<a href="https://apps.apple.com/app/id1452689527" target="_blank">
<img src="https://user-images.githubusercontent.com/26833433/82944393-f7644d80-9f4f-11ea-8b87-1a5b04f555f1.jpg" width="1000"></a>
 

This repository represents Ultralytics open-source research into future object detection methods, and incorporates our lessons learned and best practices evolved over training thousands of models on custom client datasets with our previous YOLO repository https://github.com/ultralytics/yolov3. **All code and models are under active development, and are subject to modification or deletion without notice.** Use at your own risk.
<img src="https://user-images.githubusercontent.com/26833433/85340570-30360a80-b49b-11ea-87cf-bdf33d53ae15.png" width="1000">** GPU Speed measures end-to-end time per image averaged over 5000 COCO val2017 images using a V100 GPU with batch size 8, and includes image preprocessing, PyTorch FP16 inference, postprocessing and NMS.
- **July 23, 2020**: [v2.0 release](https://github.com/ultralytics/yolov5/releases/tag/v2.0): improved model definition, training and mAP.
- **June 22, 2020**: [PANet](https://arxiv.org/abs/1803.01534) updates: new heads, reduced parameters, improved speed and mAP [364fcfd](https://github.com/ultralytics/yolov5/commit/364fcfd7dba53f46edd4f04c037a039c0a287972).
- **June 19, 2020**: [FP16](https://pytorch.org/docs/stable/nn.html#torch.nn.Module.half) as new default for smaller checkpoints and faster inference [d4c6674](https://github.com/ultralytics/yolov5/commit/d4c6674c98e19df4c40e33a777610a18d1961145).
- **June 9, 2020**: [CSP](https://github.com/WongKinYiu/CrossStagePartialNetworks) updates: improved speed, size, and accuracy (credit to @WongKinYiu for CSP).
- **May 27, 2020**: Public release. YOLOv5 models are SOTA among all known YOLO implementations.
- **April 1, 2020**: Start development of future compound-scaled [YOLOv3](https://github.com/ultralytics/yolov3)/[YOLOv4](https://github.com/AlexeyAB/darknet)-based PyTorch models.
## Pretrained Checkpoints
| Model | AP<sup>val</sup> | AP<sup>test</sup> | AP<sub>50</sub> | Speed<sub>GPU</sub> | FPS<sub>GPU</sub> || params | FLOPS |
|---------- |------ |------ |------ | -------- | ------| ------ |------ | :------: |
| [YOLOv5s](https://drive.google.com/open?id=1Drs_Aiu7xx6S-ix95f9kNsA6ueKRpN2J) | 36.1 | 36.1 | 55.3 | **2.1ms** | **476** || 7.5M | 13.2B
| [YOLOv5m](https://drive.google.com/open?id=1Drs_Aiu7xx6S-ix95f9kNsA6ueKRpN2J) | 43.5 | 43.5 | 62.5 | 3.0ms | 333 || 21.8M | 39.4B
| [YOLOv5l](https://drive.google.com/open?id=1Drs_Aiu7xx6S-ix95f9kNsA6ueKRpN2J) | 47.0 | 47.1 | 65.6 | 3.9ms | 256 || 47.8M | 88.1B
| [YOLOv5x](https://drive.google.com/open?id=1Drs_Aiu7xx6S-ix95f9kNsA6ueKRpN2J) | **49.0** | **49.0** | **67.4** | 6.1ms | 164 || 89.0M | 166.4B
| | | | | | || |
| [YOLOv3-SPP](https://drive.google.com/open?id=1Drs_Aiu7xx6S-ix95f9kNsA6ueKRpN2J) | 45.6 | 45.5 | 65.2 | 4.5ms | 222 || 63.0M | 118.0B
** AP<sup>test</sup> denotes COCO [test-dev2017](http://cocodataset.org/#upload) server results, all other AP results in the table denote val2017 accuracy.
