没有合适的资源?快使用搜索试试~ 我知道了~
Multiple Linear and 1D Regression
需积分: 9 3 下载量 65 浏览量
2018-10-02
20:23:37
上传
评论
收藏 4.7MB PDF 举报
温馨提示
Regression is the study of the conditional distribution Y |x of the response Y given the p × 1 vector of nontrivial predictors x. In a 1D regression model, Y is conditionally independent of x given a single linear combination α + βTx of the predictors, written Y x|(α + βTx) or Y x|βTx. Many of the most used statistical methods are 1D models, including generalized linear models such as multiple linear regression, logistic regression, and Poisson regression
资源推荐
资源详情
资源评论
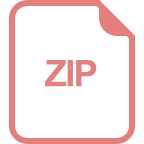
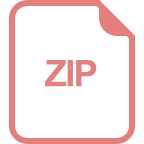
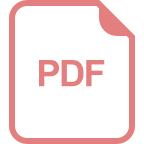
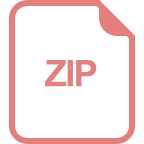
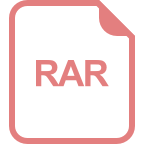
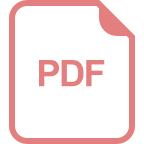
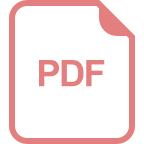
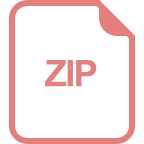
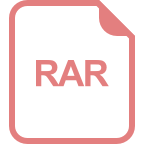
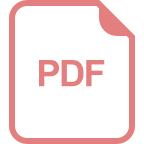
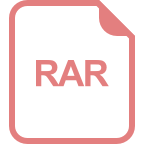
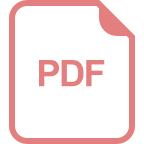
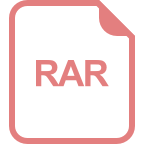
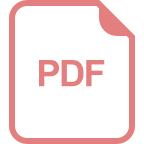
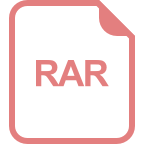
