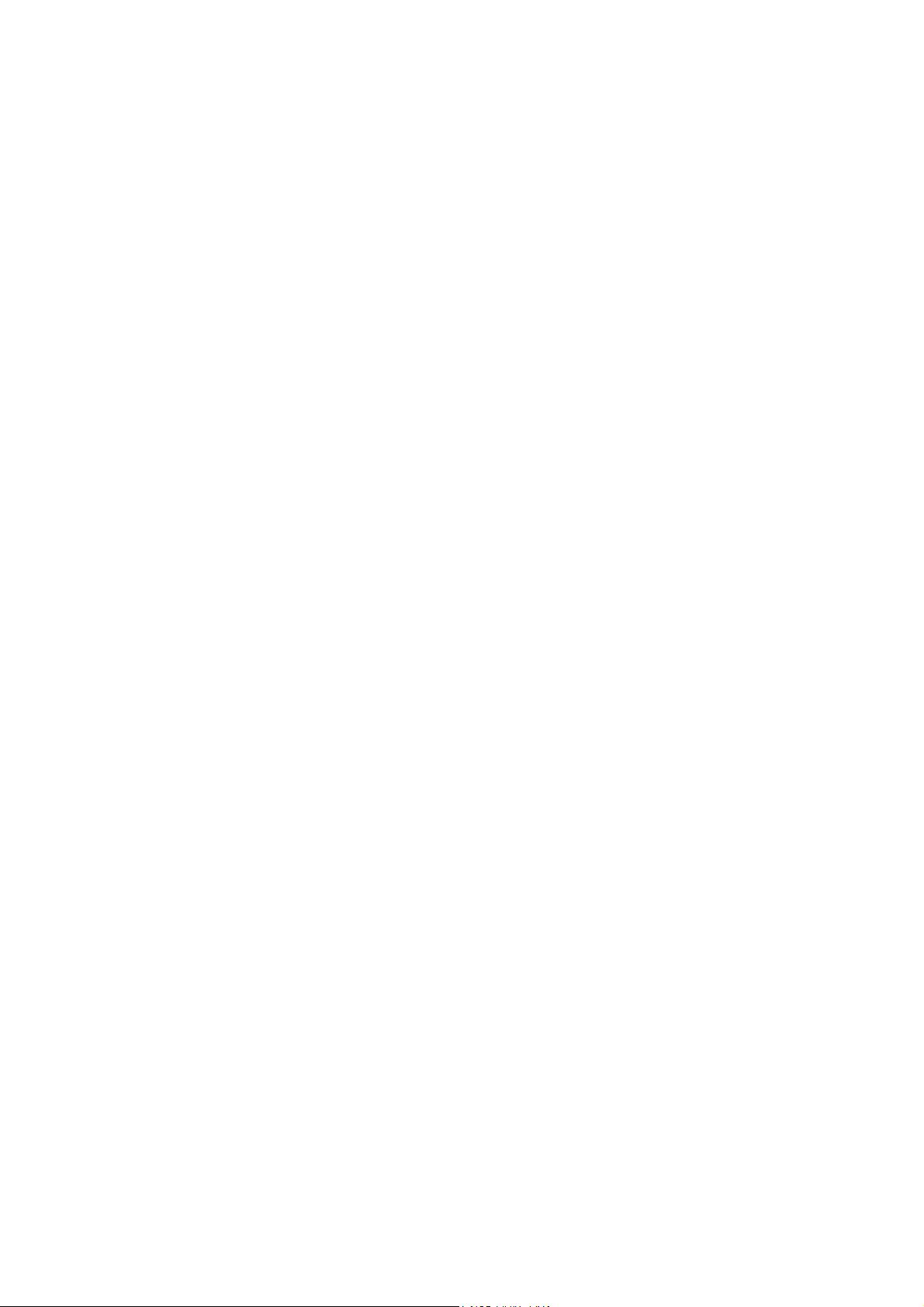
对原 NSGA-II 工具箱进行了修正,将其中一些 Warnings 进行了修改。
有什么问题联系:赵志斌(重庆大学自动化学院),欢迎交流:QQ: 278139331
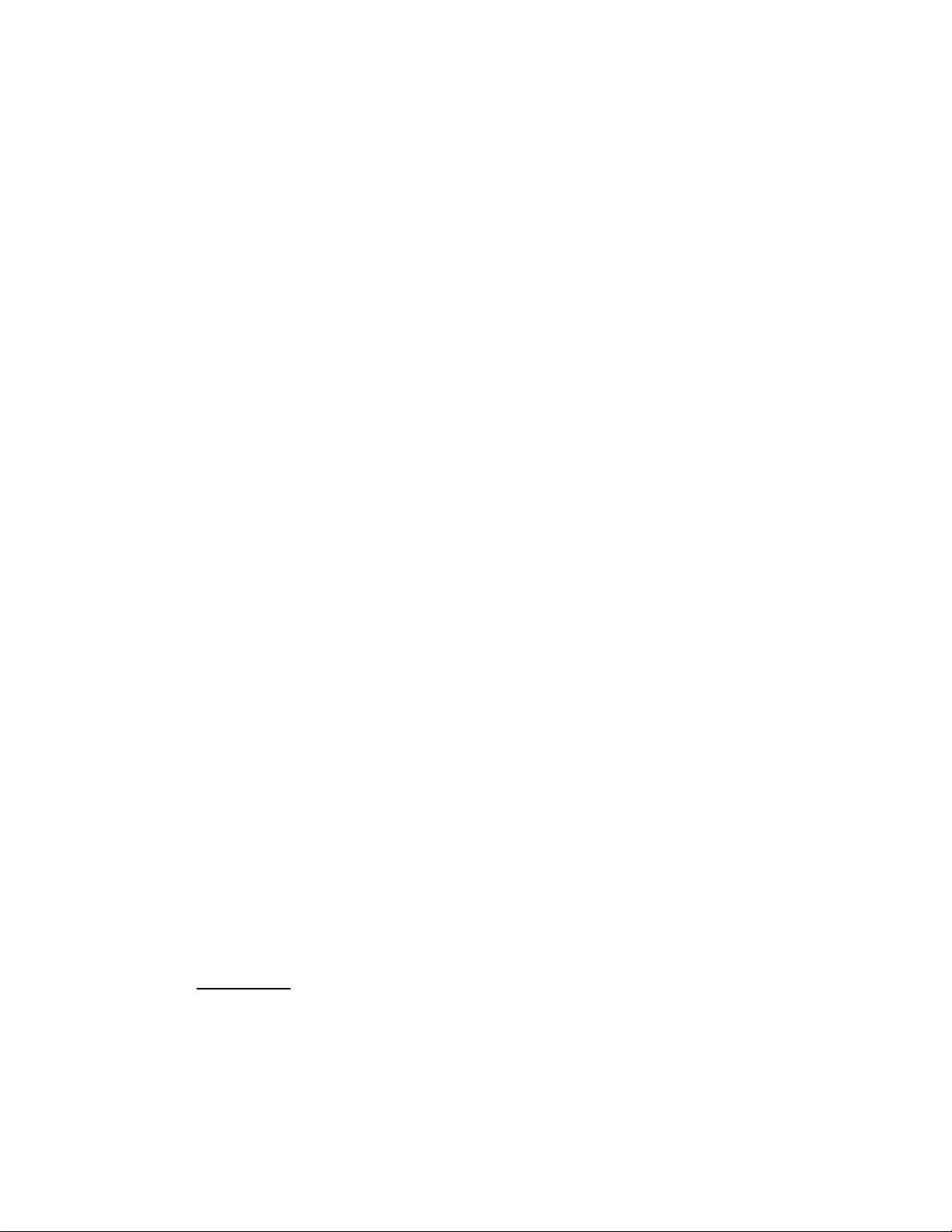
MULTI-OBJECTIVE OPTIMIZATION USING EVOLUTIONARY
ALGORITHMS (MOEA)
ARAVIND SESHADRI
1. Multi-Objective Optimization Using NSGA-II
NSGA (Non-Dominated Sorting in Genetic Algorithms [5]) is a popular non-
domination based genetic algorithm for multi-objective optimization. It is a very
effective algorithm but has been generally criticized for its computational com-
plexity, lack of elitism and for cho osing the optimal parameter value for sharing
parameter σ
share
. A modified version, NSGA-II ( [3]) was developed, which has a
better sorting algorithm , incorporates e litism and no sharing parameter needs to
be chosen a priori. NSGA-II is discussed in detail in this report and two sample
test functions are optimized using it.
