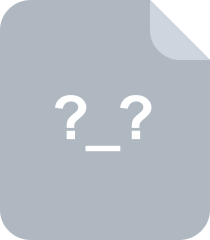
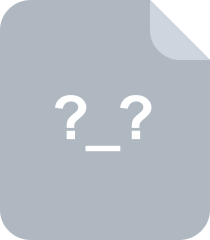
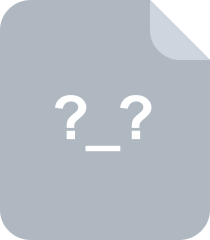
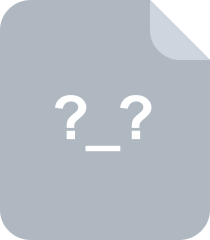
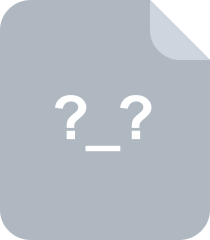
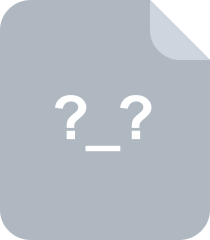
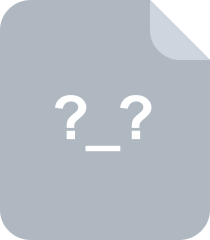
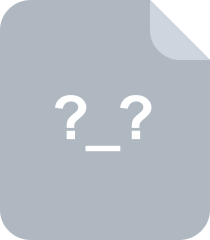
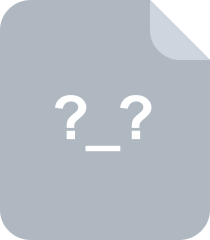
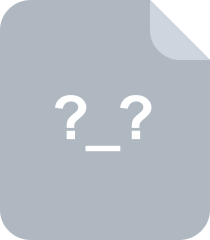
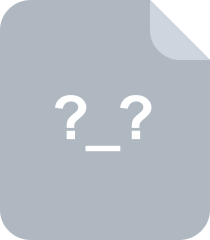
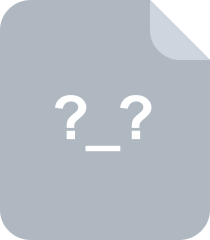
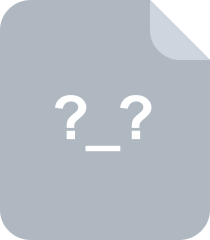
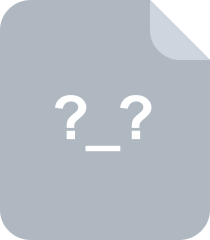
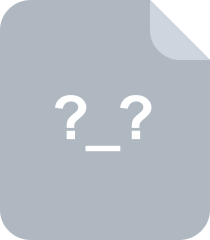
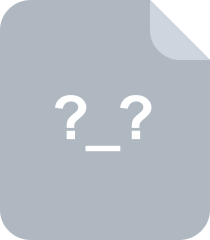
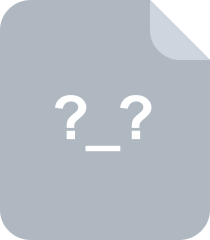
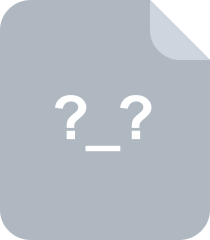
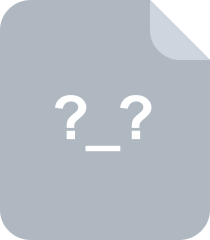
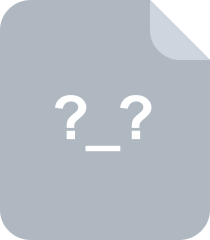
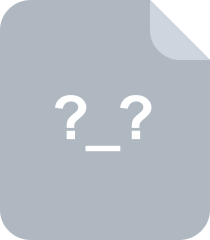
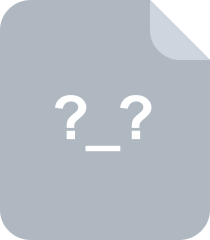
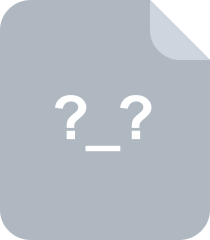
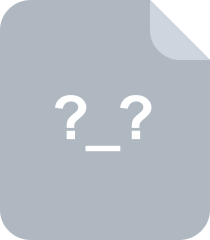
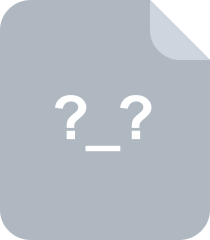
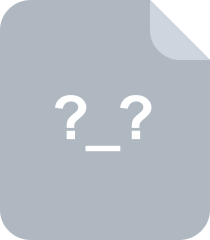
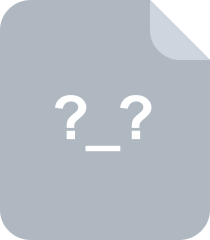
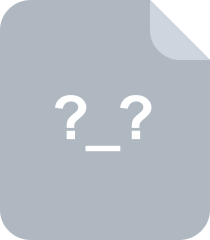
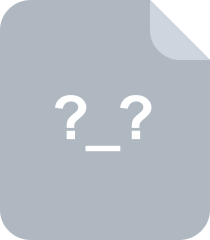
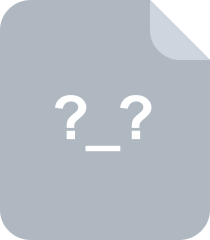
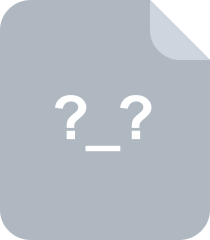
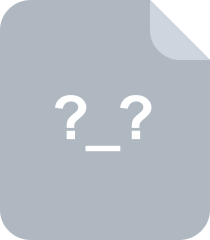
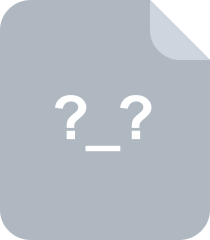
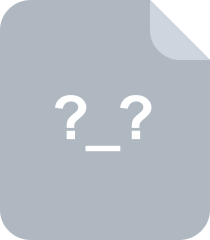
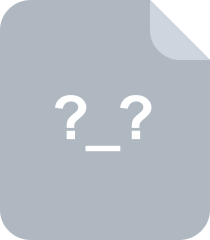
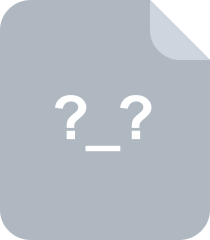
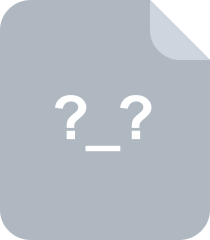
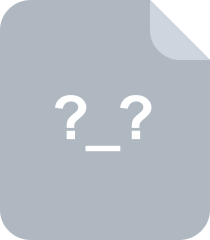
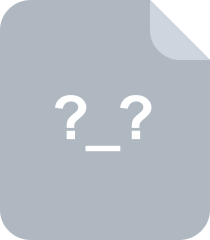
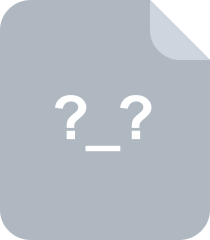
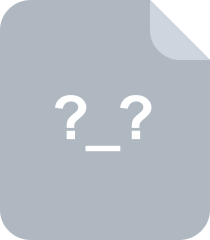
共 115 条
- 1
- 2
资源评论
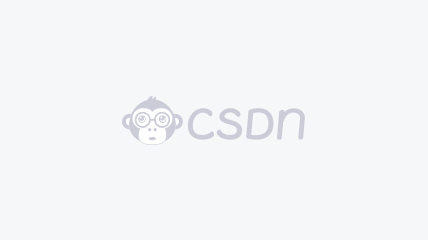

