没有合适的资源?快使用搜索试试~ 我知道了~
资源推荐
资源详情
资源评论
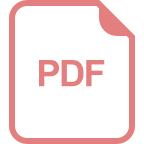
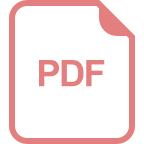
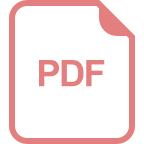
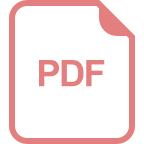
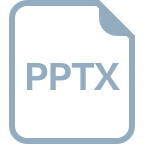
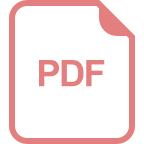
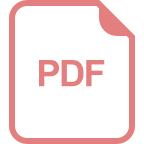
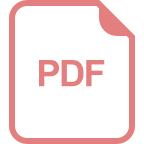
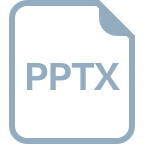
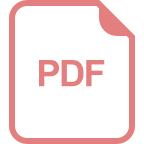
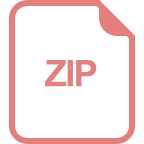
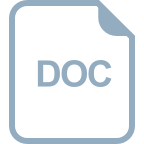
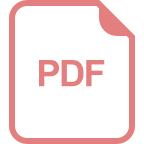
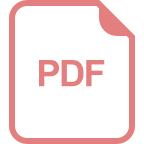
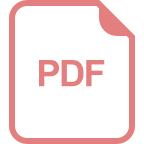
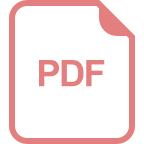
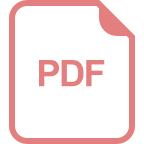
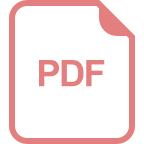
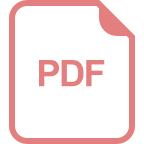
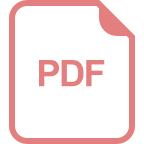
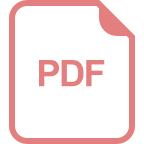
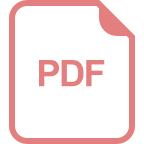
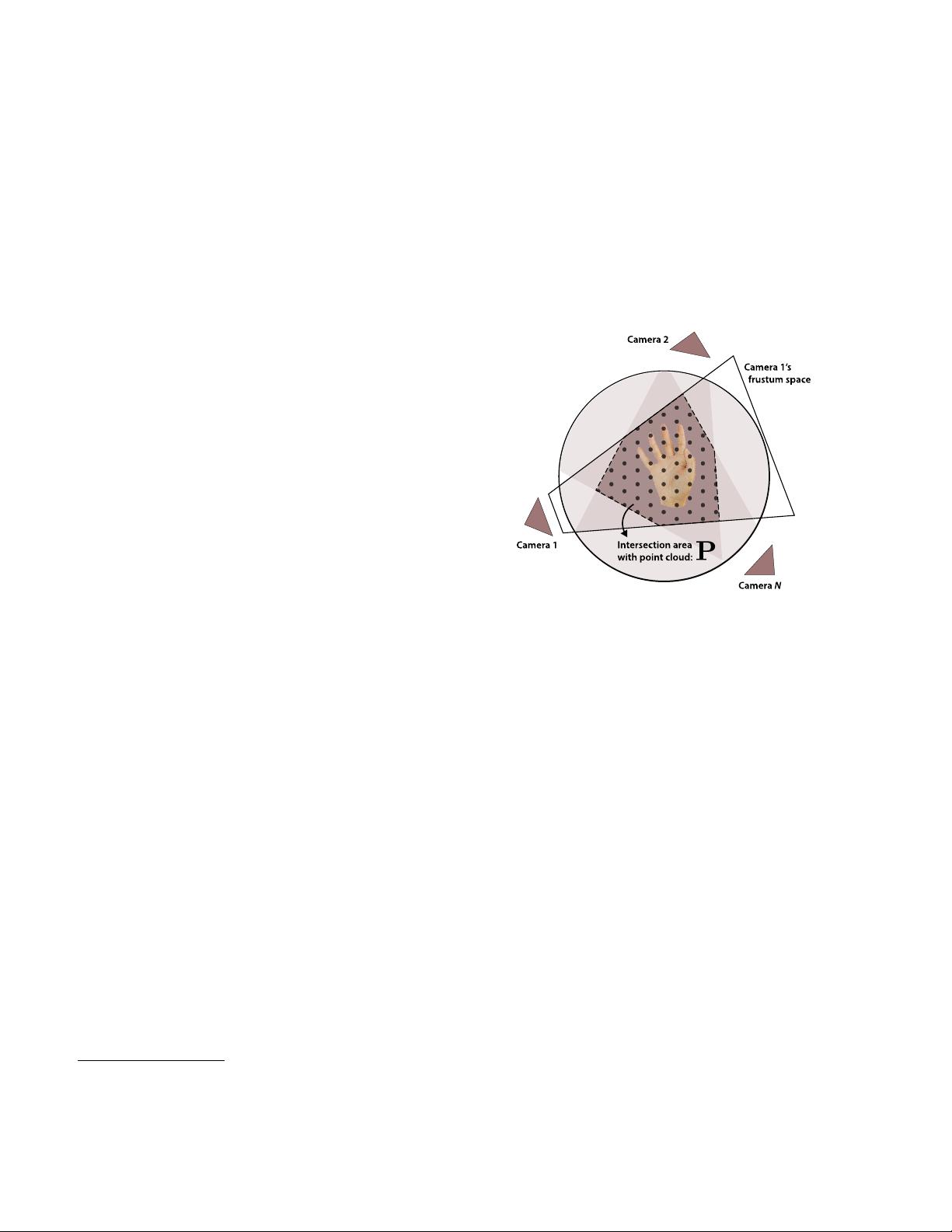
POEM: Reconstructing Hand in a Point Embedded Multi-view Stereo
Lixin Yang
1,2
Jian Xu
3
Licheng Zhong
1
Xinyu Zhan
1
Zhicheng Wang
3
Kejian Wu
3
Cewu Lu
1,2†
1
Shanghai Jiao Tong University
2
Shanghai Qi Zhi Institute
3
Nreal
{siriusyang, zlicheng, kelvin34501, lucewu}@sjtu.edu.cn
{jianxu, kejian}@nreal.ai chgggo@gmail.com
Abstract
Enable neural networks to capture 3D geometrical-
aware features is essential in multi-view based vision tasks.
Previous methods usually encode the 3D information of
multi-view stereo into the 2D features. In contrast, we
present a novel method, named POEM, that directly oper-
