import cv2
from utils.datasets import *
from utils.utils import *
import numpy as np
import colorsys
import os
import torch
import torch.nn as nn
import torch.backends.cudnn as cudnn
from torch.autograd import Variable
import math
import time
import csv
import lightTest
from utils.draw import draw_boxes,pil_draw_box_2
from licence import Licence
class YOLO(object):
_defaults = {
"model_path": 'yolo4_weights.pth',
"anchors_path": 'model_data/yolo_anchors.txt',
"classes_path": 'model_data/coco_classes.txt',
"model_image_size" : (416, 416, 3),
"confidence": 0.5,
"cuda": True
}
@classmethod
def get_defaults(cls, n):
if n in cls._defaults:
return cls._defaults[n]
else:
return "Unrecognized attribute name '" + n + "'"
def __init__(self, weights):
self.__dict__.update(self._defaults)
#self.class_names = self._get_class()
#self.anchors = self._get_anchors()
#self.generate()
self.dir=['UP','RIGHT','DOWN',"LEFT"]
self.currentCarID=0
self.virtureLine=[[0,0],[0,0]]
self.carCnt=0
self.motoCnt=0
self.personCnt=0
self.truckCnt=0
self.flag=False
self.trafficLine=None
self.trafficLight=[0,0,0,0]
self.curpath=0
self.trafficLightColor=None
self.device=torch.device("cuda:0" if torch.cuda.is_available() else "cpu")
self.model=torch.load(weights,map_location=self.device)['model'].float()
self.model.to(self.device).eval()
self.names = self.model.module.names if hasattr(self.model, 'module') else self.model.names
self.colors = [[random.randint(0, 255) for _ in range(3)] for _ in range(len(self.names))]
self.licence=Licence()
self.leftlight=[]
self.forwardlight=[]
self.rightlight=[]
self.leftlightColor=0
self.forwardlightColor=0
self.rightlightColor=0
self.carDirection={}
self.config=None
def detect_image(self,img,trafficline,path,idx_frame,illegal,config):
self.config=config
self.leftlight=self.config.leftlight
#print(self.leftlight)
self.forwardlight=self.config.forwardlight
self.rightlight=self.config.rightlight
self.trafficLine=trafficline
self.curpath=path
#(filepath,filename)=os.path.split(path)
self.personCnt=self.carCnt=self.motoCnt=self.truckCnt=0
im0=img.copy()
image=im0
half=self.device.type!='cpu'
if half:
self.model.half()
img = letterbox(im0,new_shape=(640,640))[0]
img = img[:, :, ::-1].transpose(2, 0, 1) # BGR to RGB, to 3x416x416
img = np.ascontiguousarray(img)
img = torch.from_numpy(img).to(self.device)
img = img.half() if half else img.float() # uint8 to fp16/32
img /= 255.0 # 0 - 255 to 0.0 - 1.0
if img.ndimension() == 3:
img = img.unsqueeze(0)
pred = self.model(img)[0]
pred = non_max_suppression(pred, 0.4, 0.5)
cars=[]
return_boxs=[]
return_class_names=[]
return_scores=[]
for i, det in enumerate(pred): # detections per image
if det is not None and len(det):
#print(det)
det[:, :4] = scale_coords(img.shape[2:], det[:, :4], im0.shape).round()
# Write results
for *xyxy, conf, cls in det:
c=cls
x=int(xyxy[0])
y=int(xyxy[1])
w=int(xyxy[2]-xyxy[0])
h=int(xyxy[3]-xyxy[1])
label = '%s %.2f' % (self.names[int(cls)], conf)
if (self.config.preflag[3]==1 or self.config.preflag[4]==1 or self.config.preflag[5]==1) :
#self.flag=True
pass
else:
if c==9:
if self.flag:
if y<self.trafficLight[1]:
self.trafficLight=xyxy
w=int(xyxy[2]-xyxy[0])