程序员Chino的日记
- 粉丝: 3743
- 资源: 5万+
上传资源 快速赚钱
我的内容管理 展开
我的资源 快来上传第一个资源
我的收益
登录查看自己的收益我的积分 登录查看自己的积分
我的C币 登录后查看C币余额
我的收藏
我的下载
下载帮助

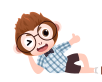
最新资源
- mamba 2 windows (cuda12.4)安装包,包括causal-conv1d,mamba-ssm
- simulink风储调频,风机混合储能电池超级电容储能联合一次调频,系统频率特性如下 电池储能采用双闭环pwm设计,频率控制环节为下垂控制 超级电容采用恒流充放电,降低电压释放存储在电容器中的能量
- OPC用户使用操作使用说明
- 0java实验报告.7z
- 两层液压梯程序,使用三菱FX1N系列PLC,已在设备上正常使用,非常稳定可靠,包含PLC程序
- zb25电气调车参考文档
- C++、基于MFC图像处理系统-2025
- JAVA基础编程练习:涵盖条件语句、循环结构、类和对象、继承多态及异常处理的应用案例集合
- zb45电气调车参考文档
- C++期末大作业-MFC 开发的Windows标准计算器和程序员计算器-2025
- Java编程实践:多位数字解析、文本检索、格式校验及其他基础练习
- python 的虚拟环境 原理
- C++-MFC框架为基础,结合opencv来对图像做处理-2025
- LSSVM,SSA-LSSVM,VMD-LSSVM,VMD-SSA-LSSVM四种算法做短期电力负荷预测,做对比 结果分析-lssvm 均方根误差(RMSE):0.79172 平均绝对误差(MAE)
- JAVA面向对象编程与异常处理核心技术解析及实际应用
- ZB45电气简化图册1
资源上传下载、课程学习等过程中有任何疑问或建议,欢迎提出宝贵意见哦~我们会及时处理!
点击此处反馈


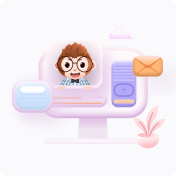
安全验证
文档复制为VIP权益,开通VIP直接复制