2. General Description of NSGA-II
The population is initialized as usual. Once the population in initialized the
population is sorted based on no n-domination into each front. The first front being
completely non-dominant set in the current population and the second front b eing
dominated by the individuals in the first front only and the front goes so on. Ea ch
individual in the each front a re assigned rank (fitness) values or based on front in
which they belong to. Individuals in first front are given a fitness value of 1 and
individuals in second are assigned fitness value as 2 and so on.
In addition to fitness value a new parameter called crowding distance is cal-
culated for each individual. The cr owding distance is a measure of how close an
individual is to its neighbors. Large average crowding distance will res ult in better
diversity in the population.
Parents are selected from the po pulation by using binary tournament selection
based on the rank and crowding distance. An individual is selected in the rank is
lesser than the other or if crowding distance is greater than the other
1
. The selected
population generates offsprings from crossover and mutation operators, which will
be discussed in detail in a later section.
The population with the current population a nd current offsprings is sorted a gain
based on non-domination and only the best N individuals are selected, w here N is
the population size. The selectio n is based on rank and the on crowding distance
on the last front.
3. Detailed Description of NSGA-II
3.1. Population Initialization. The population is initialized based on the prob-
lem range and constr aints if any.
1
Crowding distance is compared only if the rank for both individuals are same
1
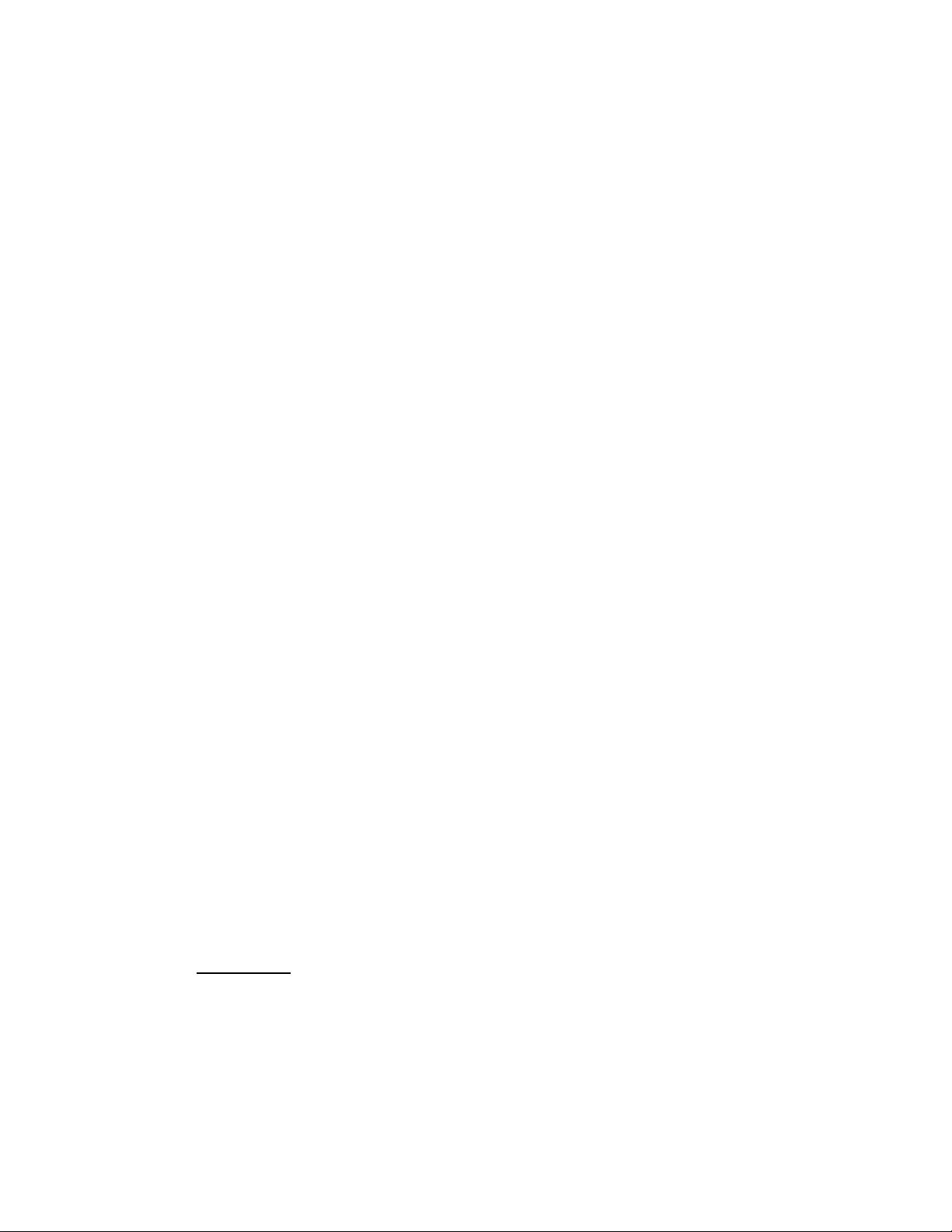
2 ARAVIND SESHADRI
3.2. Non-Dominated sort. The initialized population is sorted based on non-
domination
2
. The fast sort algorithm [3] is described as below for each
• for each individual p in main populatio n P do the following
– Initialize S
p
= ∅. This set would contain all the individuals tha t is
being dominated by p.
