Pandas Downcast
===============
[](https://pypi.python.org/pypi/pandas-downcast)
[](https://pypi.python.org/pypi/pandas-downcast/)
[](https://travis-ci.com/domvwt/pandas-downcast)
[](https://codecov.io/gh/domvwt/pandas-downcast)
Shrink [Pandas](https://pandas.pydata.org/) DataFrames with precision safe schema inference.
`pandas-downcast` finds the minimum viable type for each column, ensuring that resulting values
are within tolerance of original values.
## Installation
```bash
pip install pandas-downcast
```
## Dependencies
* python >= 3.6
* pandas
* numpy
## License
[MIT](https://opensource.org/licenses/MIT)
## Usage
```python
import pdcast as pdc
import numpy as np
import pandas as pd
data = {
"integers": np.linspace(1, 100, 100),
"floats": np.linspace(1, 1000, 100).round(2),
"booleans": np.random.choice([1, 0], 100),
"categories": np.random.choice(["foo", "bar", "baz"], 100),
}
df = pd.DataFrame(data)
# Downcast DataFrame to minimum viable schema.
df_downcast = pdc.downcast(df)
# Infer minimum schema from DataFrame.
schema = pdc.infer_schema(df)
# Coerce DataFrame to schema - required if converting float to Pandas Integer.
df_new = pdc.coerce_df(df, schema)
```
Smaller data types == smaller memory footprint.
```python
df.info()
# <class 'pandas.core.frame.DataFrame'>
# RangeIndex: 100 entries, 0 to 99
# Data columns (total 4 columns):
# # Column Non-Null Count Dtype
# --- ------ -------------- -----
# 0 integers 100 non-null float64
# 1 floats 100 non-null float64
# 2 booleans 100 non-null int64
# 3 categories 100 non-null object
# dtypes: float64(2), int64(1), object(1)
# memory usage: 3.2+ KB
df_downcast.info()
# <class 'pandas.core.frame.DataFrame'>
# RangeIndex: 100 entries, 0 to 99
# Data columns (total 4 columns):
# # Column Non-Null Count Dtype
# --- ------ -------------- -----
# 0 integers 100 non-null uint8
# 1 floats 100 non-null float32
# 2 booleans 100 non-null bool
# 3 categories 100 non-null category
# dtypes: bool(1), category(1), float32(1), uint8(1)
# memory usage: 932.0 bytes
```
Numerical data types will be downcast if the resulting values are within tolerance of the original values.
For details on tolerance for numeric comparison, see the notes on [`np.allclose`](https://numpy.org/doc/stable/reference/generated/numpy.allclose.html).
```python
print(df.head())
# integers floats booleans categories
# 0 1.0 1.00 1 foo
# 1 2.0 11.09 0 baz
# 2 3.0 21.18 1 bar
# 3 4.0 31.27 0 bar
# 4 5.0 41.36 0 foo
print(df_downcast.head())
# integers floats booleans categories
# 0 1 1.000000 True foo
# 1 2 11.090000 False baz
# 2 3 21.180000 True bar
# 3 4 31.270000 False bar
# 4 5 41.360001 False foo
print(pdc.options.ATOL)
# >>> 1e-08
print(pdc.options.RTOL)
# >>> 1e-05
```
Tolerance can be set at module level or passed in function arguments.
```python
pdc.options.ATOL = 1e-10
pdc.options.RTOL = 1e-10
df_downcast_new = pdc.downcast(df)
```
Or
```python
infer_dtype_kws = {
"ATOL": 1e-10,
"RTOL": 1e-10
}
df_downcast_new = pdc.downcast(df, infer_dtype_kws=infer_dtype_kws)
```
The `floats` column is now kept as `float64` to meet the tolerance requirement.
Values in the `integers` column are still safely cast to `uint8`.
```python
df_downcast_new.info()
# <class 'pandas.core.frame.DataFrame'>
# RangeIndex: 100 entries, 0 to 99
# Data columns (total 4 columns):
# # Column Non-Null Count Dtype
# --- ------ -------------- -----
# 0 integers 100 non-null uint8
# 1 floats 100 non-null float64
# 2 booleans 100 non-null bool
# 3 categories 100 non-null category
# dtypes: bool(1), category(1), float64(1), uint8(1)
# memory usage: 1.3 KB
```
## Example
The following example shows how downcasting data often leads to size reductions of **greater than 70%**, depending on the original types.
```python
import pdcast as pdc
import pandas as pd
import seaborn as sns
df_dict = {df: sns.load_dataset(df) for df in sns.get_dataset_names()}
results = []
for name, df in df_dict.items():
size_pre = df.memory_usage(deep=True).sum()
df_post = pdc.downcast(df)
size_post = df_post.memory_usage(deep=True).sum()
shrinkage = int((1 - (size_post / size_pre)) * 100)
results.append(
{"dataset": name, "size_pre": size_pre, "size_post": size_post, "shrink_pct": shrinkage}
)
results_df = pd.DataFrame(results).sort_values("shrink_pct", ascending=False).reset_index(drop=True)
print(results_df)
```
```
dataset size_pre size_post shrink_pct
0 fmri 213232 14776 93
1 titanic 321240 28162 91
2 attention 5888 696 88
3 penguins 75711 9131 87
4 dots 122240 17488 85
5 geyser 21172 3051 85
6 gammas 500128 108386 78
7 anagrams 2048 456 77
8 planets 112663 30168 73
9 anscombe 3428 964 71
10 iris 14728 5354 63
11 exercise 3302 1412 57
12 flights 3616 1888 47
13 mpg 75756 43842 42
14 tips 7969 6261 21
15 diamonds 3184588 2860948 10
16 brain_networks 4330642 4330642 0
17 car_crashes 5993 5993 0
```

程序员Chino的日记
- 粉丝: 3740
- 资源: 5万+
最新资源
- 3b015大学生创业项目管理系统_springboot+vue0.zip
- x86-64架构下gmssl工具
- 3b016个性化课程推荐系统_springboot+vue.zip
- 电影订票及评论网站的设计与实现-springboot毕业项目,适合计算机毕-设、实训项目、大作业学习.zip
- 3b014宠物猫店管理系统_springboot+vue.zip
- 仓库管理系统pf-springboot毕业项目,适合计算机毕-设、实训项目、大作业学习.zip
- 洞见研报Geek+(智能物流机器人研发商,北京极智嘉科技股份有限公司)创投信息
- 付费问答系统的设计与实现-springboot毕业项目,适合计算机毕-设、实训项目、大作业学习.rar
- 3b017旅游景区预约服务系统_springboot+vue0.zip
- 新版在线生成一合三网站缩微图工具PHP源码
- 基于BS的社区物业管理系统-springboot毕业项目,适合计算机毕-设、实训项目、大作业学习.rar
- 基于Java的美妆购物网站的设计与实现-springboot毕业项目,适合计算机毕-设、实训项目、大作业学习.rar
- 基于HTML语言的环保网站的设计与实现-springboot毕业项目,适合计算机毕-设、实训项目、大作业学习.rar
- 德普微一级代理 DP040N04DTL TO-252 DPMOS N-MOSFET 40V 100A 3.2mΩ
- 3b019企业人事管理系统_springboot+vue.zip
- 3b018企业人力资源管理系统_springboot+vue.zip
资源上传下载、课程学习等过程中有任何疑问或建议,欢迎提出宝贵意见哦~我们会及时处理!
点击此处反馈


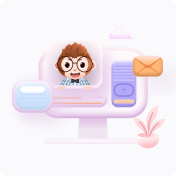