[](https://conventionalcommits.org)



[](https://opensource.org/licenses/MIT)
The package contains just a few features that make using pandas categorical types easier to use.
The main purpose of using categorical types is to reduce RAM consumption when working with large datasets. Experience shows that on average, there is a decrease of 2 times (for datasets of several GB, this is very significant). The full justification of the reasons and examples will be given below.
# Quickstart
```
pip install pandas-categorical
```
```python
import pandas as pd
import pandas-categorical as pdc
```
```python
df.astype('category') -> pdc.cat_astype(df, ...)
pd.concat() -> pdc.concat_categorical()
pd.merge() -> pdc.merge_categorical()
df.groupby(...) -> df.groupby(..., observed=True)
```
## cat_astype
```python
df = pd.read_csv("path_to_dataframe.csv")
SUB_DTYPES = {
'cat_col_with_int_values': int,
'cat_col_with_string_values': 'string',
'ordered_cat_col_with_bool_values': bool,
}
pdc.cat_astype(
data=df,
cat_cols=SUB_DTYPES.keys(),
sub_dtypes=SUB_DTYPES,
ordered_cols=['ordered_cat_col_with_bool_values']
)
```
## concat_categorical
```python
df_1 = ... # dataset with some categorical columns
df_2 = ... # dataset with some categorical columns (categories are not equals)
df_res = pdc.concat_categorical((df_1, df_2), axis=0, ignore_index=True)
```
## merge_categorical
```python
df_1 = ... # dataset with some categorical columns
df_2 = ... # dataset with some categorical columns (categories are not equals)
df_res = pdc.merge_categorical(df_1, df_2, on=['cat_col_1', 'cat_col_2'])
```
# A bit of theory
The advantages are discussed in detail in the articles [here](https://towardsdatascience.com/staying-sane-while-adopting-pandas-categorical-datatypes-78dbd19dcd8a), [here](https://towardsdatascience.com/pandas-groupby-aggregate-transform-filter-c95ba3444bbb) and [here](https://pandas.pydata.org/docs/user_guide/categorical.html).
The categorical type implies the presence of a certain set of unique values in this column, which are often repeated. By reducing the copying of identical values, it is possible to reduce the size of the column (the larger the dataset, the more likely repetitions are). By default, categories (unique values) have no order. That is, they are not comparable to each other. It is possible to make them ordered.
Pandas already has everything for this (for example, `.astype(’category’)`). However, standard methods, in my opinion, require a high entry threshold and therefore are rarely used.
Let's try to outline a number of problems and ways to solve them.
## 1. Categorical types are easy to lose
Suppose you want to connect two datasets into one using `pd.concat(..., axis=0)`. Datasets contain columns with categorical types.
If the column categories of the source datasets are different, then `pandas` it does not combine multiple values, but simply resets their default type (for example, `object`, `int`, ...).
In other words,
$$\textcolor{red}{category1} + \textcolor{green}{category2} = object$$
$$\textcolor{red}{category1} + \textcolor{red}{category1} = \textcolor{red}{category1}$$
But we would like to observe a different behavior:
$$\textcolor{red}{category1} + \textcolor{green}{category2} = \textcolor{blue}{category3}$$
$$(\textcolor{blue}{category3} = \textcolor{red}{category1} \cup \textcolor{green}{category2})$$
As a result, you need to monitor the reduction of categories before actions such as `merge` or `concat`.
## 2. Categories type control
When you do a type conversion
```python
df['col_name'] = df['col_name'].astype('category')
```
the type of categories is equal to the type of the source column.
But, if you want to change the type of categories, you probably want to write something like
```python
df['col_name'] = df['col_name'].astype('some_new_type').astype('category')
```
That is, you will temporarily lose the categorical type (respectively, and the advantages of memory).
By the way, the usual way of control
```python
df.dtypes
```
does not display information about the type of categories themselves. You will only see only `category` next to the desired column.
## 3. Unused values
Suppose you have filtered the dataset. At the same time, the actual set of values of categorical columns could decrease, but the data type will not be changed.
This can negatively affect, for example, when working with `groupby` on such a column. As a result, grouping will also occur by unused categories. To prevent this from happening, you need to specify the `observed=True` parameter.
