import tensorflow as tf
from tensorflow.examples.tutorials.mnist import input_data
# disable debugging logs
tf.logging.set_verbosity(tf.logging.ERROR) # tf.logging.WARN
# import data set
mnist = input_data.read_data_sets("data\MNIST", one_hot=True)
# set placeholders
x = tf.placeholder(tf.float32, [None, 784])
y_ = tf.placeholder(tf.float32, [None, 10])
# vector to matrix
x_image = tf.reshape(x, [-1, 28, 28, 1])
# generating weight
def weight_variable(shape):
initial = tf.truncated_normal(shape, stddev=0.1)
return tf.Variable(initial)
# generating bias
def bias_variable(shape):
initial = tf.constant(0.1, shape=shape)
return tf.Variable(initial)
# convolution
def conv2d(x, W):
return tf.nn.conv2d(x, W, strides=[1, 1, 1, 1], padding='SAME')
# pooling
def max_pool_2x2(x):
return tf.nn.max_pool(x, ksize=[1, 2, 2, 1], strides=[1, 2, 2, 1], padding='SAME')
# convolution layer 1
W_conv1 = weight_variable([5, 5, 1, 32])
b_conv1 = bias_variable([32])
h_conv1 = tf.nn.relu(conv2d(x_image, W_conv1) + b_conv1)
# pooling layer 1
h_pool1 = max_pool_2x2(h_conv1)
# convolution layer 2
W_conv2 = weight_variable([5, 5, 32, 64])
b_conv2 = bias_variable([64])
h_conv2 = tf.nn.relu(conv2d(h_pool1, W_conv2) + b_conv2)
# pooling layer 2
h_pool2 = max_pool_2x2(h_conv2)
# matrix to vector
h_pool2_flat = tf.reshape(h_pool2, [-1, 7 * 7 * 64])
# full connect layer 1
W_fc1 = weight_variable([7 * 7 * 64, 1024])
b_fc1 = bias_variable([1024])
h_fc1 = tf.nn.relu(tf.matmul(h_pool2_flat, W_fc1) + b_fc1)
# set dropout
keep_prob = tf.placeholder(tf.float32) # connect to noting, a parameter of session must be a placeholder
h_fc1_drop = tf.nn.dropout(h_fc1, keep_prob)
# full connect layer 2
W_fc2 = weight_variable([1024, 10])
b_fc2 = bias_variable([10])
y_conv = tf.matmul(h_fc1_drop, W_fc2) + b_fc2
# set cross entropy loss function
cross_entropy = tf.reduce_mean(tf.nn.softmax_cross_entropy_with_logits(labels=y_, logits=y_conv))
# set gradient descent optimizer
train_step = tf.train.AdamOptimizer(1e-4).minimize(cross_entropy)
# definite accuracy
correct_prediction = tf.equal(tf.argmax(y_conv, 1), tf.argmax(y_, 1))
accuracy = tf.reduce_mean(tf.cast(correct_prediction, tf.float32))
# create session
sess = tf.InteractiveSession()
# initialize variables
tf.global_variables_initializer().run()
# 1000 times gradient descent
for _ in range(20000):
batch = mnist.train.next_batch(50)
# print accuracy per 100 times
if _ % 100 == 0:
train_accuracy = accuracy.eval(feed_dict={x: batch[0], y_: batch[1], keep_prob: 1.0})
print("step %d, training accuracy %g" % (_, train_accuracy))
train_step.run(feed_dict={x: batch[0], y_: batch[1], keep_prob: 0.5})
# print testing accuracy
print("testing accuracy %g" % accuracy.eval(feed_dict={x: mnist.test.images, y_: mnist.test.labels, keep_prob: 1.0}))
没有合适的资源?快使用搜索试试~ 我知道了~
温馨提示
【资源说明】 1、该资源包括项目的全部源码,下载可以直接使用! 2、本项目适合作为计算机、数学、电子信息等专业的课程设计、期末大作业和毕设项目,作为参考资料学习借鉴。 3、本资源作为“参考资料”如果需要实现其他功能,需要能看懂代码,并且热爱钻研,自行调试。 基于《21个项目玩转深度学习》课程的项目实例源码.zip
资源推荐
资源详情
资源评论
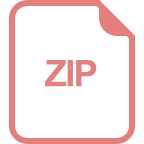
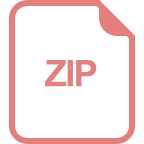
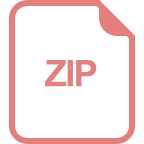
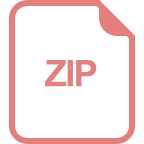
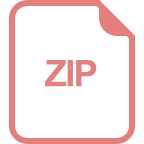
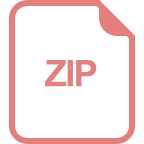
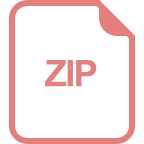
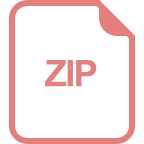
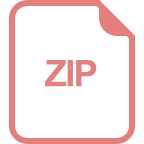
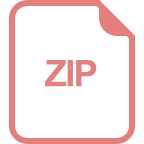
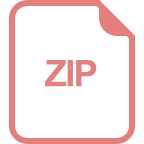
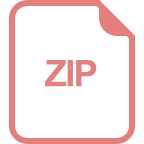
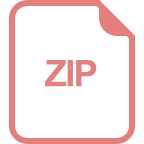
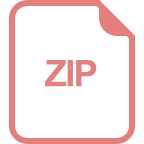
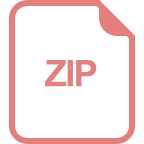
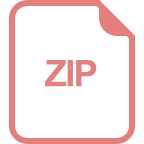
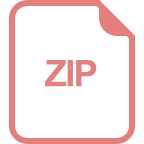
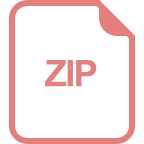
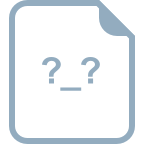
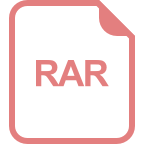
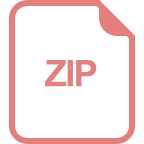
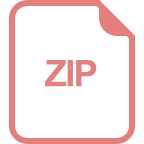
收起资源包目录




