没有合适的资源?快使用搜索试试~ 我知道了~
温馨提示
资源说明 【1】资源属于对应项目写的论文,写作规范、逻辑紧密、用语专业严谨,内容丰富饱满,可读性强,很适合对该领域的初学者、工程师、在校师生、毕业生等下载使用。 【2】论文适合学习借鉴参考,为您的类似项目开发或写作提供专业知识介绍及思路。 【3】资源非项目源码,如需项目源码,请私信沟通,不Free。 【4】可用于毕业设计、课程设计,切记完全照抄! 【5】鼓励大家下载后仔细研读,多看、多思考!搞懂里面的知识点及实验内容。欢迎交流学习!
资源推荐
资源详情
资源评论
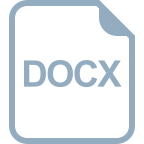
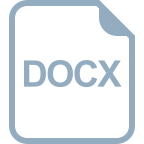
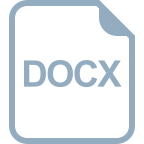
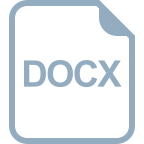
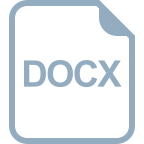
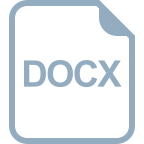
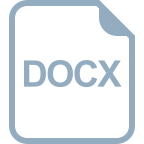
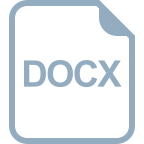
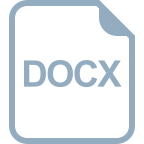
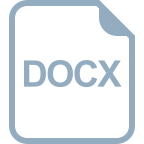