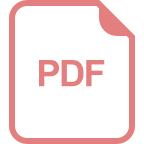
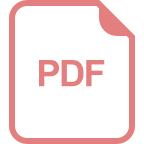
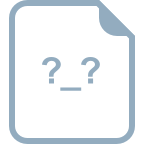
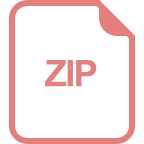
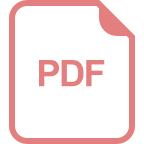
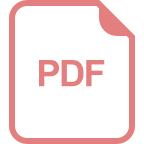
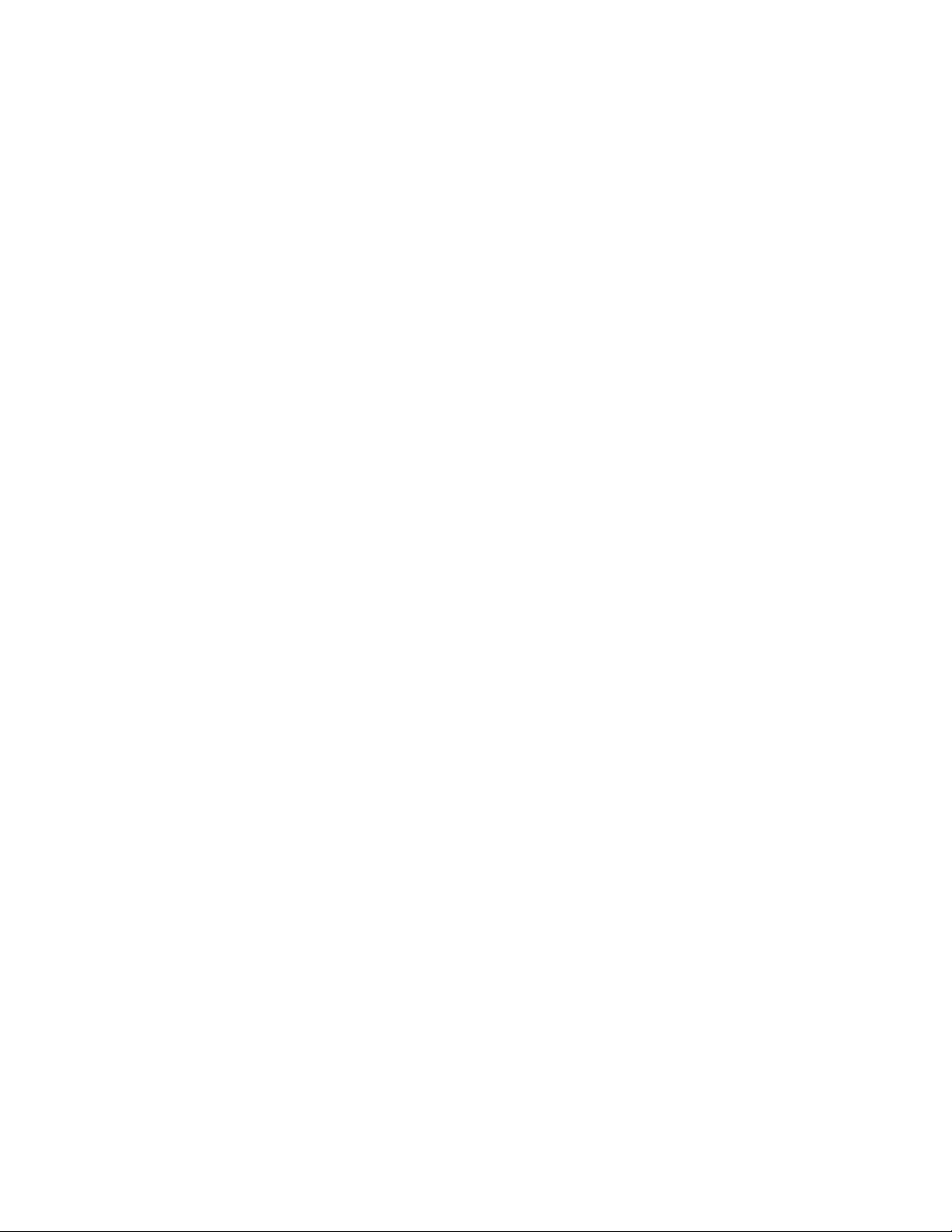
Multiple Linear and 1D Regression
David J. Olive
Southern Illinois University
Department of Mathematics
Mailcode 4408
Carbondale, IL 62901-4408
dolive@math.siu.edu
January 4, 2010
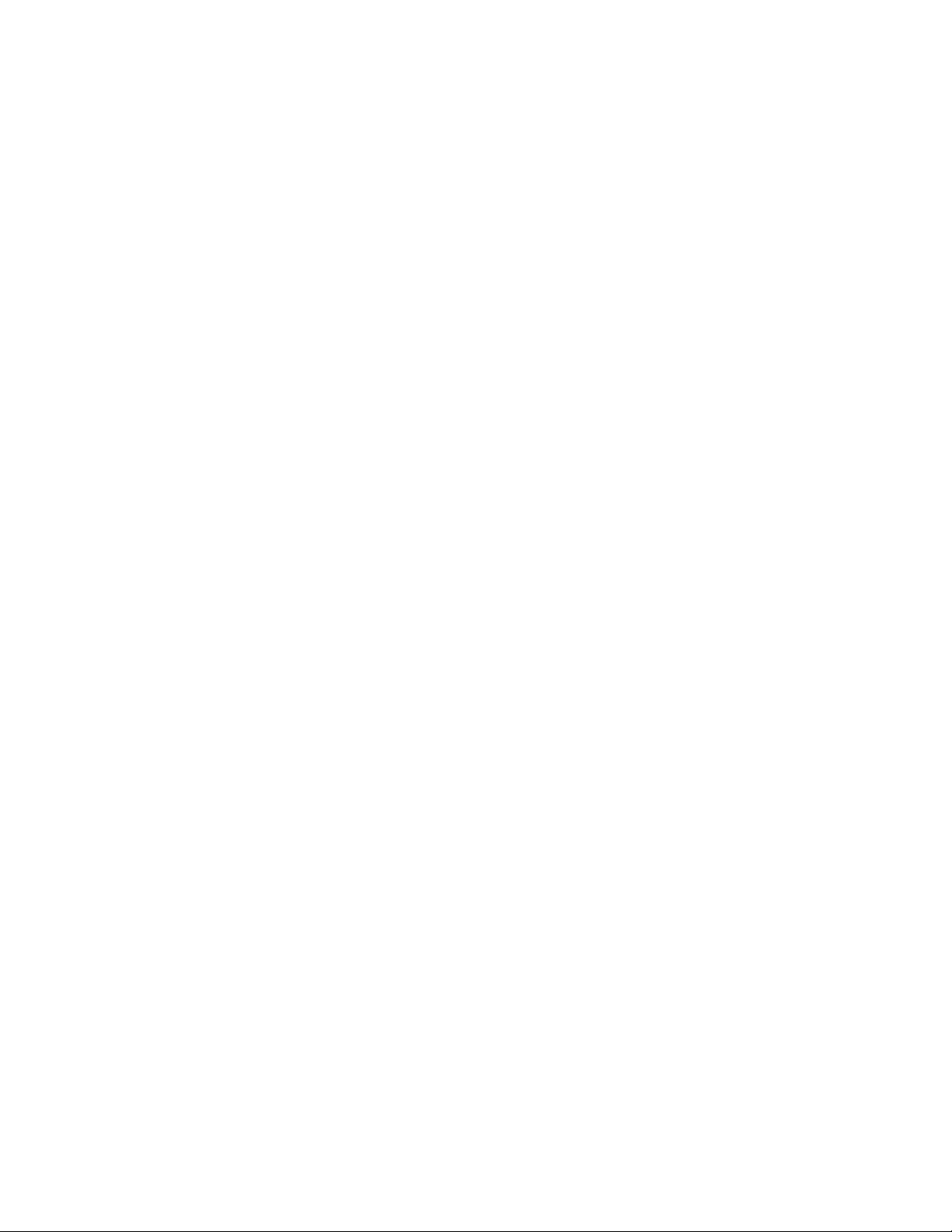
Contents
Preface vi
1 Introduction 1
1.1 Multiple Linear Regression ................. 5
1.2 Logistic Regression ...................... 9
1.3 Poisson Regression ....................... 12
1.4 Single Index Models ...................... 16
1.5 Survival Regression Models ................. 19
1.6 Variable Selection ....................... 20
1.7 Other Issues ........................... 25
1.8 Complements .......................... 27
1.9 Problems ............................. 27
2 Multiple Linear Regression 28
2.1 The MLR Model ........................ 28
2.2 Checking Goodness of Fit .................. 31
2.3 Checking Lack of Fit ..................... 35
2.3.1 Residual Plots ..................... 36