** All AP numbers are for single-model single-scale without ensemble or test-time augmentation. Reproduce by `python test.py --data coco.yaml --img 672 --conf 0.001`
** Speed<sub>GPU</sub> measures end-to-end time per image averaged over 5000 COCO val2017 images using a GCP [n1-standard-16](https://cloud.google.com/compute/docs/machine-types#n1_standard_machine_types) instance with one V100 GPU, and includes image preprocessing, PyTorch FP16 image inference at --batch-size 32 --img-size 640, postprocessing and NMS. Average NMS time included in this chart is 1-2ms/img. Reproduce by `python test.py --data coco.yaml --img 640 --conf 0.1`
** All checkpoints are trained to 300 epochs with default settings and hyperparameters (no autoaugmentation).
## Requirements
Python 3.7 or later with all `requirements.txt` dependencies installed, including `torch >= 1.5`. To install run:
```bash
$ pip install -U -r requirements.txt
```
## Tutorials
* [Train Custom Data](https://github.com/ultralytics/yolov5/wiki/Train-Custom-Data)
* [Multi-GPU Training](https://github.com/ultralytics/yolov5/issues/475)
* [PyTorch Hub](https://github.com/ultralytics/yolov5/issues/36)
* [ONNX and TorchScript Export](https://github.com/ultralytics/yolov5/issues/251)
* [Test-Time Augmentation (TTA)](https://github.com/ultralytics/yolov5/issues/303)
* [Model Ensembling](https://github.com/ultralytics/yolov5/issues/318)
* [Model Pruning/Sparsity](https://github.com/ultralytics/yolov5/issues/304)
## Environments
YOLOv5 may be run in any of the following up-to-date verified environments (with all dependencies including [CUDA](https://developer.nvidia.com/cuda)/[CUDNN](https://developer.nvidia.com/cudnn), [Python](https://www.python.org/) and [PyTorch](https://pytorch.org/) preinstalled):
- **Google Colab Notebook** with free GPU: <a href="https://colab.research.google.com/github/ultralytics/yolov5/blob/master/tutorial.ipynb"><img src="https://colab.research.google.com/assets/colab-badge.svg" alt="Open In Colab"></a>
- **Kaggle Notebook** with free GPU: [https://www.kaggle.com/ultralytics/yolov5](https://www.kaggle.com/ultralytics/yolov5)
- **Google Cloud** Deep Learning VM. See [GCP Quickstart Guide](https://github.com/ultralytics/yolov5/wiki/GCP-Quickstart)
- **Docker Image** https://hub.docker.com/r/ultralytics/yolov5. See [Docker Quickstart Guide](https://github.com/ultralytics/yolov5/wiki/Docker-Quickstart) 
## Inference
Inference can be run on most common media formats. Model [checkpoints](https://drive.google.com/open?id=1Drs_Aiu7xx6S-ix95f9kNsA6ueKRpN2J) are downloaded automatically if available. Results are saved to `./inference/output`.
```bash
$ python detect.py --source 0 # webcam
file.jpg # image
file.mp4 # video
path/ # directory
path/*.jpg # glob
rtsp://170.93.143.139/rtplive/470011e600ef003a004ee33696235daa # rtsp stream
http://112.50.243.8/PLTV/88888888/224/3221225900/1.m3u8 # http stream
```
To run inference on examples in the `./inference/images` folder:
```bash
$ python detect.py --source ./inference/images/ --weights yolov5s.pt --conf 0.4
Namespace(agnostic_nms=False, augment=False, classes=None, conf_thres=0.4, device='', fourcc='mp4v', half=False, img_size=640, iou_thres=0.5, output='inference/output', save_txt=False, source='./inference/images/', view_img=False, weights='yolov5s.pt')
Using CUDA device0 _CudaDeviceProperties(name='Tesla P100-PCIE-16GB', total_memory=16280MB)
Downloading https://drive.google.com/uc?export=download&id=1R5T6rIyy3lLwgFXNms8whc-387H0tMQO as yolov5s.pt... Done (2.6s)
image 1/2 inference/images/bus.jpg: 640x512 3 persons, 1 buss, Done. (0.009s)
image 2/2 inference/images/zidane.jpg: 384x640 2 persons, 2 ties, Done. (0.009s)
Results saved to /content/yolov5/inference/output
```
<img src="https://user-images.githubusercontent.com/26833433/83082816-59e54880-a039-11ea-8abe-ab90cc1ec4b0.jpeg" width="500">
## Training
Download [COCO](https://github.com/ultralytics/yolov5/blob/master/data/get_coco2017.sh), install [Apex](https://github.com/NVIDIA/apex) and run command below. Training times for YOLOv5s/m/l/x are 2/4/6/8 days on a single V100 (multi-GPU times faster). Use the largest `--batch-size` your GPU allows (batch sizes shown for 16 GB devices).