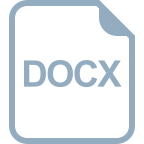
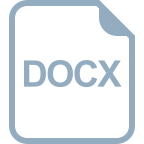
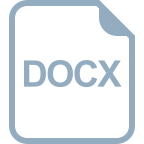
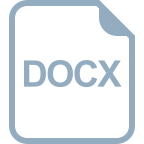
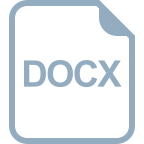
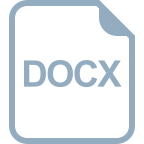
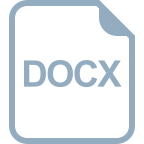
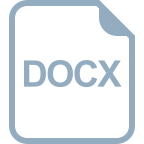
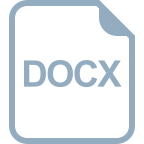
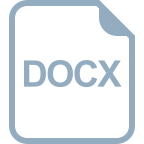
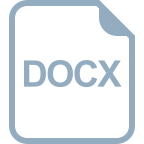
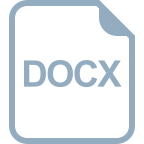
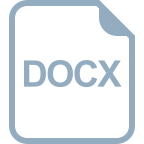
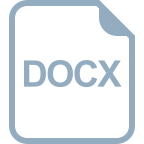
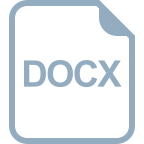
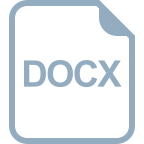
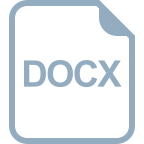