2.3.2 Other Model Violations ............... 40
2.4 The ANOVA F TEST ..................... 42
2.5 Prediction ............................ 49
2.6 The Partial F or Change in SS TEST ........... 56
2.7 The Wald t Test ........................ 61
2.8 The OLS Criterion ....................... 64
2.9 Two Important Special Cases ................ 68
2.9.1 The Location Model .................. 68
2.9.2 Simple Linear Regression .............. 69
2.10 The No Intercept MLR Model ............... 71
i
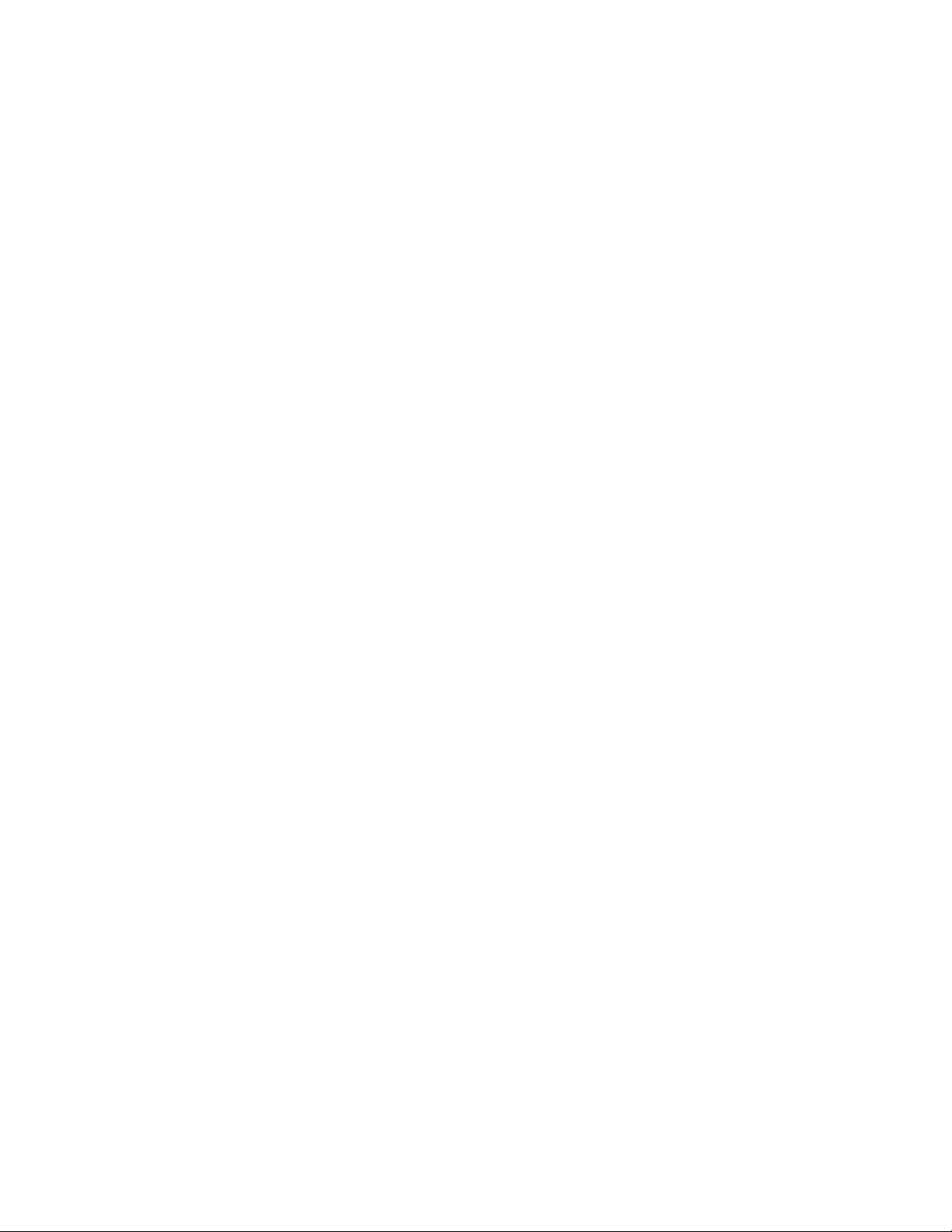
2.11 Summary ............................. 74
2.12 Complements .......................... 77
2.12.1 Lack of Fit Tests .................... 79
2.13 Problems ............................. 81
3 Building an MLR Model 102
3.1 Predictor Transformations ..................103
3.2 Graphical Methods for Response Transformations . . . 109
3.3 Main Effects, Interactions and Indicators ........116
3.4 Variable Selection .......................118
3.5 Diagnostics ...........................141
3.6 Outlier Detection .......................146
3.7 Summary .............................151
3.8 Complements ..........................155
3.9 Problems .............................160