– Initialize n
p
= 0. This would be the number of individuals that domi-
nate p.
– for each individual q in P
∗ if p dominated q then
· add q to the set S
p
i.e. S
p
= S
p
∪ {q}
∗ else if q dominates p then
· increment the domination counter for p i.e. n
p
= n
p
+ 1
– if n
p
= 0 i.e. no individuals dominate p then p belongs to the first
front; Set rank of individual p to one i.e p
rank
= 1. Update the first
front set by adding p to front one i.e F
1
= F
1
∪ {p}
• This is carrie d out for all the individuals in main population P .
• Initialize the front c ounter to one. i = 1
• following is carried out while the i
th
front is nonempty i.e. F
i
6= ∅
– Q = ∅. The set for storing the individuals for (i + 1)
th
front.
– for each individual p in front F
i
∗ for each individual q in S
p
(S
p
is the set of individuals dominated
by p)
· n
q
= n
q
−1, decrement the domination count for individual
q.
· if n
q
= 0 then no ne of the individuals in the subsequent
fronts would dominate q. Hence set q
rank
= i + 1. Update
the set Q with individual q i.e. Q = Q ∪ q.
– Increment the front c ounter by one.
– Now the set Q is the next front and hence F
i
= Q.
This algorithm is better than the original NSGA ( [5]) since it utilize the infor-
mation about the set that an individual dominate (S
p
) and number of individuals
that dominate the individual (n
p
).
3.3. Crowding Distance. Once the non-dominated sort is complete the crowding
distance is assigned. Since the individuals are selec ted based on rank and crowding
distance all the individuals in the population are assigned a crowding distance value.
Crowding distance is assigned front wise and comparing the crowding distance
between two individuals in different front is mea ning less. The crowing distance is
calculated as below
• For each front F
i
, n is the number of individuals.
– initialize the dis tance to be zero for a ll the individuals i.e. F
i
(d
j
) = 0,
where j co rresponds to the j
th
individual in front F
i
.
– for each objective function m
∗ Sort the individuals in front F
i
based on objective m i.e. I =
sort(F
i
, m).
