在进行文本分析时,"分词"是至关重要的一步,它是将一段连续的文本切分成具有语义的独立单元,如单词或短语。在中文处理中,由于汉字没有明显的空格分隔,分词显得更为复杂。常用的中文分词工具有jieba、THULAC、HanLP等,它们通过建立词汇库和算法模型来识别和分割文本中的词语。 "停用词"是指在文本分析中通常被过滤掉的常见词汇,因为它们在大量文本中频繁出现,但往往不包含太多具体信息,例如“的”、“是”、“在”等。停用词表通常根据特定领域或任务进行定制,以减少无意义词汇对分析结果的影响。 在Python中,我们可以利用这些工具进行批量文本处理,例如对大量文档进行关键字词频统计。在《python批量文本分析_指定关键字词频统计并输出csv结果》这篇文章中,作者可能介绍了如何利用Python的文件操作、数据处理库(如pandas)以及分词库(如jieba)来实现这一目标。我们需要读取文本文件,然后使用分词工具进行分词,接着去除停用词,最后统计并输出指定关键字的词频,这个结果通常会保存为CSV格式,便于后续的数据分析和可视化。 下面是一个简化的Python代码示例,演示了上述过程: ```python import jieba from collections import Counter import pandas as pd # 定义停用词表 stopwords = set(['的', '是', '在', ...]) # 批量读取文件 files = ['file1.txt', 'file2.txt', '...'] word_counts = Counter() for file in files: with open(file, 'r', encoding='utf-8') as f: text = f.read() words = jieba.lcut(text) # 使用jieba进行分词 words = [word for word in words if word not in stopwords] # 去除停用词 word_counts.update(words) # 将结果转化为DataFrame并输出为CSV df = pd.DataFrame.from_dict(word_counts, orient='index', columns=['频率']) df.sort_values('频率', ascending=False, inplace=True) df.to_csv('output.csv') ``` 在这个例子中,`needfile`可能是包含待处理文本文件的目录名或压缩包文件名。如果是一个压缩包,可以使用`zipfile`或`tarfile`库来解压文件,然后再进行上述处理。 通过这样的方法,我们可以快速获取大量文本的关键信息,这对于内容分析、情感分析、主题建模等任务非常有用。在实际应用中,可能还需要考虑其他因素,如词语的词性标注、命名实体识别等,以进一步提升分析的准确性和深度。同时,对于特定领域的文本,可能需要定制化的停用词表,以便更准确地提取有意义的信息。


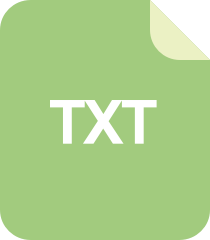
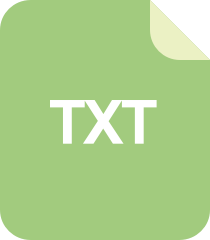
- 1
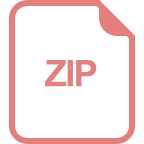
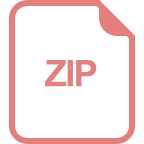
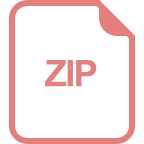
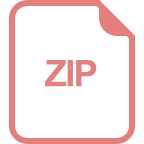
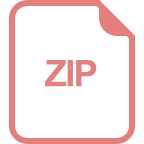
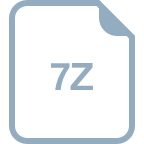
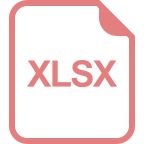
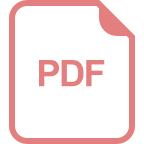
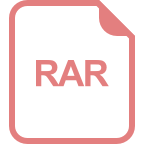
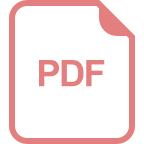

- 粉丝: 7
- 资源: 1
我的内容管理 展开
我的资源 快来上传第一个资源
我的收益
登录查看自己的收益我的积分 登录查看自己的积分
我的C币 登录后查看C币余额
我的收藏
我的下载
下载帮助

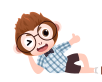
最新资源
- java进销存管理系统(jsp+mssql)130305.rar
- springboot-校友录管理系统.zip
- jeecms-2012.rar
- JSP EIMS系统-OA子系统的设计与开发(源代码+论文).rar
- springboot-学生报名管理系统.zip
- 《大型活动斜坡微路网人群失稳致因分析报告》
- 计算24-3班刘硕丰2406010314.docx
- 全国计算机二级教材pdf
- 树木检测13-YOLO(v5至v9)、COCO、CreateML、Darknet、Paligemma、TFRecord、VOC数据集合集.rar
- python入门基础教程易学易懂.pdf
- 元气桌面壁纸9.05VIP版.apk
- 计算机二级-计算机二级考试Java语言题集+题解.zip
- 双向链表的定义、初始化、打印、插入、删除、查找、销毁等操作的实现及测试代码
- 树木检测16-YOLO(v5至v9)、COCO、CreateML、Darknet、Paligemma、TFRecord、VOC数据集合集.rar
- resnet50-0676ba61.pth
- QQGameMini_1080001462_cid0.exe

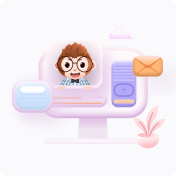

评论0