4 WLS and Generalized Least Squares 181
4.1 Random Vectors ........................181
4.2 GLS, WLS and FGLS .....................183
4.3 Inference for GLS .......................188
4.4 Complements ..........................191
4.5 Problems .............................191
5 One Way ANOVA 194
5.1 Introduction ...........................194
5.2 Fixed Effects One Way ANOVA ..............196
5.3 Random Effects One Way ANOVA ............207
5.4 Response Transformations for Experimental Design . . 209
5.5 Summary .............................211
5.6 Complements ..........................216
5.7 Problems .............................222
6 K Way ANOVA 234
6.1 Two Way ANOVA .......................234
6.2 kWayAnovaModels .....................240
6.3 Summary .............................240
6.4 Complements ..........................243
6.5 Problems .............................243
ii
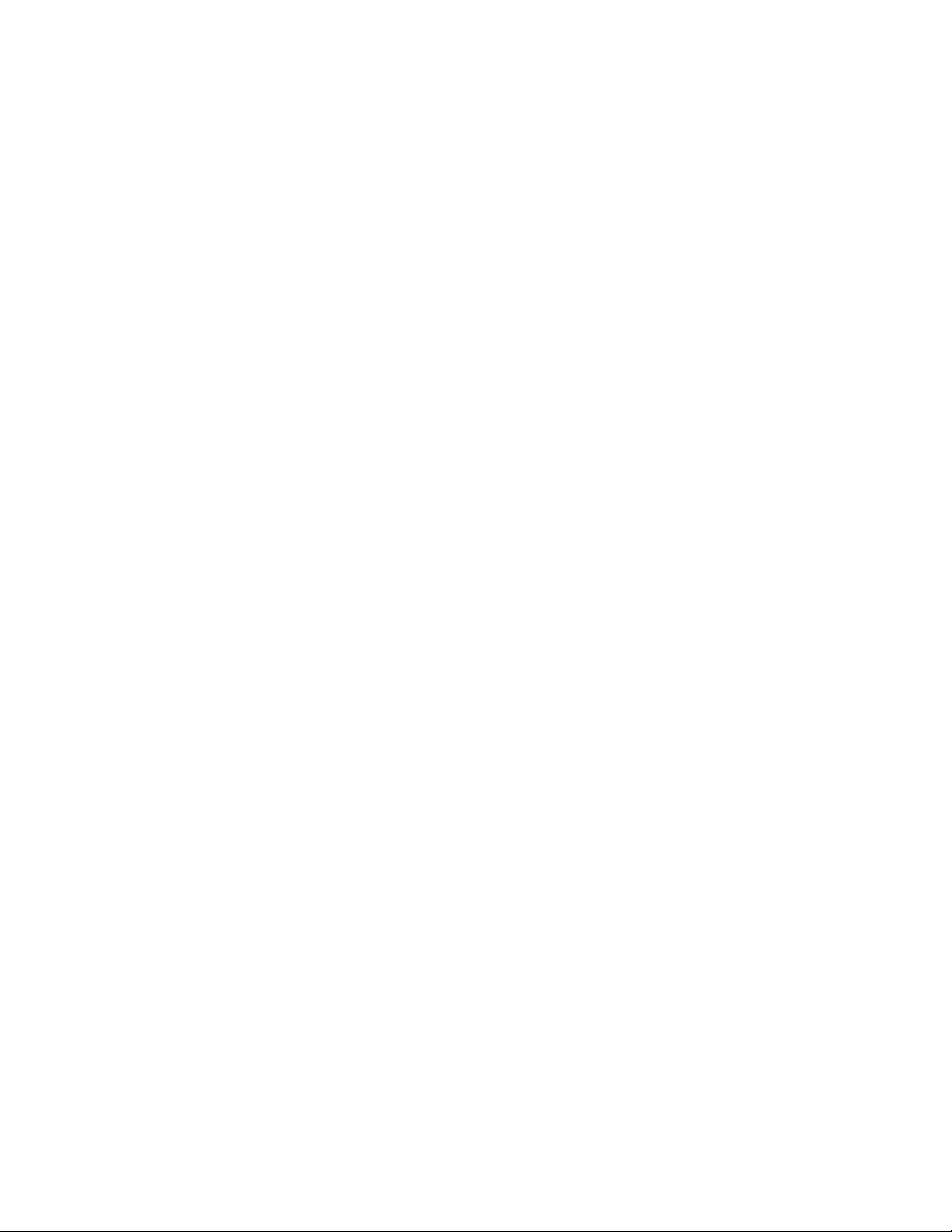
7 Block Designs 248
7.1 One Way Block Designs ...................249
7.2 Blocking with the K Way Anova Design .........253
7.3 Latin Square Designs .....................255
7.4 Summary .............................259
7.5 Complements ..........................262