For example,
```python
df.groupby(['cat_col_1', 'cat_col_2'], observed=True).agg('mean')
```
## 4. Ordered categories
There is a understandable instruction for converting a column type to a categorical (unordered) one
```python
df[col] = df[col].astype('category')
```
But there is no similar command to convert to an ordered categorical type.
There are two non-obvious ways:
```python
df[col] = df[col].astype('category').cat.as_ordered()
```
Or
```python
df[col] = df[col].astype(pd.CategoricalDtype(ordered=True))
```
## 5. Minimum copying
To process large datasets, you need to minimize the creation of copies of even its parts. Therefore, the functions from this package do the transformations in place.
## 6. Data storage in parquet format
When using `pd.to_parquet(path, engine='pyarrow')` and `pd.read_parque(path, engine='pyarrow')`categorical types of some columns can be reset to normal. To solve this problem, you can use `engine='fastparquet'`.
Note 1: `fastparquet` usually runs a little slower than `pyarrow`.
Note 2: `pyarrow` and `fastparquet` cannot be used together (for example, save by one and read by the other). This can lead to data loss.
```python
import pandas as pd
df = pd.DataFrame(
{
"Date": pd.date_range('2023-01-01', periods=10),
"Object": ["a"]*5+["b"]+["c"]*4,
"Int": [1, 1, 1, 2, 3, 1, 2, 4, 3, 2],
"Float": [1.1]*5+[2.2]*5,
}
)
print(df.dtypes)
df = df.astype('category')
print(df.dtypes)
df.to_parquet('test.parquet', engine='pyarrow')
df = pd.read_parquet('test.parquet', engine='pyarrow')
print(df.dtypes)
```
Output:
```
Date datetime64[ns]
Object object
Int int64
Float float64
dtype: object
Date category
Object category
Int category
Float category
dtype: object
Date datetime64[ns]
Object category
Int int64
Float float64
dtype: object
```
# Examples
- [Jupiter notebook with examples](https://www.kaggle.com/code/loskost/problems-of-pandas-categorical-dtypes) of problems is posted on kaggle. A copy can be found in the `examples/` folder.
- [Jupiter notebook with solution](https://www.kaggle.com/code/loskost/problems-of-pandas-categorical-dtypes-solution) of problems. A copy can be found in the `examples/` folder.
- Also, usage examples can be found in the tests folder.
# Remarks
1. Processing of categorical indexes has not yet been implemented.
2. In the future, the function `pdc.join_categorical()` will appear.
3. The `cat_astype` function was designed so that the type information could be redundant (for example, it is specified for all possible column names in the project at once). In the future, it will be possible to set default values for this function.
# Links
1. [Official pandas documentation](https://pandas.pydata.org/docs/user_guide/categorical.html).
2. https://towardsdatascience.com/staying-sane-while-adopting-pandas-categorical-datatypes-78dbd19dcd8a
3. htt
没有合适的资源?快使用搜索试试~ 我知道了~
pandas_categorical-1.0.2.tar.gz
需积分: 1 0 下载量 136 浏览量
2024-03-11
16:22:45
上传
评论
收藏 6KB GZ 举报
温馨提示
Python库是一组预先编写的代码模块,旨在帮助开发者实现特定的编程任务,无需从零开始编写代码。这些库可以包括各种功能,如数学运算、文件操作、数据分析和网络编程等。Python社区提供了大量的第三方库,如NumPy、Pandas和Requests,极大地丰富了Python的应用领域,从数据科学到Web开发。Python库的丰富性是Python成为最受欢迎的编程语言之一的关键原因之一。这些库不仅为初学者提供了快速入门的途径,而且为经验丰富的开发者提供了强大的工具,以高效率、高质量地完成复杂任务。例如,Matplotlib和Seaborn库在数据可视化领域内非常受欢迎,它们提供了广泛的工具和技术,可以创建高度定制化的图表和图形,帮助数据科学家和分析师在数据探索和结果展示中更有效地传达信息。
资源推荐
资源详情
资源评论
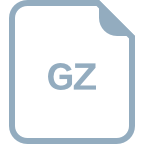
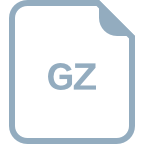
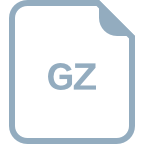
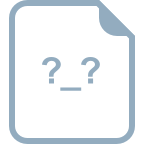
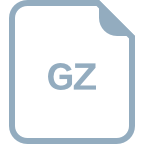
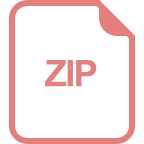
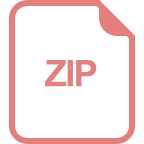
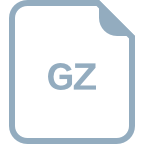
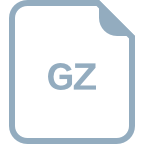
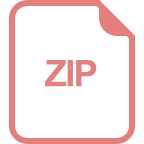
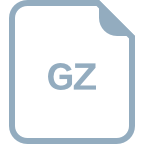
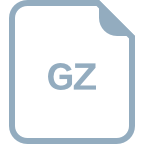
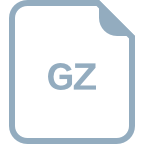
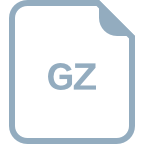
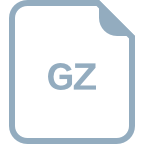
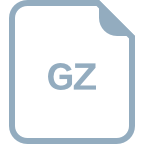
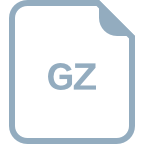
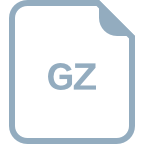
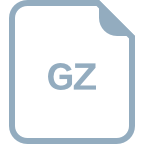
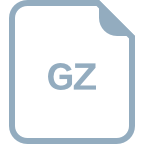
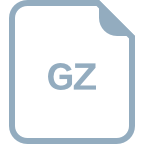
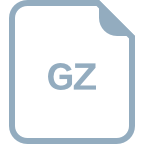
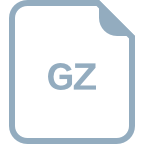
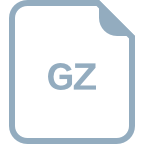
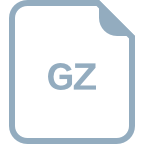
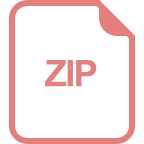
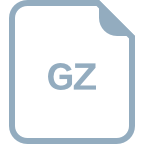
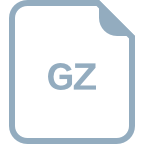
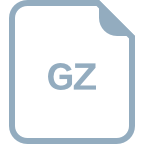
收起资源包目录


