# TA-Lib
This is a Python wrapper for [TA-LIB](http://ta-lib.org) based on Cython
instead of SWIG. From the homepage:
> TA-Lib is widely used by trading software developers requiring to perform
> technical analysis of financial market data.
> * Includes 150+ indicators such as ADX, MACD, RSI, Stochastic, Bollinger
> Bands, etc.
> * Candlestick pattern recognition
> * Open-source API for C/C++, Java, Perl, Python and 100% Managed .NET
The original Python bindings use [SWIG](http://swig.org) which unfortunately
are difficult to install and aren't as efficient as they could be. Therefore
this project uses Cython and Numpy to efficiently and cleanly bind to TA-Lib
-- producing results 2-4 times faster than the SWIG interface.
## Installation
You can install from PyPI:
```
$ pip install TA-Lib
```
Or checkout the sources and run ``setup.py`` yourself:
```
$ python setup.py install
```
### Troubleshooting
Sometimes installation will produce build errors like this:
```
func.c:256:28: fatal error: ta-lib/ta_libc.h: No such file or directory
compilation terminated.
```
This typically means that it can't find the underlying ``TA-Lib`` library,
a dependency which needs to be installed.
### Dependencies
To use TA-Lib for python, you need to have the
[TA-Lib](http://ta-lib.org/hdr_dw.html) already installed:
##### Mac OS X
```
$ brew install ta-lib
```
##### Windows
Download [ta-lib-0.4.0-msvc.zip](http://prdownloads.sourceforge.net/ta-lib/ta-lib-0.4.0-msvc.zip)
and unzip to ``C:\ta-lib``
##### Linux
Download [ta-lib-0.4.0-src.tar.gz](http://prdownloads.sourceforge.net/ta-lib/ta-lib-0.4.0-src.tar.gz) and:
```
$ untar and cd
$ ./configure --prefix=/usr
$ make
$ sudo make install
```
> If you build ``TA-Lib`` using ``make -jX`` it will fail but that's OK!
> Simply rerun ``make -jX`` followed by ``[sudo] make install``.
## Function API
Similar to TA-Lib, the Function API provides a lightweight wrapper of the
exposed TA-Lib indicators.
Each function returns an output array and have default values for their
parameters, unless specified as keyword arguments. Typically, these functions
will have an initial "lookback" period (a required number of observations
before an output is generated) set to ``NaN``.
For convenience, the Function API supports both ``numpy.ndarray`` and
``pandas.Series`` types.
All of the following examples use the Function API:
```python
import numpy
import talib
close = numpy.random.random(100)
```
Calculate a simple moving average of the close prices:
```python
output = talib.SMA(close)
```
Calculating bollinger bands, with triple exponential moving average:
```python
from talib import MA_Type
upper, middle, lower = talib.BBANDS(close, matype=MA_Type.T3)
```
Calculating momentum of the close prices, with a time period of 5:
```python
output = talib.MOM(close, timeperiod=5)
```
## Abstract API
If you're already familiar with using the function API, you should feel right
at home using the Abstract API.
Every function takes a collection of named inputs, either a ``dict`` of
``numpy.ndarray`` or ``pandas.Series``, or a ``pandas.DataFrame``. If a
``pandas.DataFrame`` is provided, the output is returned as a
``pandas.DataFrame`` with named output columns.
For example, inputs could be provided for the typical "OHLCV" data:
```python
import numpy as np
# note that all ndarrays must be the same length!
inputs = {
'open': np.random.random(100),
'high': np.random.random(100),
'low': np.random.random(100),
'close': np.random.random(100),
'volume': np.random.random(100)
}
```
Functions can either be imported directly or instantiated by name:
```python
from talib import abstract
# directly
sma = abstract.SMA
# or by name
sma = abstract.Function('sma')
```
From there, calling functions is basically the same as the function API:
```python
from talib.abstract import *
# uses close prices (default)
output = SMA(inputs, timeperiod=25)
# uses open prices
output = SMA(inputs, timeperiod=25, price='open')
# uses close prices (default)
upper, middle, lower = BBANDS(inputs, 20, 2, 2)
# uses high, low, close (default)
slowk, slowd = STOCH(inputs, 5, 3, 0, 3, 0) # uses high, low, close by default
# uses high, low, open instead
slowk, slowd = STOCH(inputs, 5, 3, 0, 3, 0, prices=['high', 'low', 'open'])
```
## Supported Indicators and Functions
We can show all the TA functions supported by TA-Lib, either as a ``list`` or
as a ``dict`` sorted by group (e.g. "Overlap Studies", "Momentum Indicators",
etc):
```python
import talib
# list of functions
print talib.get_functions()
# dict of functions by group
print talib.get_function_groups()
```
### Indicator Groups
* Overlap Studies
* Momentum Indicators
* Volume Indicators
* Volatility Indicators
* Price Transform
* Cycle Indicators
* Pattern Recognition
#### Overlap Studies
```
BBANDS Bollinger Bands
DEMA Double Exponential Moving Average
EMA Exponential Moving Average
HT_TRENDLINE Hilbert Transform - Instantaneous Trendline
KAMA Kaufman Adaptive Moving Average
MA Moving average
MAMA MESA Adaptive Moving Average
MAVP Moving average with variable period
MIDPOINT MidPoint over period
MIDPRICE Midpoint Price over period
SAR Parabolic SAR
SAREXT Parabolic SAR - Extended
SMA Simple Moving Average
T3 Triple Exponential Moving Average (T3)
TEMA Triple Exponential Moving Average
TRIMA Triangular Moving Average
WMA Weighted Moving Average
```
#### Momentum Indicators
```
ADX Average Directional Movement Index
ADXR Average Directional Movement Index Rating
APO Absolute Price Oscillator
AROON Aroon
AROONOSC Aroon Oscillator
BOP Balance Of Power
CCI Commodity Channel Index
CMO Chande Momentum Oscillator
DX Directional Movement Index
MACD Moving Average Convergence/Divergence
MACDEXT MACD with controllable MA type
MACDFIX Moving Average Convergence/Divergence Fix 12/26
MFI Money Flow Index
MINUS_DI Minus Directional Indicator
MINUS_DM Minus Directional Movement
MOM Momentum
PLUS_DI Plus Directional Indicator
PLUS_DM Plus Directional Movement
PPO Percentage Price Oscillator
ROC Rate of change : ((price/prevPrice)-1)*100
ROCP Rate of change Percentage: (price-prevPrice)/prevPrice
ROCR Rate of change ratio: (price/prevPrice)
ROCR100 Rate of change ratio 100 scale: (price/prevPrice)*100