```bash
$ python train.py --data coco.yaml --cfg yolov5s.yaml --weights '' --batch-size 64
yolov5m
没有合适的资源?快使用搜索试试~ 我知道了~
温馨提示
深度学习-YOLO目标检测yolov5抽烟识别检测数据集(5000张图片数据),吸烟行为检测数据集,5000多张使用lableimg软件标注软件标注好的吸烟数据,图片格式为jpg,标签有两种,分别为xml格式和txt格式
资源推荐
资源详情
资源评论
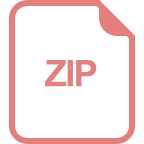
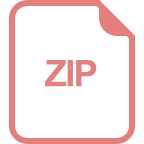
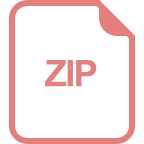
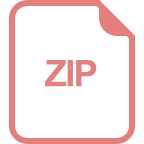
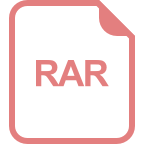
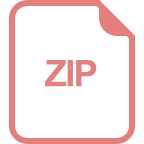
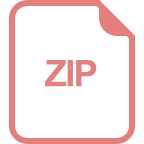
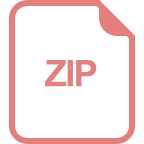
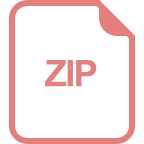
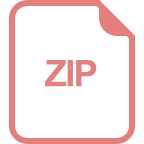
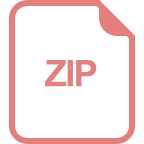
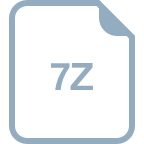
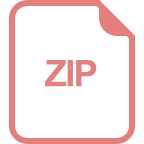
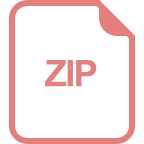
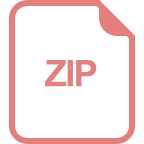
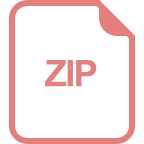
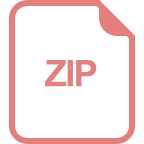
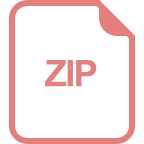
