"""Select axis labels (columns or index) of a data frame."""
import operator
from typing import Any, Callable, List, Optional, Sequence
try:
from typing import Literal
except ImportError:
from typing_extensions import Literal
from warnings import warn
import numpy as np
import pandas as pd
Indices = List[int]
class Selection:
"""Container for selection along a data frame axis with combination logic. """
def __init__(self, included:Optional[Indices]=None, excluded:Optional[Indices]=None, *, mask:Optional[Sequence[int]]=None):
"""
If ``mask`` is passed, ``included`` and ``excluded`` must be ``None``!
Parameters
----------
included:
List of indices included in the selection.
excluded:
List of indices excluded from the selection.
mask
Boolean array that will be converted to list of included
indices: All indices with corresponding truthy/non-zero value
will be included in the selection.
"""
if mask is not None:
if included is not None:
raise ValueError("included indices and mask cannot be passed together")
if excluded is not None:
raise ValueError("excluded indices and mask cannot be passed together")
included = np.nonzero(mask)[0].tolist()
self.included: Optional[Indices] = included
self.excluded: Optional[Indices] = excluded
def apply(self, axis:Literal["columns", "index"], df: pd.DataFrame):
labels = getattr(df, axis)
included = self.included
if included is None:
included = range(len(labels))
if self.excluded is not None:
excluded = set(self.excluded)
else:
excluded = set()
return labels[[i for i in included if not i in excluded]]
def __and__(self, other: "Selection") -> "Selection":
included=_combine_nones(self.included, other.included, intersect_indices)
excluded=_combine_nones(self.excluded, other.excluded, union_indices)
if included is not None and excluded is not None:
included = [i for i in included if i not in excluded]
return Selection(included, excluded)
def __or__(self, other: "Selection") -> "Selection":
included = _combine_nones(self.included, other.included, union_indices)
excluded = _combine_nones(self.excluded, other.excluded, intersect_indices)
if included is not None and excluded is not None:
excluded = [i for i in excluded if i not in included]
return Selection(included, excluded)
def __invert__(self) -> "Selection":
return Selection(self.excluded, self.included)
# Utilities to collect and combine column selections
def _combine_nones(a: Optional[Indices], b: Optional[Indices], fn_both:Callable[[Indices, Indices], Indices]) -> Optional[Indices]:
if a is None and b is None:
return None
if a is not None and b is None:
return a
if a is None and b is not None:
return b
return fn_both(a, b)
def intersect_indices(left: Indices, right: Indices) -> Indices:
r = []
for i in right:
if i in left:
r.append(i)
return r
def union_indices(left: Indices, right: Indices) -> Indices:
return left + [i for i in right if i not in left]
# Column selection operator closures
class BaseOp:
"""API definition of the closure object."""
def __call__(self, axis: Literal["columns", "index"], df: pd.DataFrame) -> Selection:
"""Evaluate operator on data frame from context."""
raise NotImplementedError("Must be implemented in sub-class.")
class LabelSelectionOp(BaseOp):
"""Explicitely select labels."""
def __init__(self, labels, level=None):
if isinstance(labels, list):
labels = tuple(labels)
elif not isinstance(labels, (slice, tuple)):
# Convert "scalar" values to some iterable
labels = (labels,)
self.labels = labels
self.level = level
def __call__(self, axis, df):
labels = getattr(df, axis)
idx = np.arange(len(labels))
if self.level is None:
cands = labels
else:
cands = labels.get_level_values(self.level)
indices = []
if isinstance(self.labels, tuple):
for lbl in self.labels:
indices.extend(idx[cands == lbl])
elif isinstance(self.labels, slice):
# NOTE: We need to make this more complex because we also need
# to treat situation with multiple repetitions of the same
# value, e.g., cases of multi-index levels.
in_slice = self.labels.start is None
reached_slice_stop = False
for i, lbl in enumerate(cands):
if not in_slice and lbl == self.labels.start:
in_slice = True
if reached_slice_stop and lbl != self.labels.stop:
# We stepped over the end of the slice.
break
if in_slice:
indices.append(i)
if self.labels.stop is not None and lbl == self.labels.stop:
reached_slice_stop = True
else:
