%% gru network
% Programmed by qq,2027771338
% Updated 20 jun. 2024.
clc
clear
close all
%读取double格式数据
[a,ax,ay] = xlsread('数据.xlsx',1);
% t=[];
% for ii =1:4
% y(ii)=myPearson(a(:,ii),a(:,5));
% end
% plot(y)
for ii =1:2
a(:,5) =smooth(a(:,5),5);
end
num = [];
for ii = 1:length(a)-1
num = [num;a(ii,5) a(ii+1,:)];
end
m1=569;
n = randperm(m1);
m=500;
input_train=num(n(1:569),1:5)';%训练数据的输入数据
output_train=num(n(1:569),6)';%训练数据的输出数据
input_test=num((m+1:end),1:5)';%测试数据的输入数据
output_test=num((m+1:end),6)'; %测试数据的输出数据
[inputn,inputps]=mapminmax(input_train,-20,100);%训练数据的输入数据的归一化
[outputn,outputps]=mapminmax(output_train,-20,100);%训练数据的输出数据的归一化de
inputn_test=mapminmax('apply',input_test,inputps);
%% Define Network Architecture
% Define the network architecture.
numFeatures = size(num(:,1:5),2);%输入层维度
numResponses = size(num(:,end),2);%输出维度
% 200 hidden units
numHiddenUnits = 150;%第一层维度
% a fully connected layer of size 50 & a dropout layer with dropout probability 0.5
fungru
%
% Specify the training options.
% Train for 60 epochs with mini-batches of size 20 using the solver 'adam'
maxEpochs = 100;%最大迭代次数
miniBatchSize = 2;%最小批量
% the learning rate == 0.01
% set the gradient threshold to 1
% set 'Shuffle' to 'never'
options = trainingOptions('adam', ... %解算器
'MaxEpochs',maxEpochs, ... %最大迭代次数
'MiniBatchSize',miniBatchSize, ... %最小批次
'InitialLearnRate',0.01, ... %初始学习率
'GradientThreshold',inf, ... %梯度阈值
'Shuffle','every-epoch', ... %打乱顺序
'Plots','training-progress',... %画图
'Verbose',0); %不输出训练过程
%% Train the Network
net = trainNetwork(inputn,outputn,layers,options);%开始训练
%% Test the Network
y_pred = predict(net,inputn_test,'MiniBatchSize',100)';%测试仿真输出
y_pred =(mapminmax('reverse',y_pred,outputps))';
% load maydatalstm.mat
% save maydatalstm.mat net y_pred outputps inputps
%%
figure%打开一个图像窗口
plot(y_pred,'r-')%蓝色实线,点的形状^
hold on%停止画图
plot(output_test,'k-')%黑色实线,点的形状为*
hold off%继续画图
title('测试图')%标题
ylabel('')%Y轴名称
legend('测试值','实际值')%标签
error1 = y_pred-output_test;%误差
figure
plot(error1,'k-')
title('测试误差图')
ylabel('误差')
[MSE,RMSE,MBE,MAE ] =MSE_RMSE_MBE_MAE(output_test,y_pred)
R2 = R_2(output_test,y_pred)
numz = [num((m+1:end),:) y_pred'];
result_table = table;
result_table.Mpa0 = num(m+1:end,1);
result_table.ps = num(m+1:end,2);
result_table.A = num(m+1:end,3);
result_table.AB = num(m+1:end,4);
result_table.K = num(m+1:end,5);
result_table.true = output_test';
result_table.sim = y_pred';
writetable(result_table,'./结果.csv')
没有合适的资源?快使用搜索试试~ 我知道了~
GRU神经网络应力预测.zip
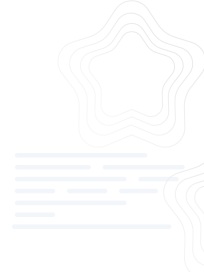
共11个文件
m:5个
xlsx:2个
jpg:2个