ates on the 3D POints Embedded in the Multi-view stereo
for reconstructing hand mesh in it. Point is a natural form
of 3D information and an ideal medium for fusing fea-
tures across views, as it has different projections on dif-
ferent views. Our method is thus in light of a simple yet
effective idea, that a complex 3D hand mesh can be rep-
resented by a set of 3D points that 1) are embedded in
the multi-view stereo, 2) carry features from the multi-view
images, and 3) encircle the hand. To leverage the power
of points, we design two operations: point-based feature
fusion and cross-set point attention mechanism. Evalua-
tion on three challenging multi-view datasets shows that
POEM outperforms the state-of-the-art in hand mesh re-
construction. Code and models are available for research
at github.com/lixiny/POEM
1. Introduction
Hand mesh reconstruction plays a central role in the field
of augmented and mixed reality, as it can not only deliver
realistic experiences for the users in gaming but also sup-
port applications involving teleoperation, communication,
education, and fitness outside of gaming. Many significant
efforts have been made for the monocular 3D hand mesh
reconstruction [1, 5, 7, 9, 31, 32]. However, it still strug-
gles to produce applicable results, mainly for these three
reasons. (1) Depth ambiguity. Recovery of the absolute
position in a monocular camera system is an ill-posed prob-
lem. Hence, previous methods [9, 31, 54] only recovered
the hand vertices relative to the wrist (i.e. root-relative).