7.6 Problems .............................263
8 Orthogonal Designs 267
8.1 Factorial Designs ........................267
8.2 Fractional Factorial Designs .................283
8.3 Plackett Burman Designs ..................288
8.4 Summary .............................291
8.5 Complements ..........................303
8.6 Problems .............................304
9 More on Experimental Designs 311
9.1 Split Plot Designs .......................311
9.1.1 Whole Plots Randomly Assigned to A ......312
9.1.2 Whole Plots Assigned to A as in a CRBD ....314
9.2 Review of the DOE Models .................317
9.3 Summary .............................320
9.4 Complements ..........................324
9.5 Problems .............................324
10 Logistic Regression 329
10.1 Binary Regression .......................329
10.2 Binomial Regression ......................335
10.3 Inference .............................340
10.4 Variable Selection .......................350
10.5 Complements ..........................358
10.6 Problems .............................361
11 Poisson Regression 375
11.1 Poisson Regression .......................375
11.2 Inference .............................383
11.3 Variable Selection .......................388
11.4 Complements ..........................393
iii
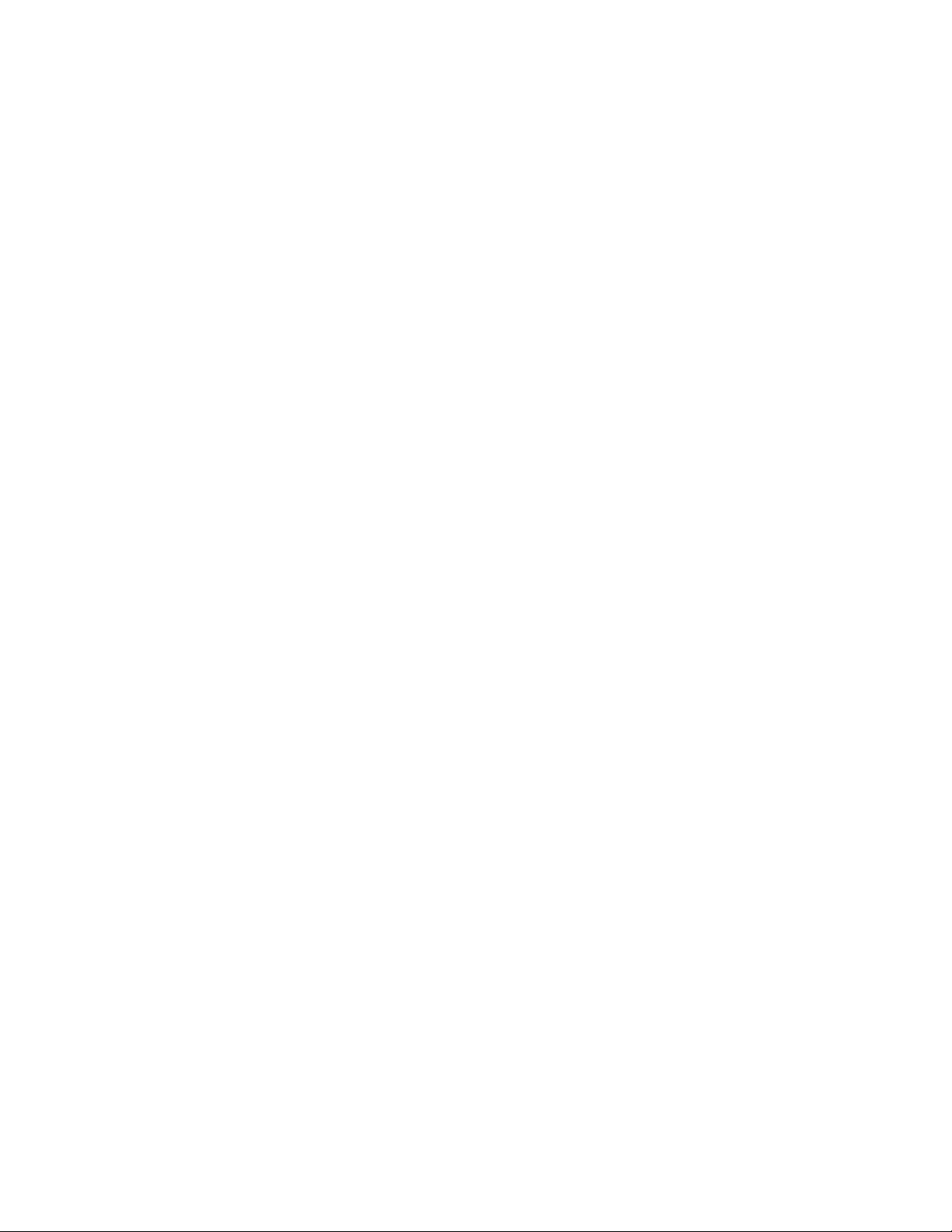
11.5 Problems .............................395
12 Generalized Linear Models 401
12.1 Introduction ...........................401
12.2 Multiple Linear Regression .................403
12.3 Logistic Regression ......................404
12.4 Poisson Regression .......................406
12.5 Inference and Variable Selection ..............407
12.6 Complements ..........................414
12.7 Problems .............................415
13 Theory for Linear Models 416
13.1 Complements ..........................416
13.2 Problems .............................417
14 Multivariate Models 419
14.1 The Multivariate Normal Distribution ..........420
14.2 Elliptically Contoured Distributions ............424
14.3 Sample Mahalanobis Distances ...............428
14.4 Complements ..........................429
14.5 Problems .............................429
15 1D Regression 433
15.1 Estimating the Sufficient Predictor ............436
15.2 Visualizing 1D Regression ..................441
15.3 Predictor Transformations ..................449
15.4 Variable Selection .......................450