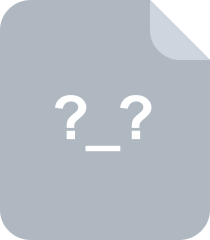
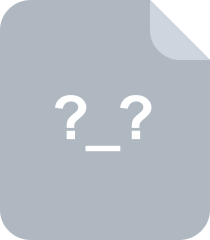

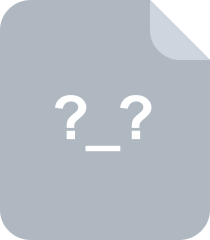
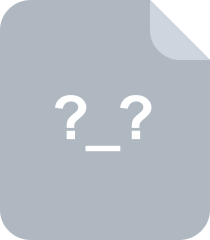
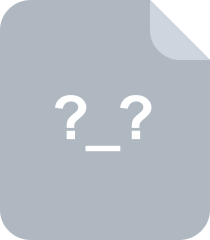
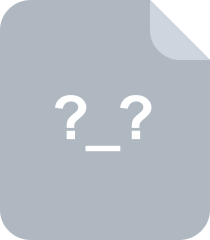
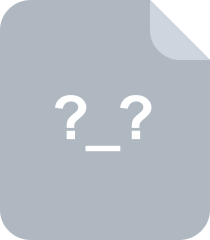
共 7 条
- 1
资源评论
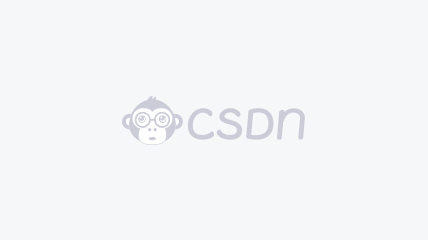

程序员Chino的日记
- 粉丝: 3664
- 资源: 5万+
上传资源 快速赚钱
我的内容管理 展开
我的资源 快来上传第一个资源
我的收益
登录查看自己的收益我的积分 登录查看自己的积分
我的C币 登录后查看C币余额
我的收藏
我的下载
下载帮助

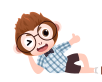
最新资源
- LitJson(0.19.0版本,适用于.NetStandard2.0)
- LitJson(0.19.0版本,适用于.NetStandard1.5)
- (源码)基于ROS的咖啡机器人控制系统.zip
- (源码)基于Qt和OpenCV的图像拼接系统.zip
- 《信号与系统》编程作业.zip
- (源码)基于C#的二级文件系统模拟.zip
- (源码)基于C++的巡飞弹三自由度弹道仿真系统.zip
- (源码)基于SpringBoot和Redis的短链接生成系统.zip
- (源码)基于Qt和GStreamer的条形码扫描系统.zip
- Apache Dubbo 是一个高性能的、基于 Java 的开源 RPC 框架 dubbo源码
资源上传下载、课程学习等过程中有任何疑问或建议,欢迎提出宝贵意见哦~我们会及时处理!
点击此处反馈


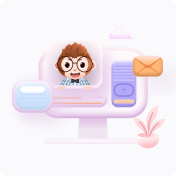
安全验证
文档复制为VIP权益,开通VIP直接复制