(2) Unknown perspectives. The shape of the hand’s 2D
†
Cewu Lu is the corresponding author, the member of Qing Yuan Re-
search Institute and MoE Key Lab of Artificial Intelligence, AI Institute,
Shanghai Jiao Tong University, China and Shanghai Qi Zhi institute.
Figure 1. Intersection area of N cameras’ frustum spaces. The
gray dots represent the point cloud P aggregated from N frustums.
Our method: POEM, standing for the point embedded multi-view
stereo, focuses on the dark area scatted with gray dots.
projection is highly dependent on the camera’s perspec-
tive model (i.e. camera intrinsic matrix). However, the
monocular-based methods usually suggest a weak perspec-
tive projection [1, 27], which is not accurate enough to re-
cover the hand’s 3D structure. (3) Occlusion. The occlu-
sion between the hand and its interacting objects also chal-
lenges the accuracy of the reconstruction [32]. These issues
limit monocular-based methods from practical application,
in which the absolute and accurate position of the hand sur-
face is required for interacting with our surroundings.
Our paper is thus focusing on reconstructing hands from
multi-view images. Motivation comes from two aspects.
First, the issues mentioned above can be alleviated by lever-
aging the geometrical consistency among multi-view im-
ages. Second, the prospered multi-view hand-object track-
ing setups [2, 4, 49, 55] and VR headsets bring us an urgent
demand and direct application of multi-view hand recon-
struction in real-time. A common practice of multi-view 3D
pose estimation follows a two-stage design. It first estimates
the 2D key points of the skeleton in each view and then
back-project them to 3D space through several 2D-to-3D
lifting methods, e.g. algebraic triangulation [17,18,39], Pic-
torial Structures Model (PSM) [33, 38], 3D CNN [18, 43],
21108
2023 IEEE/CVF Conference on Computer Vision and Pattern Recognition (CVPR)
979-8-3503-0129-8/23/$31.00 ©2023 IEEE
DOI 10.1109/CVPR52729.2023.02022
2023 IEEE/CVF Conference on Computer Vision and Pattern Recognition (CVPR) | 979-8-3503-0129-8/23/$31.00 ©2023 IEEE | DOI: 10.1109/CVPR52729.2023.02022
Authorized licensed use limited to: Institute of Software. Downloaded on November 08,2024 at 02:43:11 UTC from IEEE Xplore. Restrictions apply.
资源评论
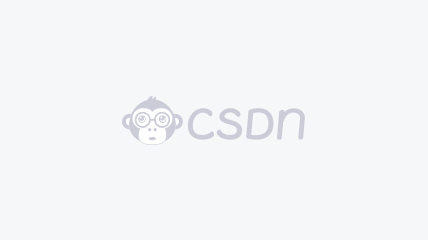


GL_Rain
- 粉丝: 677
- 资源: 5
上传资源 快速赚钱
我的内容管理 展开
我的资源 快来上传第一个资源
我的收益
登录查看自己的收益我的积分 登录查看自己的积分
我的C币 登录后查看C币余额
我的收藏
我的下载
下载帮助

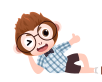
最新资源
资源上传下载、课程学习等过程中有任何疑问或建议,欢迎提出宝贵意见哦~我们会及时处理!
点击此处反馈


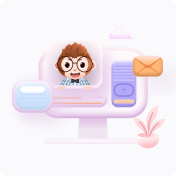
安全验证
文档复制为VIP权益,开通VIP直接复制