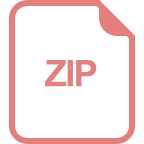
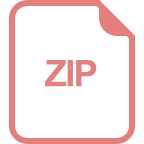
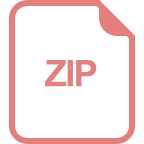
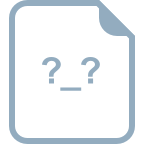
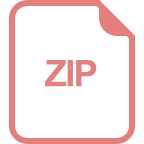
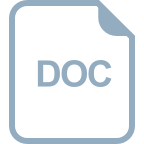
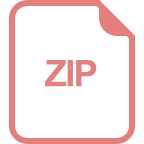
收起资源包目录

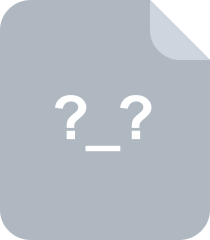
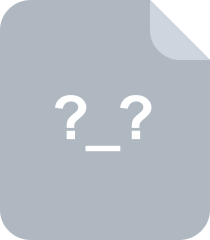
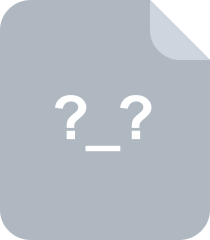
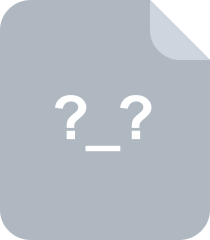
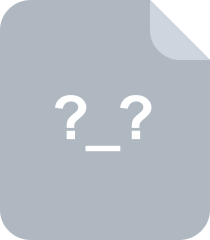
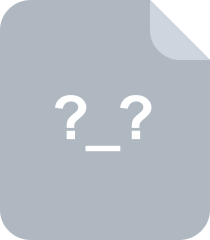
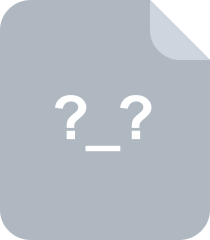
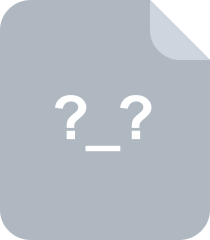
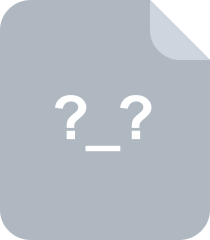
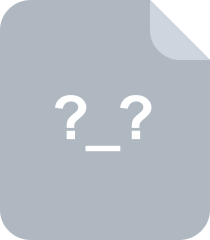
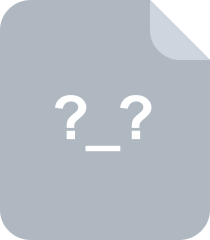
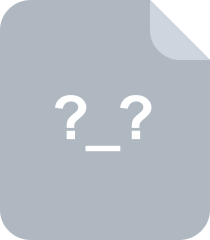
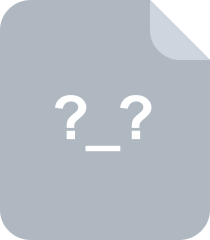
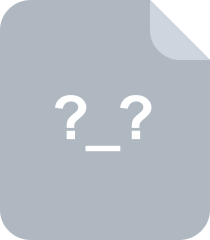
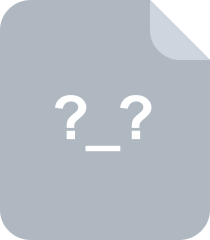
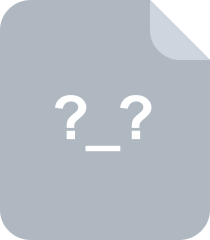