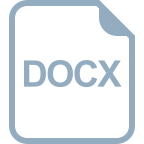
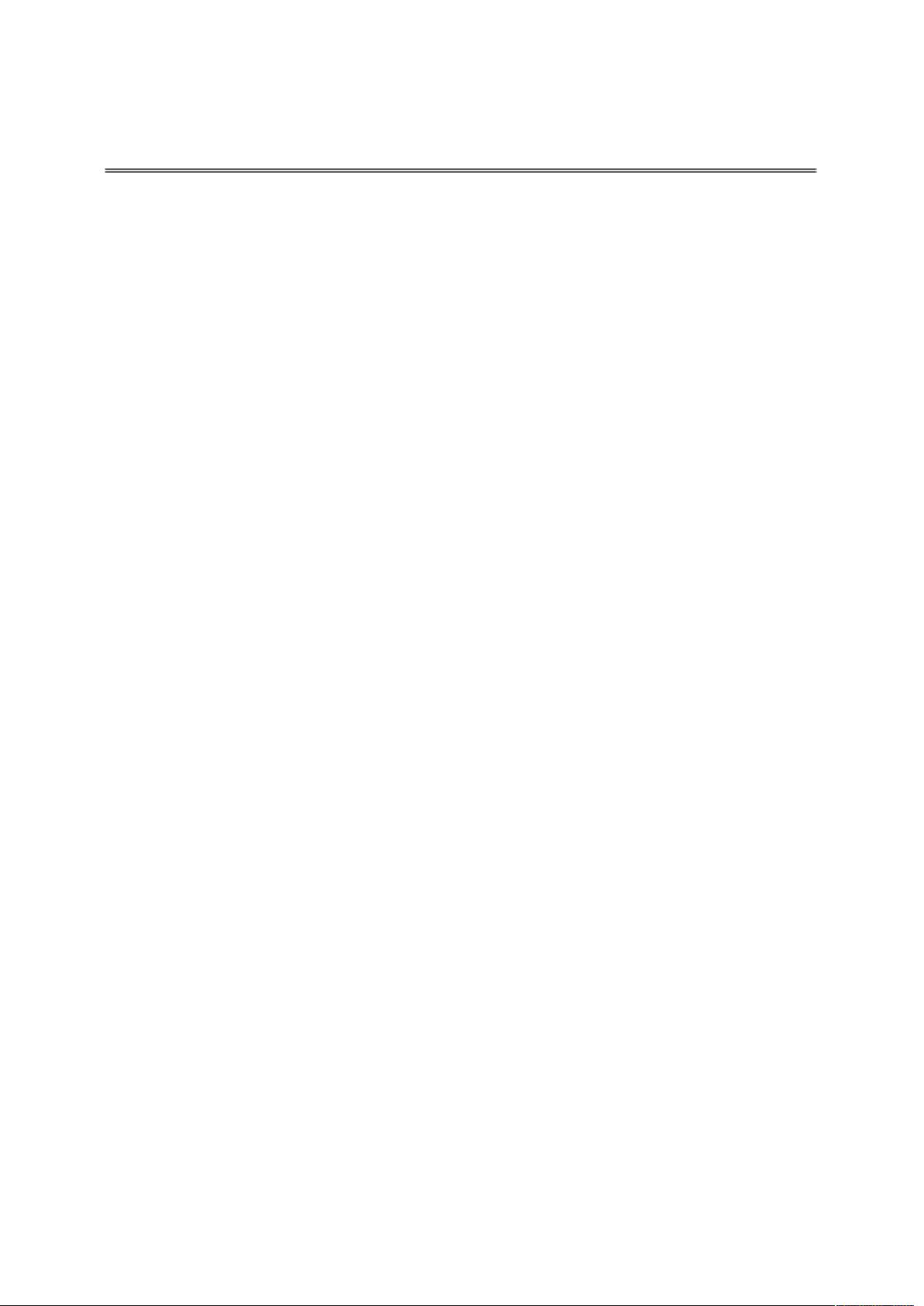
基于深度学习的行李取放行为
算法研究
摘 要
随着深度学习技术的发展,如何应用它改善人们的生活的正在成为研究热点。在
实际列车车厢中,人流量较大,因此很容易出现错拿行李、盗拿行李的情况,仅通过
人力来判断行李取放十分困难,因此,针对该场景研究动作识别算法,以行李取放行
为为切入点,进行动作识别分析具有重要的研究意义和实际价值。
在模拟车厢场景搜集并标注的行李取放的图片的基础上,设计并在这些数据上
训练了一系列高精度且兼顾实时性的模型。具体而言,针对基于关键点检测的人物交
互检测算法 PPDM,其交互中心点设置的位置不适用取放行为的检测的情形,修改了
交互中心点预测位置,并借鉴其他融合姿态信息的网络提升关键点检测能力。同时,
在进一步提升人物交互检测算法对行李取放识别性能的基础上,分离出交互检测算
法实现动作识别的核心模块目标检测分支和姿态分支,分别探究边界框标注、数据增
强对目标检测表现的影响,以及基于姿态估计网络的关键点位置,手工特征设计与机
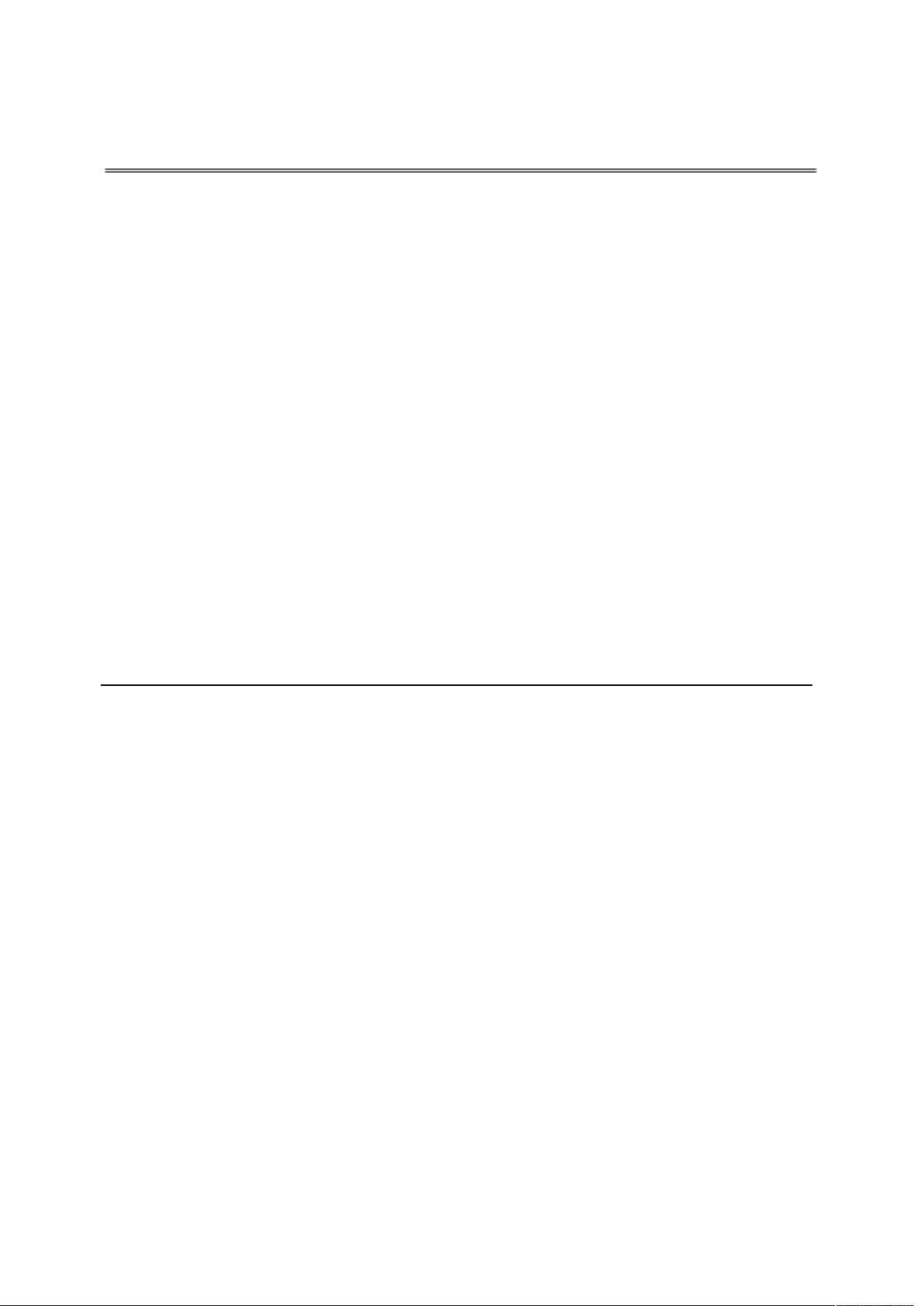
器特征学习对动作检测的影响,并在这两者基础上,探究模型融合策略对最终动作检
测效果的提升。
实际测试表明,针对取放行为专门优化的交互点检测方案与添加的姿态分支,能
在原方法基准上提升分类的精确率与召回率;经过数据增强的目标检测与基于多层
感知机处理姿态信息的检测方法的精确率与召回率均超过了 90%,在此基础上,对