15.5 Inference .............................461
15.6 Complements ..........................472
15.7 Problems .............................475
16 Survival Analysis 481
16.1 Univariate Survival Analysis .................482
16.2 Proportional Hazards Regression ..............495
16.2.1 Visualizing the Cox PH Regression Model ....496
16.2.2 Testing and Variable Selection ...........502
16.3 Weibull and Exponential Regression ............509
16.4 Accelerated Failure Time Models .............516
iv
剩余640页未读,继续阅读
资源评论
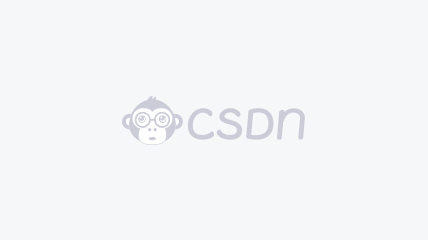

Alladins
- 粉丝: 1
- 资源: 57
上传资源 快速赚钱
我的内容管理 展开
我的资源 快来上传第一个资源
我的收益
登录查看自己的收益我的积分 登录查看自己的积分
我的C币 登录后查看C币余额
我的收藏
我的下载
下载帮助

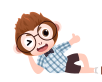
最新资源
- whl@pip install pyaudio ERROR: Failed building wheel for pyaudio
- Constantsfd密钥和权限集合.kt
- 基于Java的财务报销管理系统后端开发源码
- 基于Python核心技术的cola项目设计源码介绍
- 基于Python及多语言集成的TSDT软件过程改进设计源码
- 基于Java语言的歌唱比赛评分系统设计源码
- 基于JavaEE技术的课程项目答辩源码设计——杨晔萌、李知林、岳圣杰、张俊范小组作品
- 基于Java原生安卓开发的蔚蓝档案娱乐应用设计源码
- 基于Java、Vue、JavaScript、CSS、HTML的毕设设计源码
- 基于Java和HTML的CMS看点咨询系统设计源码
资源上传下载、课程学习等过程中有任何疑问或建议,欢迎提出宝贵意见哦~我们会及时处理!
点击此处反馈


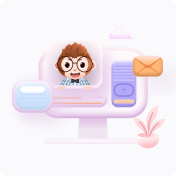
安全验证
文档复制为VIP权益,开通VIP直接复制