2
An individual is said to dominate another i f the objective functions of it is no worse than the
other and at least in one of its objective functions it is better than the other
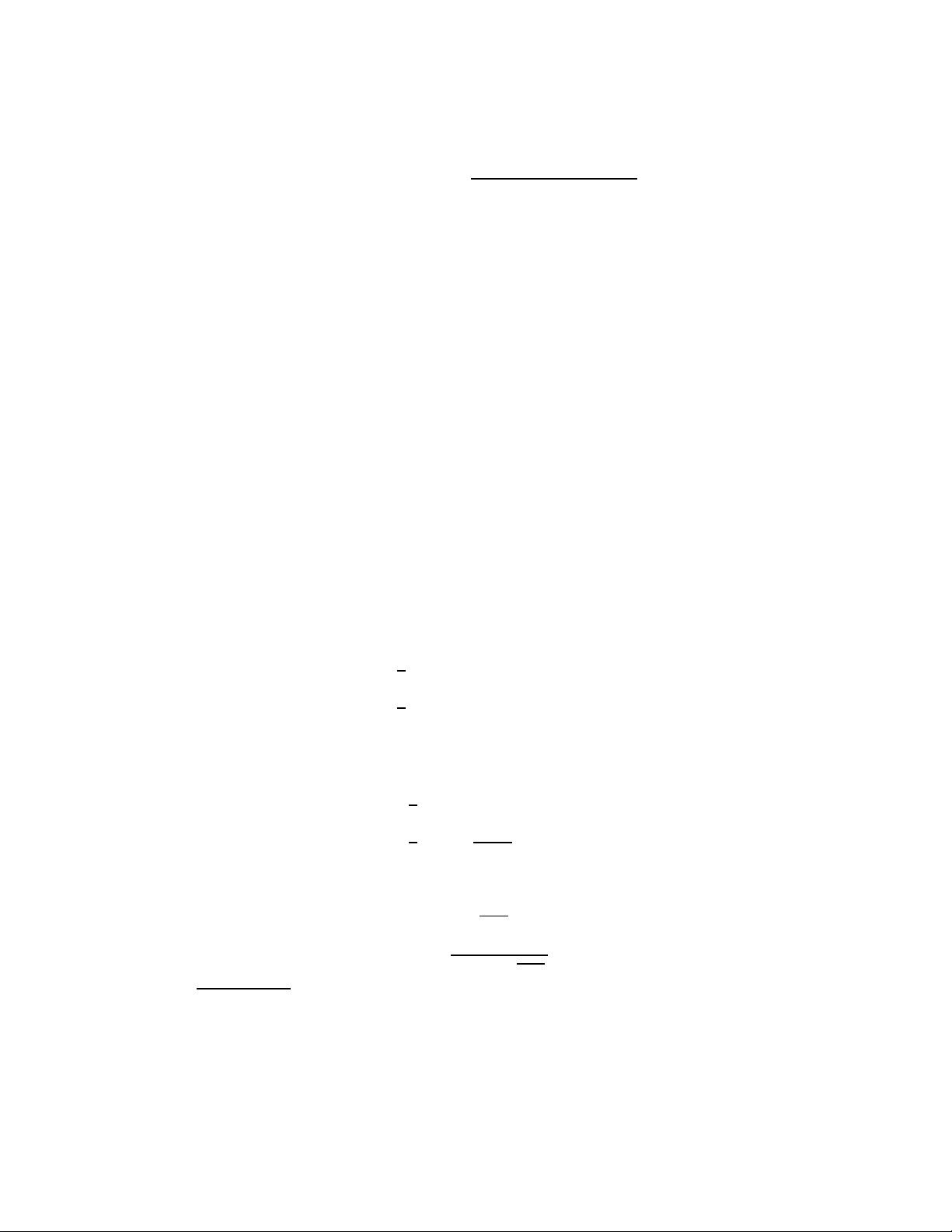
NSGA-II 3
∗ Assign infinite distance to bo undary values for ea ch individual
in F
i
i.e. I(d
1
) = ∞ and I(d
n
) = ∞
∗ for k = 2 to (n − 1)
· I(d
k
) = I(d
k
) +
I(k + 1).m − I(k − 1).m
f
max
m
− f
min
m
· I(k).m is the value of the m
th
objective function of the k
th
individual in I
The basic idea behind the crowing distance is finding the euclidian distance
between each individual in a front based o n their m objectives in the m dimensional
hyper space. The individuals in the boundary are always selected since they have
infinite distance assignment.