1.该资源内容由用户上传,如若侵权请联系客服进行举报
2.虚拟产品一经售出概不退款(资源遇到问题,请及时私信上传者)
2.虚拟产品一经售出概不退款(资源遇到问题,请及时私信上传者)
版权申诉
0 下载量 140 浏览量
2024-07-05
09:07:55
上传
评论 1
收藏 1.09MB ZIP 举报
温馨提示
基于MATLAB编程,用GRU神经网络应力预测,应力预测是一种时间序列的数据,用gru比一般神经网络更适合,代码完整,包含数据,有注释,方便扩展应用 1,如有疑问,不会运行,可以私信, 2,需要创新,或者修改可以扫描二维码联系博主, 3,本科及本科以上可以下载应用或者扩展, 4,内容不完全匹配要求或需求,可以联系博主扩展。
资源推荐
资源详情
资源评论
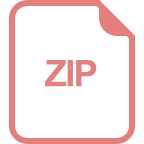
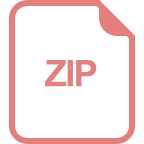
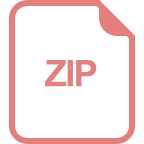
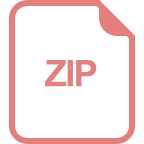
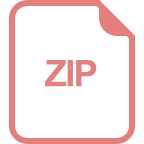
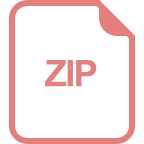
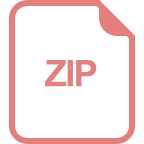
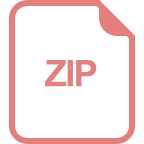
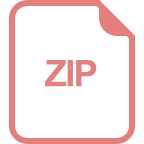
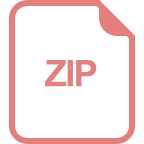
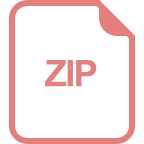
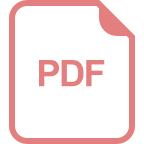
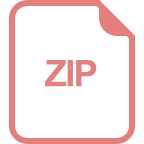
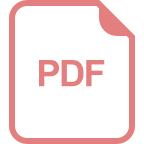
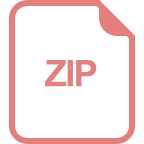
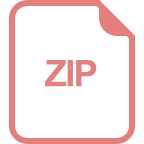
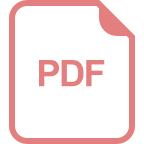
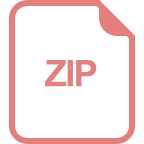
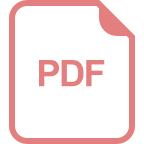
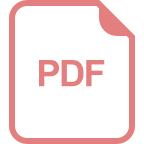
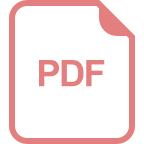
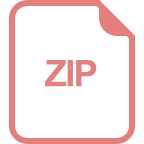
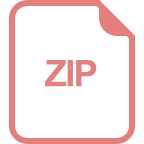
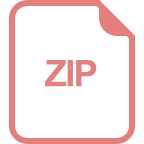
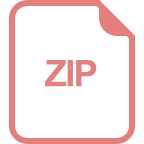
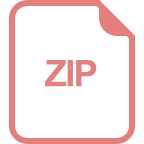
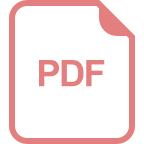
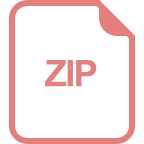
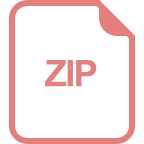
收起资源包目录