目标检测和姿态估计的输出进行模型融合以损失一定实时性的代价对行李取放取得
了最好的识别效果。
关键词: 深度学习,取放行为,动作识别,模型融合
I
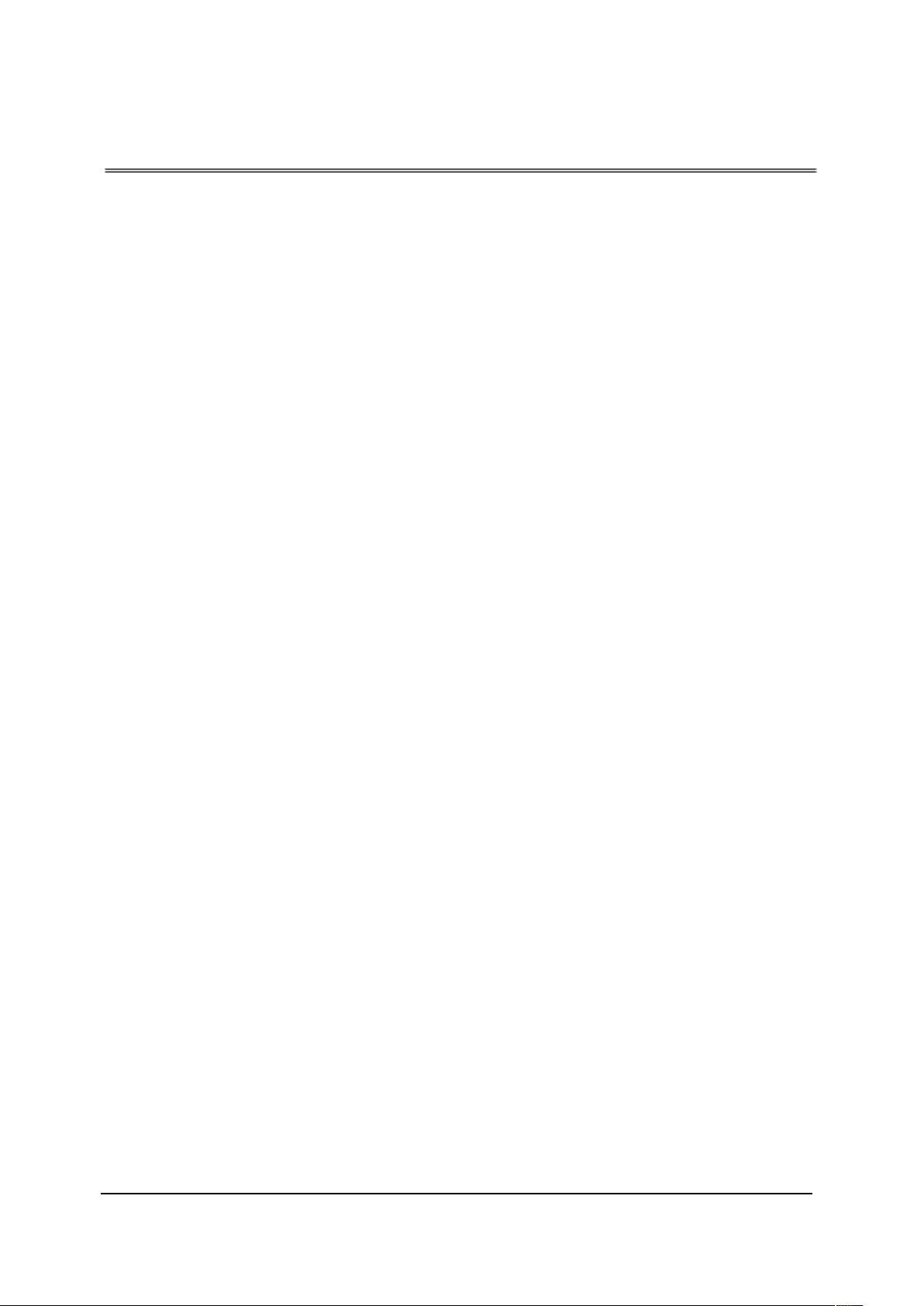
Abstract
With the development of deep learning, how to apply it to improve people's lifestyle is
becoming a research focus. In real carriages, it is usually crowded, which makes occasions
like passenger’s luggage being taken mistakenly or stolen happen frequently,
besides,
catching each
behavior is
difficult relying on
human
resources
only, therefore,
taking the
behavior of picking up
or
taking away luggages
as the reaserch perspective,
it is
of great
significance and practical value to study the action recognition algorithm for travel scenarios.