h=int(xyxy[3]-xyxy[1])
if w>h:
self.config.leftlight=self.leftlight=[xyxy[0],xyxy[1],xyxy[0]+w/2,xyxy[3]]
self.config.forwardlight=self.forwardlight=[xyxy[0]+w/2,xyxy[1],xyxy[2],xyxy[3]]
continue
self.flag=True
self.trafficLight=xyxy
print(self.trafficLight)
w=int(xyxy[2]-xyxy[0])
h=int(xyxy[3]-xyxy[1])
if w>h:
self.config.leftlight=self.leftlight=[xyxy[0],xyxy[1],xyxy[0]+w/2,xyxy[3]]
self.config.forwardlight=self.forwardlight=[xyxy[0]+w/2,xyxy[1],xyxy[2],xyxy[3]]
print(self.config.leftlight)
print(self.config.forwardlight)
continue
plot_one_box(xyxy, im0, label=label, color=self.colors[int(cls)], line_thickness=3)
if c==2:
self.carCnt=self.carCnt+1
if c==0:
self.personCnt=self.personCnt+1
if os.path.exists(self.curpath+"illegal/"):
pass
else:
os.mkdir(self.curpath+"illegal")
if os.path.exists(self.curpath+"illegal/runred"):
pass
else:
os.mkdir(self.curpath+"illegal/runred")
if self.trafficLine!=None:
if self.trafficLightColor=='green' and x>=self.trafficLine[0] and x+w<=self.trafficLine[2] and conf>0.6 and (h/w>=1.6):
if idx_frame%8==0:
imgTmp=im0[y:y+h,x:x+w]
cv2.imwrite(self.curpath+"illegal/runred/"+str(idx_frame)+".jpg",imgTmp)
if isinstance(illegal.get(idx_frame,0),int):
illegal[idx_frame]={}
illegal[idx_frame].update({'runred':True})
font = ImageFont.truetype(font='model_data/simhei.ttf',size=np.floor(0.012 * np.shape(im0)[1] ).astype('int32'))
im0=pil_draw_box_2(im0,[x,y,x+w,y+h],label="闯红灯",font=font)
if c==3:
self.motoCnt=self.motoCnt+1
if c==7:
self.truckCnt=self.truckCnt+1
if c != 2 and c != 7:
continue
if(y+h<im0.shape[0]-12):
#continue
return_boxs.append([x+w/2,y+h/2,w,h])
return_class_names.append(self.names[int(cls)])
return_scores.append(conf)
if len(self.config.leftlight)>0 or len(self.config.forwardlight)>0 or len(self.config.rightlight)>0:
#print(self.config.leftlight)
if len(self.config.leftlight)>0:
x1=int(self.config.leftlight[0])
y1=int(self.config.leftl

babyai997
- 粉丝: 907
- 资源: 169
最新资源
- 视觉定位 完整软件 开发语言:C# + Halcon 1.流程可配置; 2.海康威视相机采图,可定制成其它品牌相机(Basler,映美精 等); 3.模板匹配; 4.定位指针,拟合圆,拟合矩形;跟
- NineAi+新版AI系统网站源码+ChatGPT
- 大型立式翻转切削加工生产线sw17可编辑全套技术资料100%好用.zip
- 西门子200smart模拟量滤波防抖PLC程序,能实现电流电压和热电阻模拟量信号的采集,有滤波,有高位和低位报警,采用for循环指令和间接寻址,让程序简单好用,并且针对程序,录制了视频讲解,详细的介绍
- 永磁同步电机矢量控制C代码,全部从项目中总结得到,采用的S-function模式仿真,与实际项目运行基本一致,可以直接复制代码移植到工程实践项目中去
- 交错并联BUCK变器仿真 输入电压范围:36~70V;输出电压:28.5V;电压电流双闭环PI控制 可 单片机芯片型号:dsPIC33FJ32MC204 仿真平台:proteus8.9;编译软件:M
- 单个试管检测加载机sw22全套技术资料100%好用.zip
- 电镀池-电解槽sw16可编辑全套技术资料100%好用.zip
- Buck 电路 simulink 仿真 buck 电路 电流开环控制 电流闭环控制 闭环控制包括:PID 控制,超前补偿,前馈控制,解耦控制 控制采用离散域进行控制, 各种控制方式下的参数整定还有
- Cortex-A系列SoC工程代码
- 有限元仿真模型一:视频教程(参考视频教程)+仿真模型-基于COMSOL多物理场耦合仿真的变压器流固耦合及振动噪声分析 1、变压器流固耦合仿真:分析变压器正常运行过程中涡流损耗以及迟滞损耗产生及传播过程
- 自动驾驶控制-基于运动学模型的MPC算法路径跟踪仿真 matlab和simulink联合仿真,运动学模型实现的MPC横向控制,可以跟踪双移线,五次多项式, 以及其他各种自定义路径
- 天然气水合物降压开采,基于COMSOL热-流-固多场耦合实现,同时可以表征开采过程中的储层孔隙度、渗透率的演化,考虑水平井筒环空高压充填石英砂层,有水平井和压裂水平井模型
- 开关磁阻电机SRM的PID参数优化 PID控制开关磁阻电机,粒子群算法优化PID参数(模型里面是matlab和simulink联合仿真)
- 新能源控制器,多峰值mppt寻优仿真模型,传统扰动电导等寻优无法用在局部遮阴下,而粒子群pso算法克服了这个问题,可用于自行研究 压缩包附带使用说明及解析文档,包括传统扰动与粒子群算法模型
- 35d四桥臂三维空间矢量调制算法仿真 四桥臂3DSVPWM调制算法仿真 电压型逆变器,图为负载电压 可实现单桥臂电压独立控制
资源上传下载、课程学习等过程中有任何疑问或建议,欢迎提出宝贵意见哦~我们会及时处理!
点击此处反馈


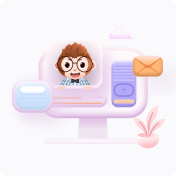