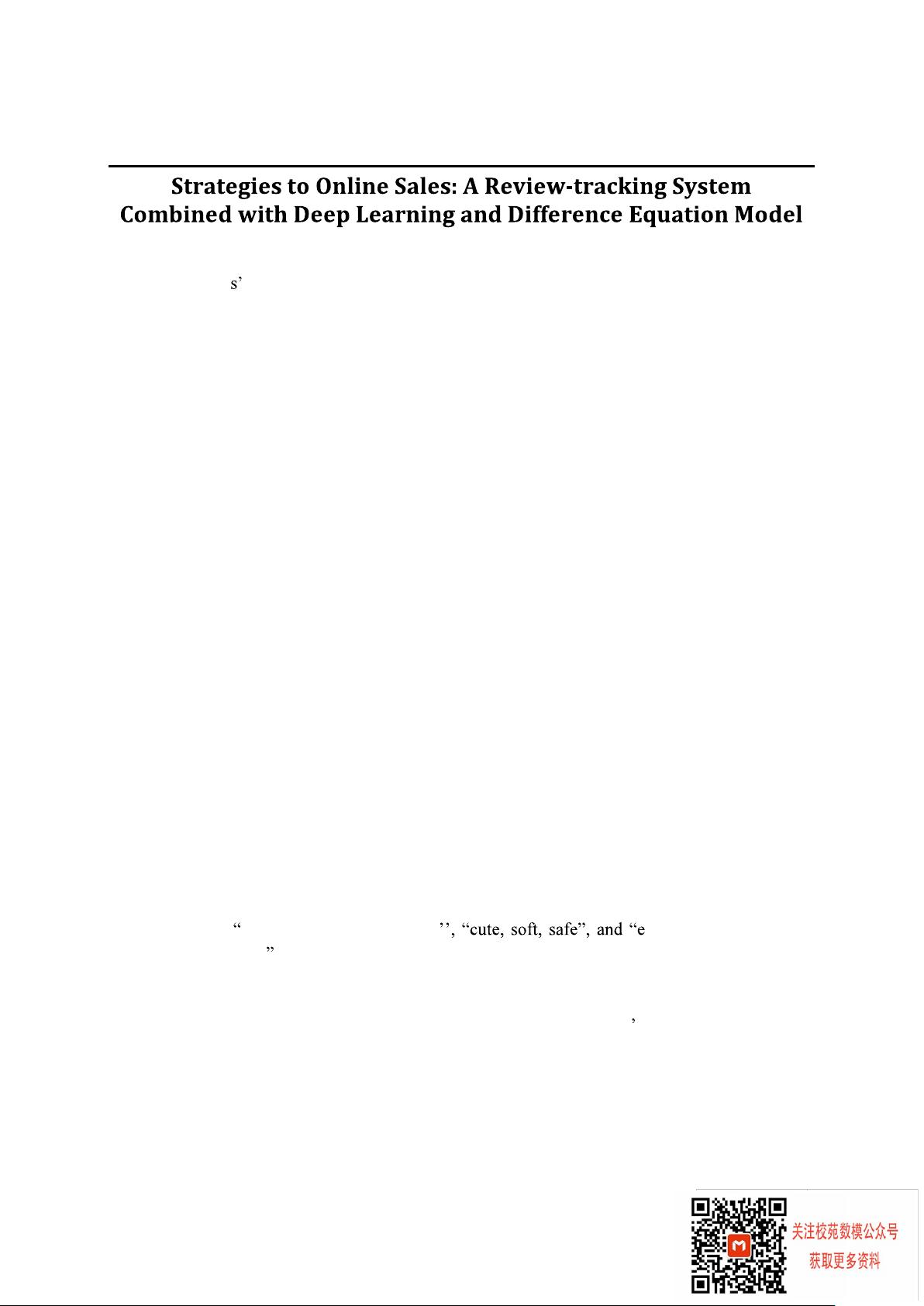
Problem Chosen
C
2020
MCM/ICM
Summary Sheet
Team Control Number
2007707
With the booming online shopping platforms, online reviews play an increasingly significant
role in customer
purchase decisions. How to identify the time-based patterns of reviews and
future reputation of newly launched products is a major concern for companies.
In order to address this problem, we make statistics on review indicators of the hair dryers,
pacifiers, and microwaves. With a preliminary understanding of data, we set five evaluation
indicators based on star-ratings and text-based reviews, namely the average star-rating, favorable
rate, number of reviews, average number of review words, and average review sentiment value.
We then build a text sentiment analysis model based on deep learning, extract keywords in the
reviews by Term Frequency-Inverse Document Frequency (TF-IDF) algorithm, and calculate
the sentiment value of reviews by the back propagation (BP) neural network. In this way, we
explore the specific relationship between review sentiment and star-rating levels and propose
design focus for the three products. Later, we build a difference equation model to identify the
changing patterns of each evaluation indicator as time and other indicators change based on the
correlation test results of the five evaluation indicators. Finally, we construct the success index
by principal component analysis (PCA) based on the evaluation indicators, which provides the
company with an easy and convenient system to track potential successes or failures of a product.
We find some insightful conclusions based on our results as follows. (1) Star-ratings,
favorable rate, number of review words, and review sentiment value all decline over time. For
hair dryers, high review sentiment value will reduce the increase in star-ratings in the next
quarter. Similarly, high star-ratings and favorable rate will also lower the review sentiment value
in the next quarter. (2) Reviews with low star-ratings cause an increase in negative sentiment,
while those with high star-ratings have no significant effect. Also, favorable reviews are strongly
associated with high star-ratings, but negative ones are not significantly related to them. (3) The
emotional words rank the first in the importance of reviews, followed by descriptive adjectives
and characteristics words of products. (4) The success index effectively reflects the operating
conditions and future reputations, which is a valid evaluation indicator.
Based on the above analysis, we provide some strategies on product sales and design for
Sunshine Company, which includes (1) the design of hair dryers, pacifiers and microwaves
should focus on
high-power, light, portable asily installed, suitable
size, timely repaired
, respectively; (2) increase the yield to reduce concentrated negative
reviews instead of pursuing favorable reviews blindly; and (3) ensure that the future product
reputation is above the average by employing the success index for timely tracking.
In the end, we make sensitivity analysis, and verify the model
s robustness and result
adaptability. In a nutshell, our model is accurate in sentiment analysis, consistent with the
reality, as well as simple, effective and practical in tracking future operating conditions.
Key words: Online Sales Strategies, Text Sentiment Analysis, Deep Learning, Difference
Model, Principal Component Analysis