3.4. Selection. Once the individuals are sorted based on non-domination and
with crowding distance assigned, the selection is carried out using a crowded-
comparison-operator (≺
n
). The comparison is carried out as below based on
(1) non-domination rank p
rank
i.e. individuals in front F
i
will have their rank
as p
rank
= i.
(2) crowding distance F
i
(d
j
)
• p ≺
n
q if
– p
rank
< q
rank
– or if p and q belong to the same front F
i
then F
i
(d
p
) > F
i
(d
q
) i.e. the
crowing distance should be more.
The individuals ar e selected by using a binary tournament selection with crowed-
comparison- operator.
3.5. Genetic Operators. Real-coded GA’s use Simulated Binary Crossover
(SBX) [2], [1] operator for cross over and polynomial mutation [2], [4].
3.5.1. Simulated Binary Crossover. Simulated binary crossover simulates the bi-
nary crossover observed in nature and is give as below.
c
1,k
=
1
2
[(1 − β
k
)p
1,k
+ (1 + β
k
)p
2,k
]
c
2,k
=
1
2
[(1 + β
k
)p
1,k
+ (1 − β
k
)p
2,k
]
where c
i,k
is the i
th
child w ith k
th
component, p
i,k
is the s e le c ted parent and β
k
(≥ 0) is a sample fro m a random number generated having the density
p(β) =
1
2
(η
c
+ 1)β
η
c
, if 0 ≤ β ≤ 1
p(β) =
1
2
(η
c
+ 1)
1
β
η
c
+2
, if β > 1.
This distribution can be obtained from a uniformly sampled random number u
between (0, 1). η
c
is the distribution index for crossover
3
. That is
β(u ) =(2u)
1
(η+1)
β(u ) =
1
[2(1 − u)]
1
(η+1)
3
This determine how well spread the children will be from their parents.
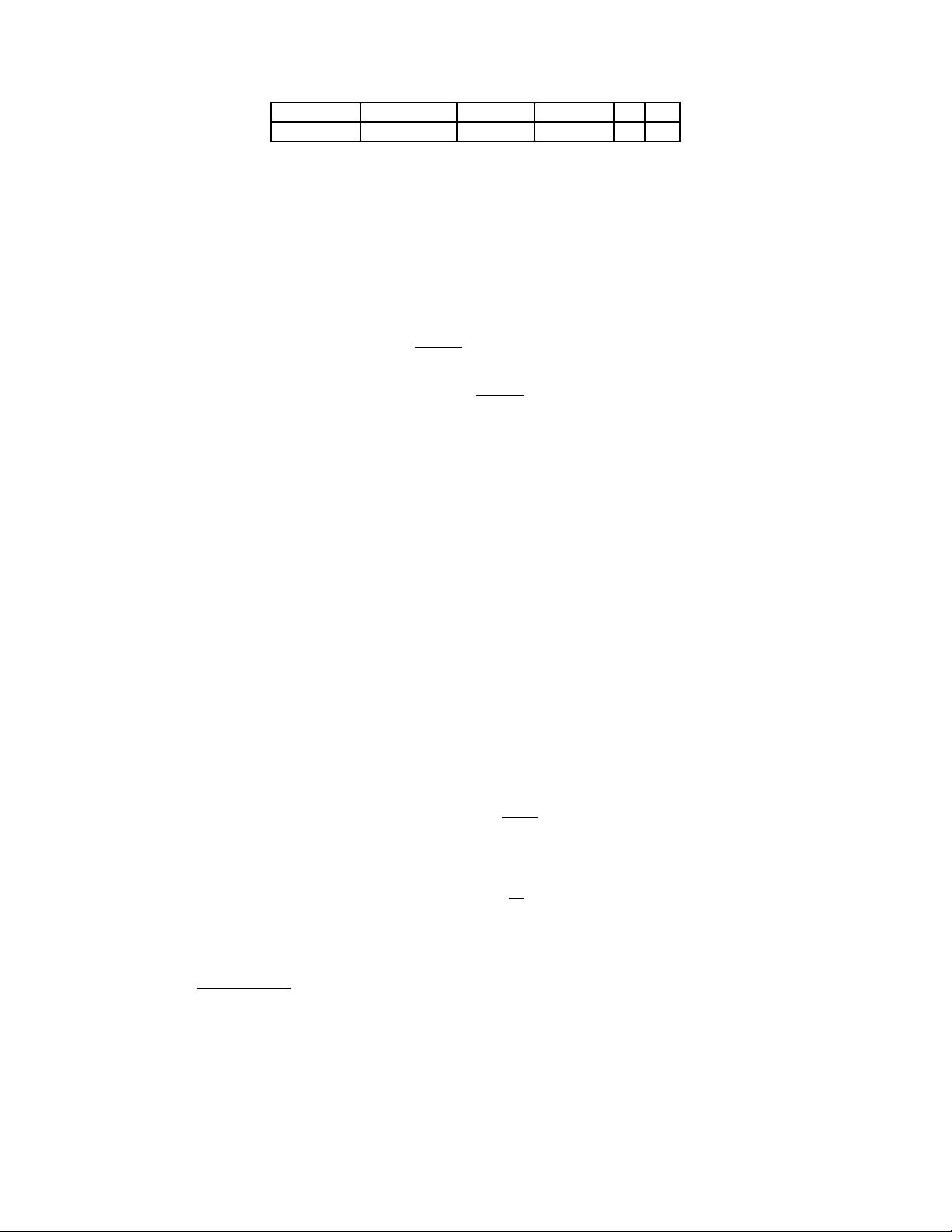
4 ARAVIND SESHADRI
Population Generations Po ol Size Tour Size η
c
η
m
200 500 50 2 20 20
Table 1. MOP1- Parameters for NSGA-II