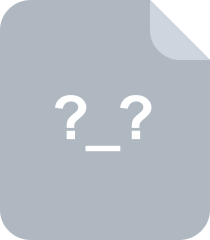
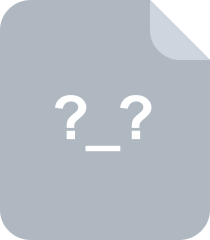
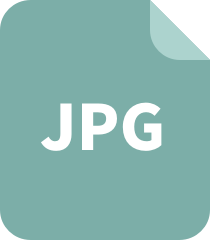
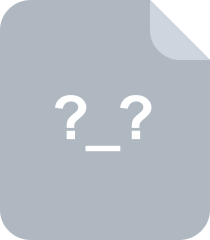
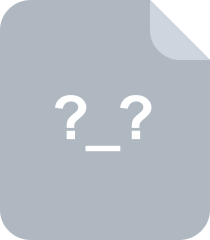
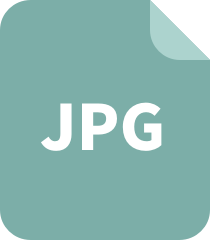
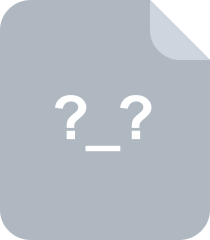
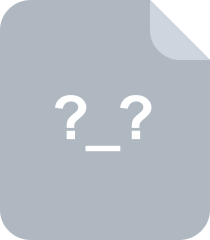
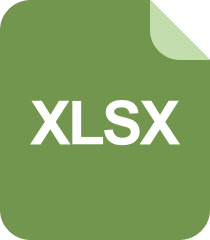
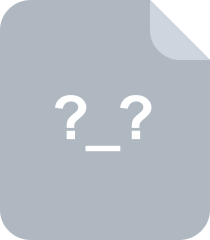
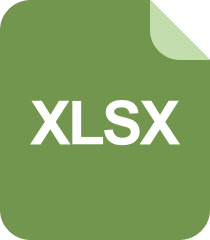
共 11 条
- 1
资源评论
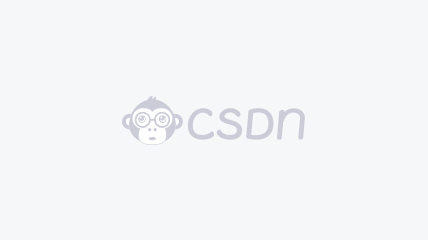


神经网络机器学习智能算法画图绘图
- 粉丝: 2797
- 资源: 659

下载权益

C知道特权

VIP文章

课程特权

开通VIP
上传资源 快速赚钱
我的内容管理 展开
我的资源 快来上传第一个资源
我的收益
登录查看自己的收益我的积分 登录查看自己的积分
我的C币 登录后查看C币余额
我的收藏
我的下载
下载帮助

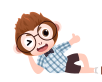
最新资源
- 提取文字_20241116.docx
- 使用 PyTorch /TensorFlow实现 ZFNet 进行 MNIST 图像分类
- Python课程设计之俄罗斯方块
- C#仓库条码管理系统源码数据库 SQL2008源码类型 WinForm
- 网安面试题合集-来自网络收集.zip
- (2024最新整理)42万+世界各国新冠疫情数据(2020.1-2024.8)
- ESP32开发板(CH340驱动芯片) Type-C口+数据线+0.96显示屏+杜邦线 链接wifi,显示当前时间
- windows hex查看工具
- C语言编程基础及其应用详解:从入门到实战练习.pdf
- C#CS小型医院HIS门诊挂号收费管理系统源码数据库 SQL2008源码类型 WinForm
资源上传下载、课程学习等过程中有任何疑问或建议,欢迎提出宝贵意见哦~我们会及时处理!
点击此处反馈


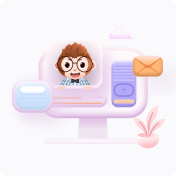
安全验证
文档复制为VIP权益,开通VIP直接复制