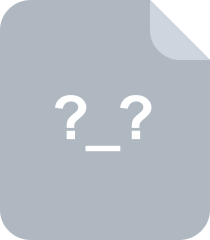
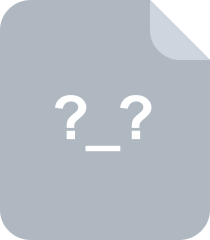
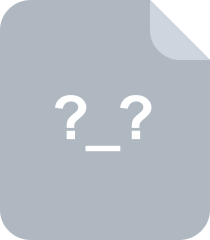
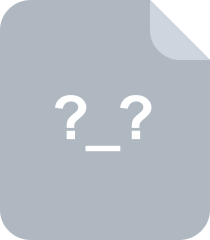
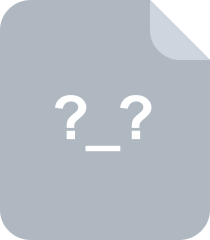
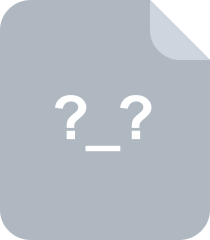
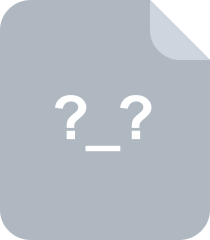
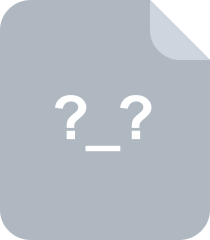
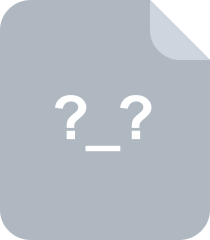
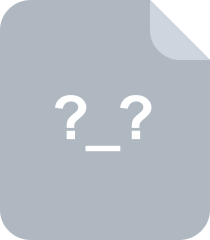
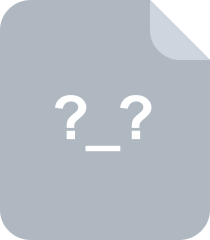
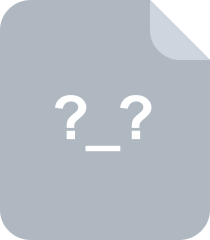
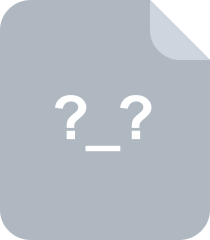
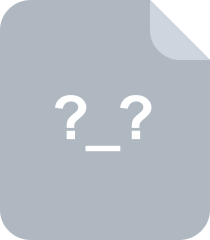
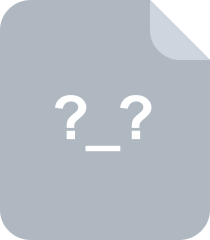
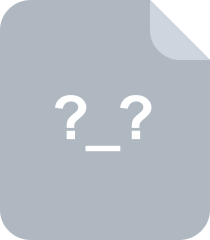
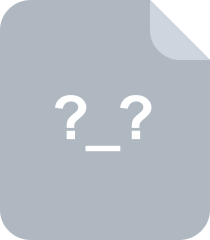
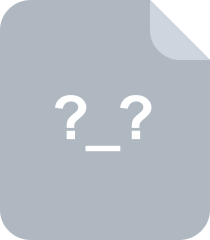
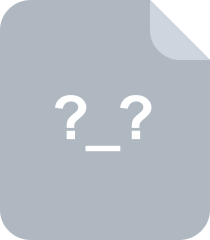
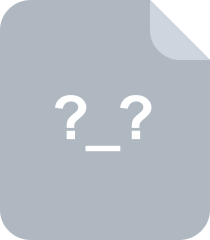
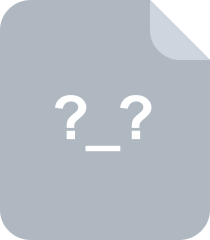
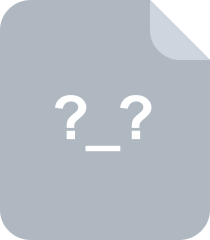
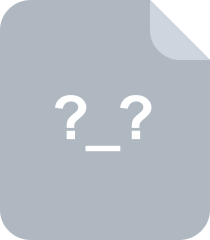
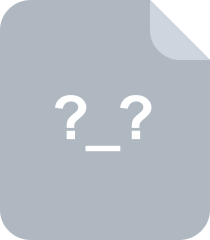