RSI Relative Strength Index
STOCH Stochastic
STOCHF Stochastic Fast
STOCHRSI Stochastic Relative Strength Index
TRIX 1-day Rate-Of-Change (ROC) of a Triple Smooth EMA
ULTOSC Ultimate Oscillator
WILLR Williams' %R
```
#### Volume Indicators
```
AD Chaikin A/D Line
ADOSC Chaikin A/D Oscillator
OBV On Balance Volume
```
#### Cycle Indicators
```
HT_DCPERIOD Hilbert Transform - Dominant Cycle Period
HT_DCPHASE Hilbert Transform - Dominant Cycle Phase
HT_PHASOR Hilbert Transform - Phasor Components
HT_SINE Hilbert Transform - SineWave
HT_TRENDMODE Hilbert Transform - Trend vs Cycle Mode
```
#### Price Transform
```
AVGPRICE Average Price
MEDPRICE Median Price
TYPPRICE Typical Price
WCLPRICE Weighted Close Price
```
#### Volatility Indicators
```
ATR Average True Range
NATR Normalized Average True Range
没有合适的资源?快使用搜索试试~ 我知道了~
温馨提示
安装方法 TA-Lib的安装方法因操作系统和编程语言的不同而有所差异。以下是在Windows系统上使用Python安装TA-Lib的示例步骤: 下载TA-Lib的源代码或预编译包,并解压到指定目录。 安装Visual Studio等必要的开发工具,并确保勾选了Visual C++功能。 使用Visual Studio的命令提示符工具,进入TA-Lib的源代码目录,并执行构建命令(如nmake)来生成TA-Lib的动态链接库(DLL)文件。 将生成的DLL文件复制到Python的库目录中,或者将其路径添加到系统的环境变量中。 使用pip命令安装TA-Lib的Python接口包(如pip install TA-Lib)。
资源推荐
资源详情
资源评论
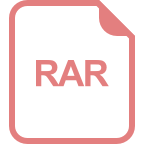
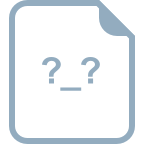
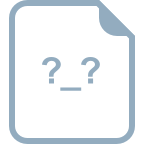
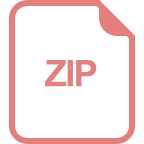
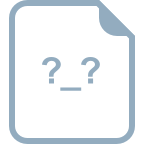
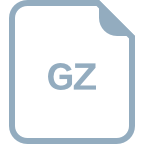
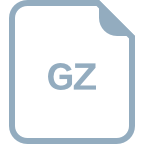
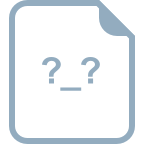
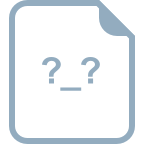
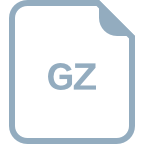
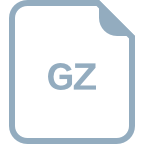
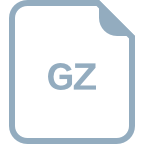
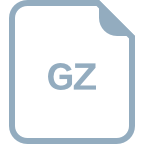
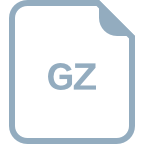
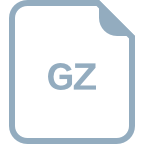
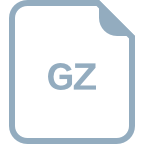
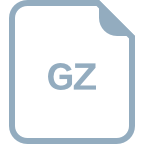
收起资源包目录


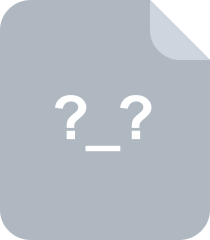
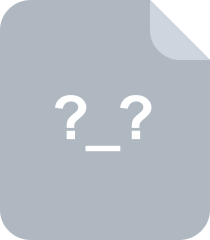
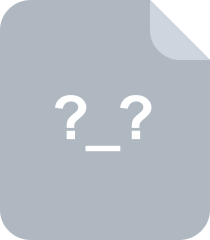
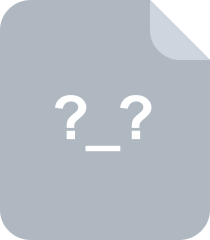
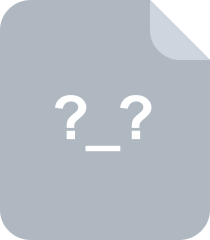

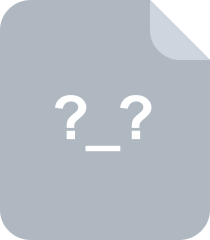
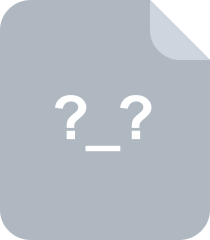
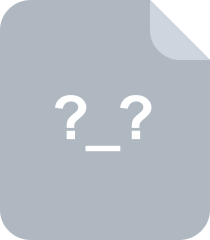
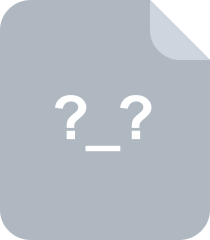
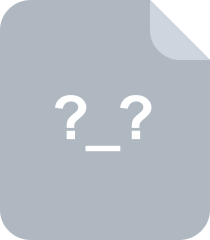
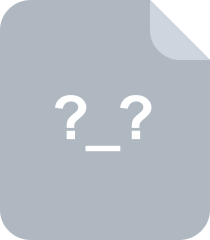
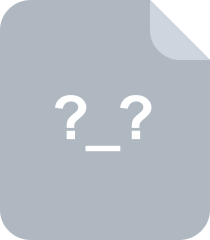
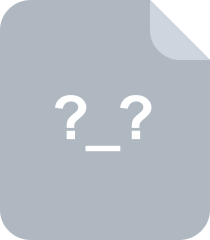
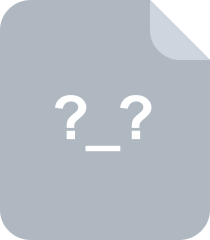
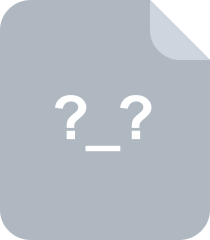
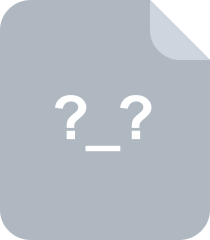
共 16 条
- 1
资源评论
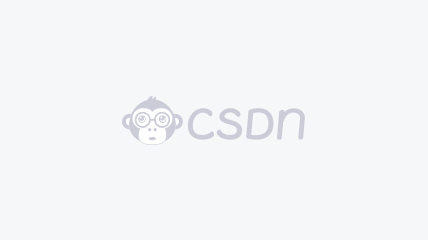

onnx
- 粉丝: 9778
- 资源: 5615
上传资源 快速赚钱
我的内容管理 展开
我的资源 快来上传第一个资源
我的收益
登录查看自己的收益我的积分 登录查看自己的积分
我的C币 登录后查看C币余额
我的收藏
我的下载
下载帮助

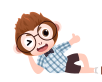
最新资源
资源上传下载、课程学习等过程中有任何疑问或建议,欢迎提出宝贵意见哦~我们会及时处理!
点击此处反馈


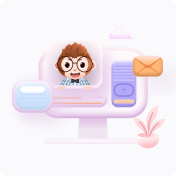
安全验证
文档复制为VIP权益,开通VIP直接复制