3.5.2. Polynomial Mutation.
c
k
= p
k
+ (p
u
k
− p
l
k
)δ
k
where c
k
is the child and p
k
is the parent with p
u
k
being the upper bound
4
on
the parent component, p
l
k
is the lower bound and δ
k
is small va riation which is
calculated from a polynomial distribution by using
δ
k
=(2r
k
)
1
η
m
+ 1
− 1, if r
k
< 0.5
δ
k
=1 − [2(1 − r
k
)]
1
η
m
+ 1
if r
k
≥ 0.5
r
k
is an uniformly sampled random numb e r between (0, 1) and η
m
is mutation
distribution index.
3.6. Recombination and Selection. The offspring population is combined with
the current generation population and selection is performed to set the individuals
of the next generation. Since all the previous and current best individuals are
added in the population, elitism is ensure d. Population is now sorted based on
non-domination. The new generation is filled by each front subsequently until the
population size exceeds the current populatio n size. If by adding all the individuals
in front F
j
the population exceeds N then individuals in front F
j
are selected based
on their crowding distance in the descending order until the po pulation size is N.
And hence the proces s repeats to generate the subsequent generations.
4. MOP1 (Example Problem - 1)
This problem is to find the global pareto front for a disc ontinuous function given
by objective function as in [3]
f
1
(x) =1 − e
−4x
1
sin
6
(6πx
1
)
f
2
(x) =g(x)(1 − (
f
1
(x)
g(x)
)
2
)
where,
g(x) =1 + 9(
6
X
i=1
x
i
4
)
0.25
subject to 0 ≤ x
i
≤ 1, i = 1, . . . , 6. This set of objective function r e sulted in the
following solution as shown in Figure 1.
4
The decision space upper bound and lower bound for that particular component.