This paper
collects and tags datas which simulate the real carriages, and designs and
trains a
series of high accuracy and real-time
models on
these
datas. Specifically, for
the
human-object interaction detection algorithm PPDM, its pre-defined interaction point is not
suitable for detecting luggage’s being picked up and taken away behavior, thus in this paper,
the interaction point’s location is modified to suit the task, besides, a pose branch is added
to
enhance the new network’s detection ability of the interaction point
inspired by
other
methods. Furthermore, to
improve
the
performance
of human-object
interaction
detection
on
luggage’s being picked up and taken away,
this paper extracts the core ways that
dominate
the
performance in
human-object
interaction,
that is, object detection and pose
estimation, and explores the factors that influence the performance such as tagging choices,
data augmentation in object detection and manual
feature design, machine
feature learning
in pose estimation
separately. Finally,
how
model fusion
influences
the final performance
will be explored in this paper.
The experimental results show that specialized modification for interaction point’s pre-
defined location and the extra pose branch improve the precision and recall of classification
compared to the original method;
object detection
enhanced by data
augment and pose
information processed by multi-layer perceptron
are all
able
to
archive the
goal
that both
precision and recall exceed 90%. On this basis, model fusion combining the output ofobject
detection and
pose estimation reaches best recognition accuracy compared with other
methods in this paper, while it just costs a little bit more inference time.
Key words: deep learning,
picking
up
and taking away luggage, action
recognition,
model fusion
II
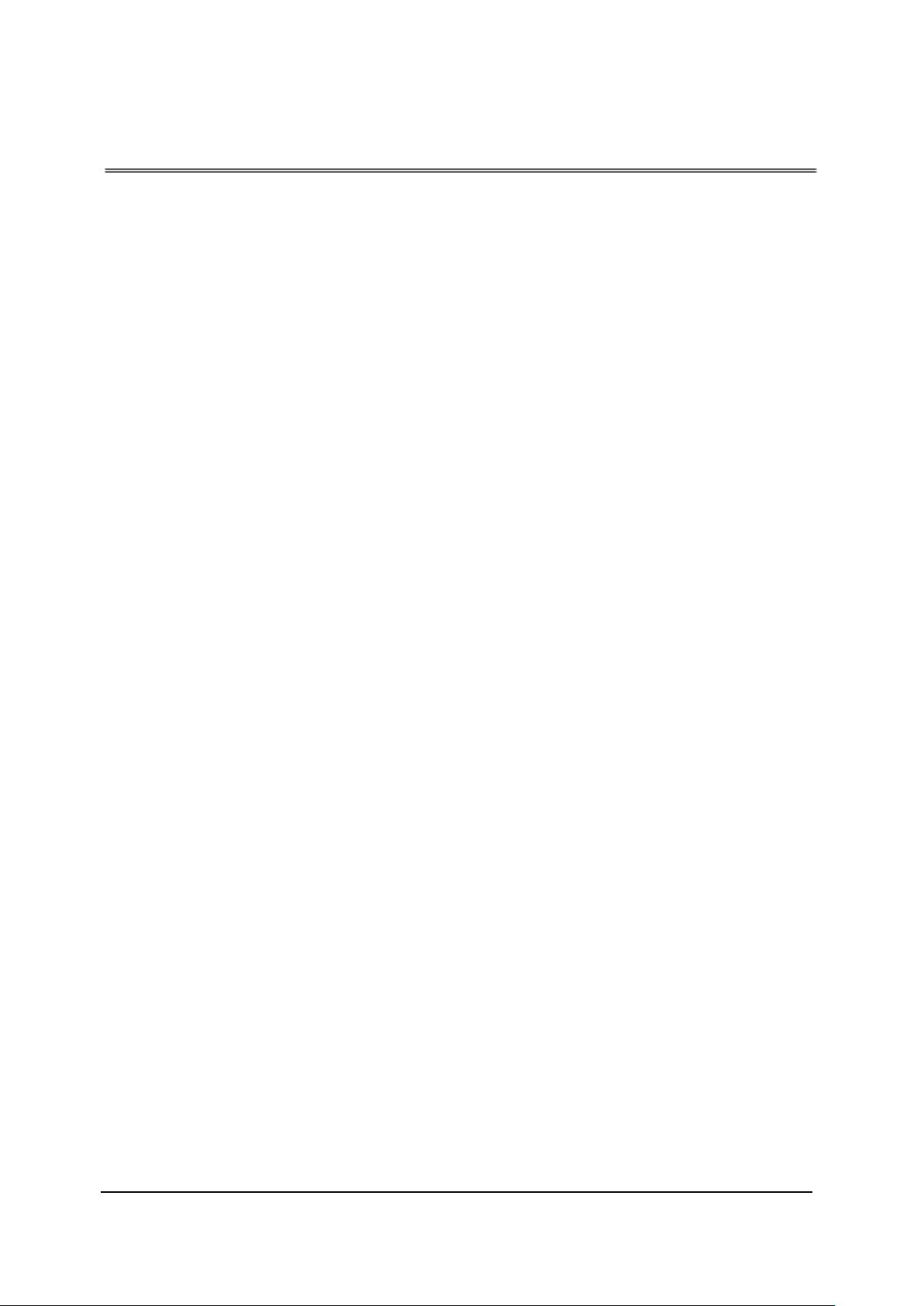
目 录
摘 要.................................................................I
Abstract ............................................................................................................................. II
1 绪论 .............................................................. 1
1.1 课题的研究背景与意义 ............................................ 1
1.2 动作识别国内外研究现状 .......................................... 2
1.3 特定场景下动作识别研究的问题 .................................... 6
1.4 本文主要研究内容 ................................................ 8
1.5 论文章节安排 .................................................... 9
2 基于人、物交互区域预测的行李取放动作识别 ..........................11
2.1 引言 ............................................................11
2.2 本文数据集介绍 ..................................................11
2.3 PPDM 网络框架 ................................................. 18
2.4 人物交互检测的改进 ............................................. 23
2.5 评估指标 ....................................................... 26
2.6 实验结果与分析 ................................................. 28
2.7 本章小结 ....................................................... 33
3 基于目标检测与姿态信息的行李取放动作识别 .......................... 34
3.1 引言 ........................................................... 34
3.2 取放动作识别算法原理与设计 ..................................... 34
3.3 数据处理与数据增强 ............................................. 40
