# car_moto_tracking_Jetson_Nano_Yolov8_TensorRT
Detect, track and count cars and motorcycles using yolov8 and TensorRT on Jetson Nano
To deploy this work on a Jetson Nano, you should do it in two steps:
## 1- On your PC:
### Install the required packages:
python -m pip install -r requirements.txt
### Create a folder for the dataset:
mkdir datasets
cd datasets
mkdir Train
mkdir Validation
### Copy your dataset:
Copy your train dataset (images + .txt files) into datasets/Train
cp -r path_to_your_train_dataset/. ${root}/train/datasets/Train
Copy your validation dataset (images + .txt files) into datasets/Validation
cp -r path_to_your_validation_dataset/. ${root}/train/datasets/Validation
If you have more than car and motorcycle classes, modify the data.yaml to add the other classes.
### Train the yolov8n model:
python main.py
When the training is finished, your custom yolov8n model will be saved in
${root}/train/runs/detect/train/weights/best.pt
### 1-6- Push your custom model to GitHub:
git add runs/detect/train/weights/best.onnx
git commit -am "Add the trained yolov8n model"
git push
### Remark:
If you want to use an already pretrained model:
python export_onnx.py --weights path_to_your_pretrained_model
git add path_to_the_onnx_model
git commit -am "Add the trained yolov8n model"
git push
## 2- On the Jetson Nano:
### 2-1- Clone this repo on the Jetson Nano:
git clone "URL to your own repository"
cd "repository_name"
export root=${PWD}
### 2-2- Export the engine from the onnx model
/usr/src/tensorrt/bin/trtexec \
--onnx=train/runs/detect/train/weights/best.onnx \
--saveEngine=best.engine
After executing the above command, you will get an engine named best.engine .
### 2-3- For Detection:
cd ${root}/detect
mkdir build
cd build
cmake ..
make
cd ${root}
#### 2-3-1- Launch Detection:
for video:
${root}/detect/build/yolov8_detect ${root}/best.engine video ${root}/src/test.mp4 1 show
#### Description of all arguments
- 1st argument : path to the maked file
- 2nd argument : path to the engine
- 3rd argument : video for saved video
- 4rth argument: path to video file
- 5th argument : if inference capacity of the Jetson is more then 30 fps, put 1, otherwise put 2, 3, 4 depending on the inference capacity of the Jetson
- 6th argument : show or save
for camera:
${root}/detect/build/yolov8_detect ${root}/best.engine camera 1 show
#### Description of all arguments
- 1st argument : path to the maked file
- 2nd argument : path to the engine
- 3rd argument : camera for using embedded camera
- 4rth argument: if inference capacity of the Jetson is more then 30 fps, put 1, otherwise put 2, 3, 4 depending on the inference capacity of the Jetson
- 5th argument : show or save
### 2-4- For Tracking and Counting:
cd ${root}/track_count
mkdir build
cd build
cmake ..
make
cd ${root}
#### 2-4-1- Launch Tracking and Counting:
If you want to count only in one direction, put 1 as 7th argument. Otherwise, for 2 directions counting, put 2 as 7th argument.
Before displaying the processed video, the first frame of the video will be displayed. You should click on this frame to indicate the position of the line(s). For one direction counting, click twice and for 2 directions counting, click four time.
for video:
${root}/track_count/build/yolov8_track_count ${root}/best.engine video ${root}/src/test.mp4 1 show
#### Description of all arguments
- 1st argument : path to the maked file
- 2nd argument : path to the engine
- 3rd argument : video for saved video
- 4rth argument: path to video file
- 5th argument : if inference capacity of the Jetson is more then 30 fps, put 1, otherwise put 2, 3, 4 depending on the inference capacity of the Jetson
- 6th argument : show or save
for camera:
${root}/track_count/build/yolov8_track_count ${root}/best.engine camera 1 show
#### Description of all arguments
- 1st argument : path to the maked file
- 2nd argument : path to the engine
- 3rd argument : camera for using embedded camera
- 4rth argument: if inference capacity of the Jetson is more then 30 fps, put 1, otherwise put 2, 3, 4 depending on the inference capacity of the Jetson
- 5th argument : show or save
#### Remark:
If you are using the Jetson with SSH, you can not see the first frame of the video to draw the line.
With SSH connection, follow these steps:
1- Add argument to the command line with ssh
for video:
${root}/track_count/build/yolov8_track_count ${root}/best.engine video ${root}/src/test.mp4 1 show ssh
for camera
${root}/track_count/build/yolov8_track_count ${root}/best.engine camera 1 show ssh
2- The first frame of the video will be saved
3- Copie this frame to you PC via SSH (in PC's terminal):
scp jetson_name@jetson_server:path_to_frame_in_jetson/frame_for_line.jpg ${root}/utils/frame_for_line.jpg
4- On your PC, launch the python script draw_line.py to draw the line and get the points.
python3 ${root}/utils/draw_line.py
5- Give the points value to Jetson in the Jetson's terminal.

Mopes__
- 粉丝: 2995
- 资源: 648
最新资源
- 红树林生态系统检测9-YOLO(v5至v9)、COCO、CreateML、Darknet、Paligemma、TFRecord、VOC数据集合集.rar
- 奇安信浏览器安装包下载地址
- 太原理工tyut-数据库真题
- 数学建模宝典:30个通用模型精讲与应用指南(学习文档)
- 航空船、车辆、飞机检测4-YOLO(v5至v11)、COCO、CreateML、Paligemma、TFRecord、VOC数据集合集.rar
- 基于 Selenium 的掘金自动签到、免费抽奖脚本资料齐全+详细文档+高分项目+源码.zip
- 第5章 阶段案例 开发文档.pdf
- 基于 python 的 selenium UI 自动化测试框架,采用 Page Object 设计模式进行二次开发,通过对页面对象和测试代码进行分离,并封装了日
- 基于 Selenium 和 Tkinter 的爬取淘宝商品的Web自动化工具资料齐全+详细文档+高分项目+源码.zip
- 基于 Selenium 爬取招聘岗位信息的基础程序资料齐全+详细文档+高分项目+源码.zip
- 基于 selenium 模拟登录空间QQ 空间爬虫,破解滑动验证码, 抓取好友留言板的所有留言与回复,并生成词图资料齐全+详细文档+高分项目+源码.zip
- 基于 selenium 自动播放b站视频资料齐全+详细文档+高分项目+源码.zip
- 基于 selenium 自动注册apple id的python 脚本资料齐全+详细文档+高分项目+源码.zip
- 基于java的selenium自动化测试框架资料齐全+详细文档+高分项目+源码.zip
- 基于Dagger+Java ScriptEngine+Java Selenium的自动化测试框架资料齐全+详细文档+高分项目+源码.zip
- 基于java的selenium抓取搜狗微信公众号文章资料齐全+详细文档+高分项目+源码.zip
资源上传下载、课程学习等过程中有任何疑问或建议,欢迎提出宝贵意见哦~我们会及时处理!
点击此处反馈


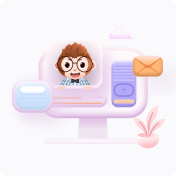