% SB1_ESTIMATE Estimate parameters in a sparse Bayesian model
%
% [W,USED,ML,A,B,G] = SB1_ESTIMATE(PHI,T,A,B,MAXITS,MONITS)
%
% OUTPUT ARGUMENTS:
%
% W Estimated weights (subset of full model)
% USED Indices of relevant basis vectors
% ML Marginal likelihood of final model
% A Estimated 'alpha' values
% B Estimated 'beta' value for regression
% G Estimated 'gamma' (well-determinedness) parameters
%
% INPUT ARGUMENTS:
%
% PHI Basis function/design matrix
% T Target vector
% A Initial alpha value (scalar)
% B Initial beta value
% - set to zero for classification
% - set negative to fix in regression,
% MAXITS Maximum number of iterations to run for
% MONITS Monitor estimation progress every MONITS iterations
% [optional, defaults to zero]
%
%
% NOTES: Arguments should be self-explanatory, although in regression
% a negative value for BETA is used to indicate that it should
% remain fixed (to the positive value) during estimation.
%
% Copyright 2009 :: Michael E. Tipping
%
% This file is part of the SPARSEBAYES baseline implementation (V1.10)
%
% Contact the author: m a i l [at] m i k e t i p p i n g . c o m
%
function [weights, used, marginal, alpha, beta, gamma] = ...
SB1_Estimate(PHI,t,alpha,beta,maxIts,monIts)
% Terminate estimation when no log-alpha value changes by more than this
MIN_DELTA_LOGALPHA = 1e-3;
% Prune basis function when its alpha is greater than this
% - reduce this to be more agressive
%
ALPHA_MAX = 1e9;
% Default to no monitoring
if ~exist('monIts','var')
monIts = 0;
end
REGRESSION = (beta~=0);
if REGRESSION
% Regression settings
%
% - negative beta is used to indicate that the noise model
% is to remain fixed
%
FIXED_BETA = beta<0;
beta = abs(beta);
else
% Classification settings
%
maxIts_pm = 25; % Maximum iterations for posterior mode-finder
end
% Set up parameters and hyperparameters
[N,M] = size(PHI);
w = zeros(M,1);
alpha = alpha*ones(M,1);
gamma = ones(M,1);
% Useful shortcut
PHIt = PHI'*t;
%
LAST_IT = 0;
%
% The main loop
%
for i=1:maxIts
%
% Prune based on large values of alpha
%
useful = (alpha<ALPHA_MAX);
alpha_used = alpha(useful);
M = sum(useful);
%
% Prune weights and basis
%
w(~useful) = 0;
PHI_used = PHI(:,useful);
%
% Compute key stats:
%
% - most probable weights
% - inverse Cholesky factor
% - data error
%
if REGRESSION
%
% Calculation is analytic for Gaussian likelihood here
%
Hessian = (PHI_used'*PHI_used)*beta + diag(alpha_used);
try chol(Hessian);
Hessian = Hessian;
catch ME;
X = diag(rand(size(Hessian,1),1));
U = orth(rand(size(Hessian,1),size(Hessian,1)));
Hessian = U' * X * U;
end
U = chol(Hessian);
Ui = inv(U);
w(useful) = (Ui * (Ui' * PHIt(useful)))*beta;
%
ED = sum((t-PHI_used*w(useful)).^2); % Data error
dataLikely = (N*log(beta) - beta*ED)/2;
else
%
% Must call posterior mode finder for Bernoulli likelihood
%
[w(useful) Ui dataLikely] = ...
SB1_PosteriorMode(PHI_used,t,w(useful),alpha_used,maxIts_pm);
end
%
% Need determinant and diagonal values of
% posterior weight covariance matrix (SIGMA in paper)
%
logdetH = -2*sum(log(diag(Ui)));
diagSig = sum(Ui.^2,2);
%
% Well-determinedness parameters (gamma)
%
gamma = 1 - alpha_used.*diagSig;
%
% Compute marginal likelihood (approximation for classification case)
%
marginal = dataLikely - 0.5*(logdetH - sum(log(alpha_used)) + ...
(w(useful).^2)'*alpha_used);
%
% Output diagnostic info when requested and at end
%
if (LAST_IT || (monIts && ~rem(i,monIts)))
if REGRESSION
SB1_Diagnostic(1,'%5d> L = %.3f\t Gamma = %.2f (nz = %d)\t s=%.3f\n',...
i, marginal, sum(gamma), sum(useful), sqrt(1/beta));
else
SB1_Diagnostic(1,'%5d> L = %.3f\t Gamma = %.2f (nz = %d)\n',...
i, marginal, sum(gamma), sum(useful));
end
end
if ~LAST_IT
%
% alpha and beta re-estimation on all but last iteration
% (only update the posterior statistics the last time around)
%
logAlpha = log(alpha(useful));
%
% Alpha re-estimation
%
% This will be much improved in the subsequent SB2 library
%
% MacKay-style update for alpha given in original NIPS paper
%
alpha(useful) = gamma ./ w(useful).^2;
%
% Terminate if the largest alpha change is smaller than threshold
%
au = alpha(useful);
maxDAlpha = max(abs(logAlpha(au~=0)-log(au(au~=0))));
if maxDAlpha<MIN_DELTA_LOGALPHA
LAST_IT = 1;
SB1_Diagnostic(1,...
'Terminating: max log(alpha) change is %g (<%g).\n', ...
maxDAlpha, MIN_DELTA_LOGALPHA);
end
%
% Beta re-estimate in regression (unless fixed)
%
if REGRESSION && ~FIXED_BETA
beta = (N - sum(gamma))/ED;
end
else
% Its the last iteration due to termination, leave outer loop
break; % that's all folks!
end
end
%
% Tidy up return values
%
weights = w(useful);
used = find(useful);
%
if ~LAST_IT
SB1_Diagnostic(1,'Terminating due to max iterations (did not converge)\n');
end
SB1_Diagnostic(1,'Hyperparameter estimation complete\n');
SB1_Diagnostic(2,'non-zero parameters:\t%d\n', length(weights));
SB1_Diagnostic(2,'log10-alpha min/max:\t%.2f/%.2f\n', ...
log10([min(alpha_used) max(alpha_used)]));


海神之光
- 粉丝: 5w+
- 资源: 6110
最新资源
- 项目采用YOLO V4算法模型进行目标检测,使用Deep SORT目标跟踪算法 .zip
- 针对实时视频流和静态图像实现的对象检测和跟踪算法 .zip
- 部署 yolox 算法使用 deepstream.zip
- 基于webmagic、springboot和mybatis的MagicToe Java爬虫设计源码
- 通过实时流协议 (RTSP) 使用 Yolo、OpenCV 和 Python 进行深度学习的对象检测.zip
- 基于Python和HTML的tb商品列表查询分析设计源码
- 基于国民技术RT-THREAD的MULTInstrument多功能电子测量仪器设计源码
- 基于Java技术的网络报修平台后端设计源码
- 基于Python的美食杰中华菜系数据挖掘与分析设计源码
- 基于Java与JavaScript混合技术的吉森摄影项目设计源码
资源上传下载、课程学习等过程中有任何疑问或建议,欢迎提出宝贵意见哦~我们会及时处理!
点击此处反馈


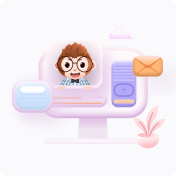