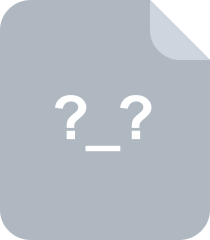
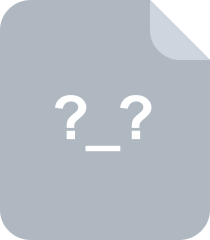
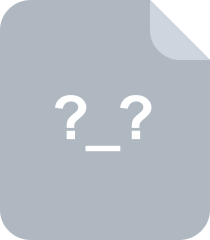
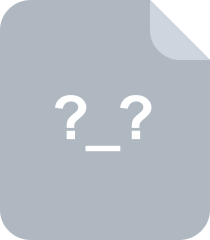
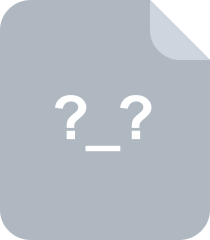
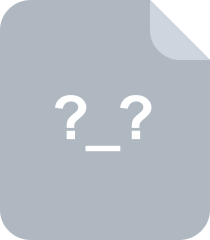
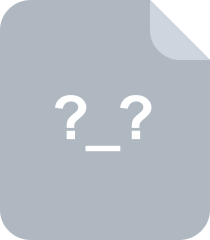
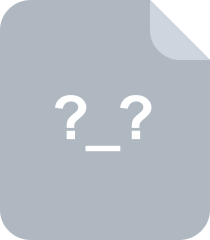
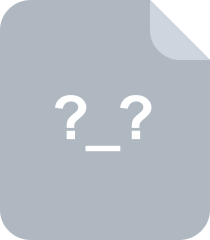
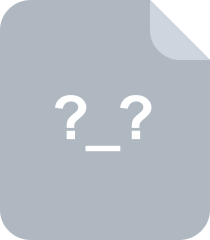
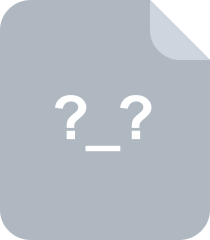
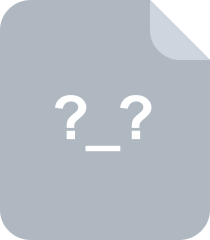
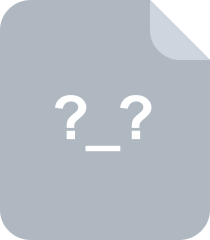
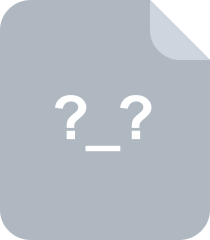
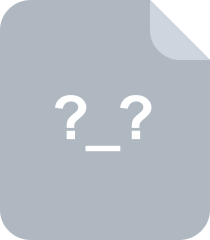
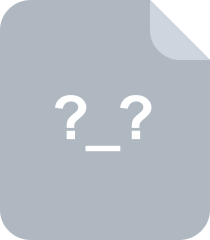
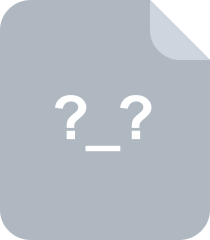
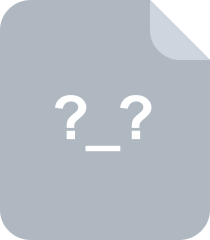
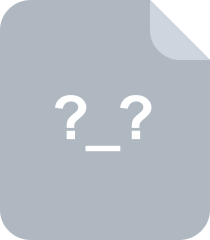
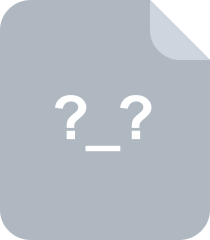
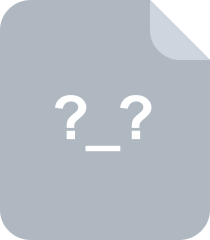
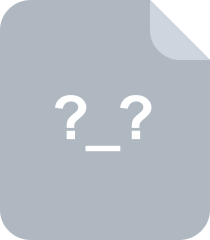
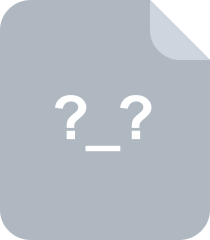
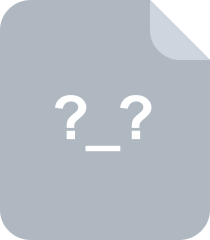