# This should never be reached becaus of the argument processing
# in __init__.
raise ValueError(f"Unexpected type for self.labels: {type(self.labels)}: {self.labels!r}")
return Selection(indices)
def __str__(self):
if isinstance(self.labels, slice):
fmt = lambda o, default: repr(o) if o else default
items = [fmt(self.labels.start, ''), fmt(self.labels.stop, '')]
if self.labels.step:
items.append(repr(self.labels.step))
pp_labels = ':'.join(items)
else:
pp_labels = ', '.join(str(l) for l in self.labels)
if self.level:
return f'(level={self.level})[{pp_labels}]'
return f'[{pp_labels}]'
class LabelPredicateOp(BaseOp):
"""Select labels by a predicate, e.g. ``startswith``."""
def __init__(self, meth, args, kwargs, level=None):
self.meth = meth
self.args = args
self.kwargs = kwargs
self.level = level
def __str__(self):
def pp(a):
if isinstance(a, tuple):
return [repr(i) for i in a]
elif isinstance(a, dict):
return [f'{k}={v!r}' for k, v in a.items()]
return [repr(a)]
pp_args = ', '.join(pp(self.args) + pp(self.kwargs))
if self.level:
return f'(level={self.level}).{self.meth}({pp_args})'
return f'.{self.meth}({pp_args})'
def __call__(self, axis, df: pd.DataFrame) -> Selection:
labels = getattr(df, axis)
if self.level is None:
str_accessor = labels.str
else:
str_accessor = labels.get_level_values(self.level).str
meth = getattr(str_accessor, self.meth)
mask = meth(*self.args, **self.kwargs)
return Selection(mask=mask)
class EllipsisOp(BaseOp):
"""Select all columns."""
def __call__(self, axis, df: pd.DataFrame) -> Selection:
labels = getattr(df, axis)
return Selection(mask=np.ones(len(labels), dtype=bool))
def __str__(self):
return '...'
class BinaryOp(BaseOp):
"""Combine two operators."""
def __init__(self, left: BaseOp, right: BaseOp, op: Callable[[Any, Any], Any]):
self.left = left
self.right = right
self.op = op
def __str__(self):
op_name = getattr(self.op, '__name__', str(self.op))
return f'({self.left}) {op_name} ({self.right})'
def __call__(self, axis, df: pd.DataFrame) -> Selection:
sel_left = self.left(axis, df)
sel_right = self.right(axis, df)
return self.op(sel_left, sel_right)
class

程序员Chino的日记
- 粉丝: 3719
- 资源: 5万+
最新资源
- HTML5实现好看的水上运动俱乐部网站源码.zip
- HTML5实现好看的私人水疗会所网页模板.zip
- HTML5实现好看的外贸商务合作公司网站模板.zip
- HTML5实现好看的涂料粉刷公司官网网站源码.zip
- HTML5实现好看的外卖订餐平台网站模板.zip
- HTML5实现好看的野生动物园网站源码.zip
- HTML5实现好看的网红沙发茶几网站源码.zip
- HTML5实现好看的响应式蓝色商城网站源码.zip
- HTML5实现好看的在线课堂教育培训网站源码.zip
- HTML5实现好看的婴儿护理教育网站源码.zip
- HTML5实现好看的游戏门户新闻网站源码.zip
- HTML5实现好看的珠宝首饰电商网站源码.zip
- HTML5实现好看的智能家居互联网产品网站源码.zip
- 苹果、柠檬、人检测3-YOLO(v5至v11)、COCO、CreateML、Paligemma、TFRecord、VOC数据集合集.rar
- 使用Python编程实现圣诞树图形绘制
- 基于多时间尺度的灵活性资源优化配置 关键词:多时间尺度;模型预测控制;日内滚动优化; 1. 程序:matlab-yalmip-cplex 2.设备:以包含风力场、光伏电站、微型燃气轮机、蓄电池、余热锅
资源上传下载、课程学习等过程中有任何疑问或建议,欢迎提出宝贵意见哦~我们会及时处理!
点击此处反馈


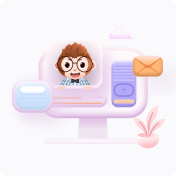