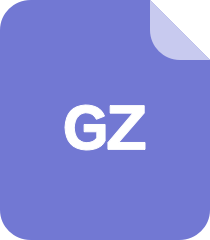
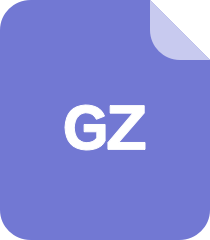
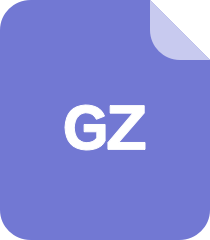
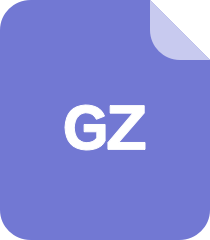
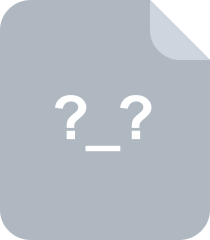
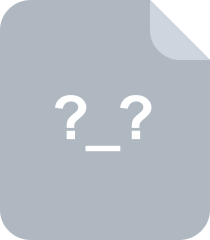
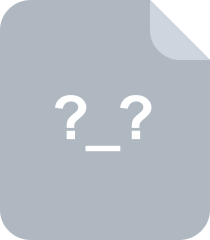
共 7 条
- 1
资源评论
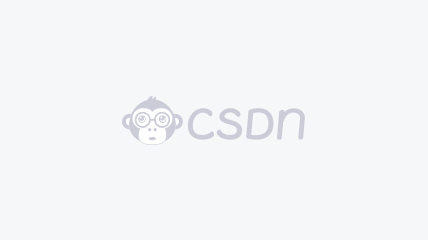

土豆片片
- 粉丝: 1572
- 资源: 5636
上传资源 快速赚钱
我的内容管理 展开
我的资源 快来上传第一个资源
我的收益
登录查看自己的收益我的积分 登录查看自己的积分
我的C币 登录后查看C币余额
我的收藏
我的下载
下载帮助

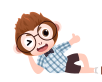
安全验证
文档复制为VIP权益,开通VIP直接复制