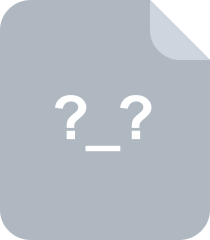
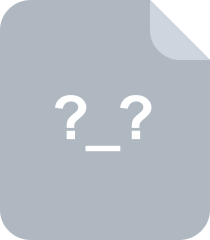
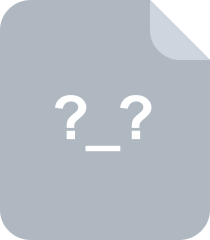
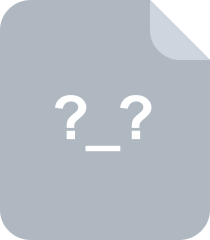
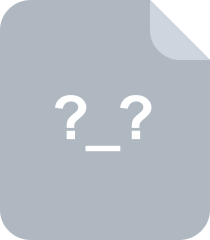
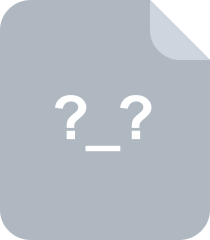
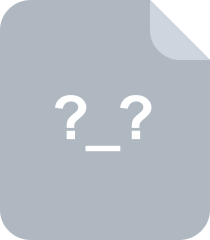
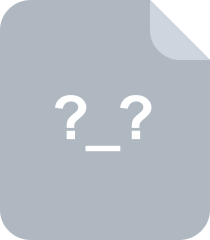
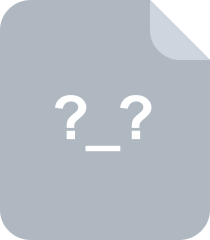
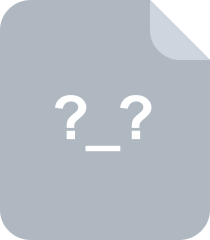
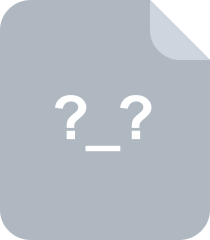
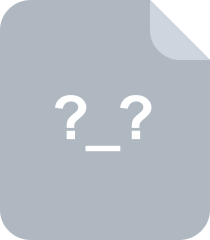
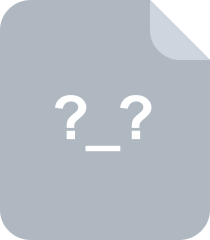
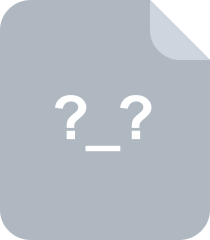
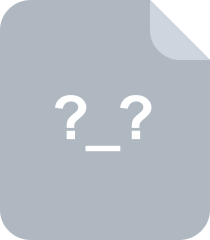
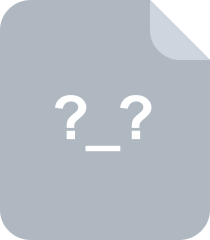
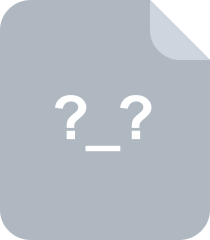
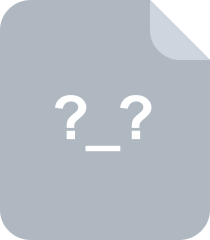
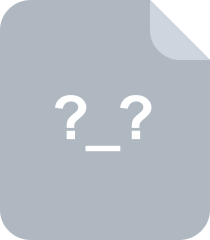
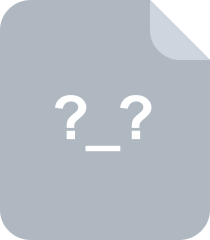
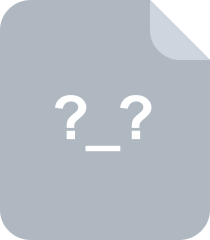
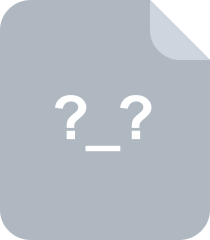
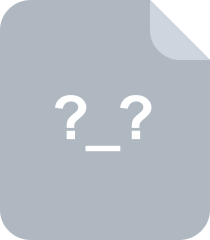
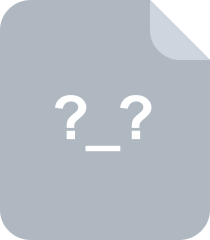