3.4 实验结果与分析 ................................................. 42
3.5 本章小结 ....................................................... 55
4 行李取放行为智能识别系统.......................................... 57
4.1 行李取放行为智能识别系统架构 ................................... 57
4.2 行李取放行为智能识别系统设计与实现 ............................. 58
4.3 行李取放行为智能识别系统测试 ................................... 61
III
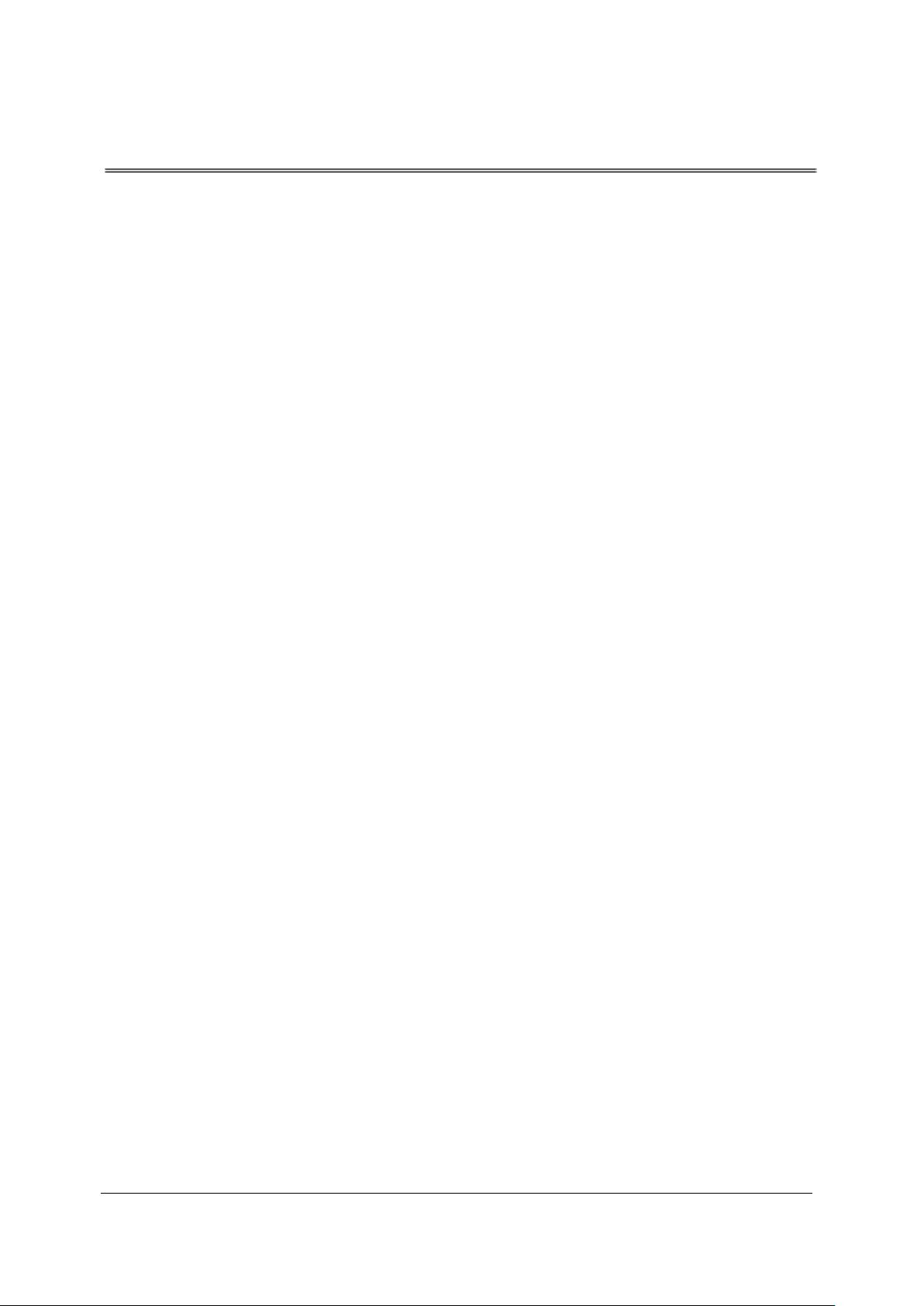
4.4 本章小结 ....................................................... 67
5 总结与展望 ....................................................... 68
5.1 全文总结 ....................................................... 68
5.2 展望 ........................................................... 69
致 谢 ............................................................... 70
参考文献 ............................................................. 71
附录 1 攻读硕士学位期间参加的科研项目目录............................ 77
IV
剩余82页未读,继续阅读
资源评论
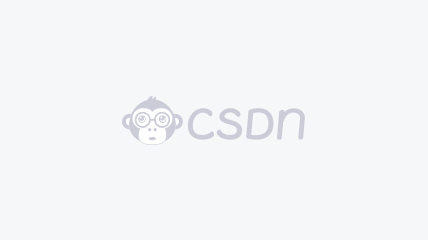

Scikit-learn
- 粉丝: 4280
- 资源: 1868

下载权益

C知道特权

VIP文章

课程特权

开通VIP
上传资源 快速赚钱
我的内容管理 展开
我的资源 快来上传第一个资源
我的收益
登录查看自己的收益我的积分 登录查看自己的积分
我的C币 登录后查看C币余额
我的收藏
我的下载
下载帮助

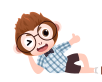
最新资源
- (源码)基于C++的简易操作系统模拟器.zip
- (源码)基于ROS和PCL的激光与UWB定位仿真系统.zip
- (源码)基于Arduino的iBeacon发送系统.zip
- (源码)基于C语言和汇编语言的简单操作系统内核.zip
- (源码)基于Spring Boot框架的AntOA后台管理系统.zip
- (源码)基于Arduino的红外遥控和灯光控制系统.zip
- (源码)基于STM32的简易音乐键盘系统.zip
- (源码)基于Spring Boot和Vue的管理系统.zip
- (源码)基于Spring Boot框架的报表管理系统.zip
- (源码)基于树莓派和TensorFlow Lite的智能厨具环境监测系统.zip
资源上传下载、课程学习等过程中有任何疑问或建议,欢迎提出宝贵意见哦~我们会及时处理!
点击此处反馈


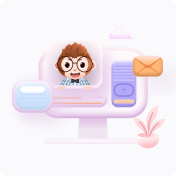
安全验证
文档复制为VIP权益,开通VIP直接复制