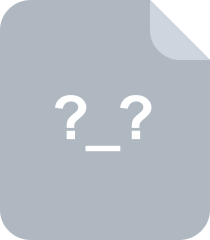
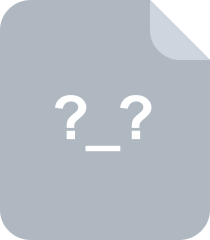
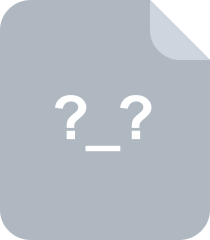
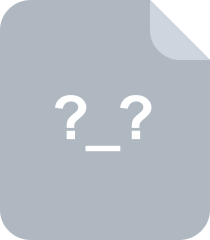
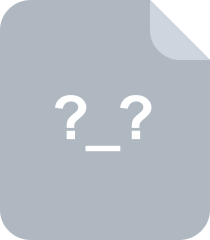
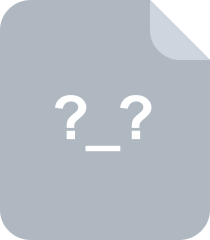
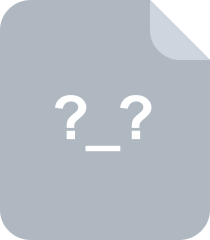
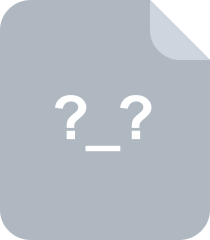
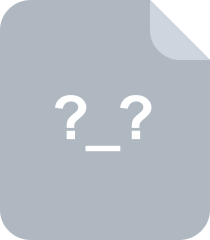
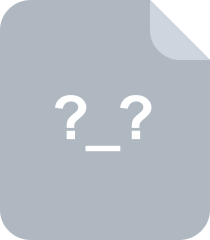
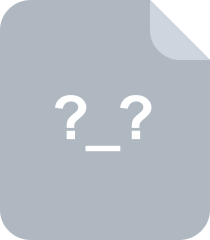
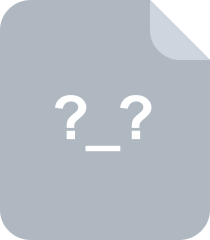
共 2000 条
- 1
- 2
- 3
- 4
- 5
- 6
- 20
资源评论
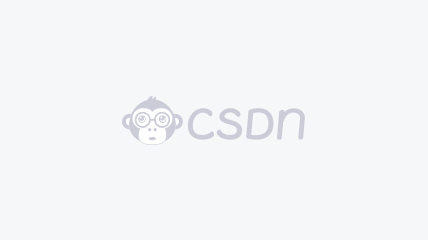
- Myysq6610262024-09-17非常有用的资源,有一定的参考价值,受益匪浅,值得下载。
- kefu1992024-06-18发现一个宝藏资源,资源有很高的参考价值,赶紧学起来~

GJZGRB
- 粉丝: 2955
- 资源: 7736
上传资源 快速赚钱
我的内容管理 展开
我的资源 快来上传第一个资源
我的收益
登录查看自己的收益我的积分 登录查看自己的积分
我的C币 登录后查看C币余额
我的收藏
我的下载
下载帮助

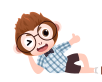
最新资源
资源上传下载、课程学习等过程中有任何疑问或建议,欢迎提出宝贵意见哦~我们会及时处理!
点击此处反馈


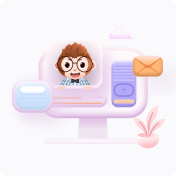
安全验证
文档复制为VIP权益,开通VIP直接复制
