0/199 5.51G 0.1073 0.0373 0.02152 0.1661 101 640 0.07267 0.4602 0.1566 0.03113 0.0829 0.03484 0.007563
1/199 5.5G 0.07996 0.02738 0.007438 0.1148 59 640 0.06386 0.4904 0.249 0.08068 0.07274 0.02475 0.004912
2/199 5.5G 0.07205 0.02287 0.005353 0.1003 69 640 0.1457 0.6301 0.2885 0.1082 0.06449 0.0213 0.004275
3/199 5.5G 0.06458 0.02092 0.004851 0.09035 73 640 0.2459 0.7153 0.435 0.175 0.05115 0.02019 0.004015
4/199 5.5G 0.05754 0.01958 0.004645 0.08176 63 640 0.4178 0.8665 0.6637 0.346 0.0409 0.01832 0.003792
5/199 5.5G 0.05189 0.01884 0.004472 0.0752 109 640 0.4653 0.8075 0.6009 0.3556 0.03773 0.01721 0.003672
6/199 5.5G 0.04762 0.01707 0.003917 0.0686 77 640 0.5113 0.8596 0.714 0.3697 0.03714 0.01672 0.003626
7/199 5.5G 0.04438 0.0166 0.003723 0.06471 71 640 0.4742 0.8826 0.7491 0.3521 0.03442 0.01531 0.003417
8/199 5.5G 0.04196 0.01606 0.003738 0.06176 70 640 0.5388 0.9417 0.8256 0.4502 0.03039 0.01469 0.003303
9/199 5.5G 0.0399 0.01565 0.003894 0.05945 79 640 0.6144 0.9324 0.8875 0.4886 0.02787 0.0138 0.003189
10/199 5.5G 0.0398 0.01577 0.003713 0.05929 62 640 0.6374 0.853 0.7897 0.3457 0.03761 0.0213 0.003943
11/199 5.5G 0.04748 0.0171 0.003987 0.06857 80 640 0.6839 0.9358 0.9026 0.5017 0.0336 0.01518 0.003432
12/199 5.5G 0.04599 0.01599 0.00403 0.06601 61 640 0.5924 0.9651 0.8905 0.5207 0.02902 0.01455 0.003427
13/199 5.5G 0.04155 0.01535 0.003717 0.06062 66 640 0.6506 0.9557 0.9019 0.4987 0.03084 0.01422 0.003359
14/199 5.5G 0.04131 0.01511 0.003738 0.06015 69 640 0.6623 0.968 0.9321 0.5347 0.02946 0.01405 0.003264
15/199 5.5G 0.03972 0.01543 0.003876 0.05903 62 640 0.6647 0.9115 0.8511 0.4652 0.03141 0.01384 0.003272
16/199 5.5G 0.03698 0.01482 0.003686 0.05548 79 640 0.6831 0.9519 0.9126 0.5262 0.02993 0.01329 0.003104
17/199 5.5G 0.03532 0.01446 0.00349 0.05327 77 640 0.6958 0.9569 0.9215 0.5102 0.029 0.01281 0.003116
18/199 5.5G 0.03543 0.01463 0.003646 0.05371 88 640 0.679 0.942 0.9063 0.5124 0.0298 0.01286 0.003061
19/199 5.5G 0.03363 0.01397 0.003282 0.05089 91 640 0.765 0.9416 0.9285 0.5973 0.02465 0.0122 0.002963
20/199 5.5G 0.03272 0.01418 0.003109 0.05 65 640 0.6961 0.9763 0.9507 0.6061 0.02324 0.0122 0.002902
21/199 5.5G 0.03137 0.01379 0.003225 0.04838 73 640 0.7002 0.958 0.9331 0.557 0.02357 0.01227 0.002907
22/199 5.5G 0.02941 0.01346 0.002746 0.04561 110 640 0.7145 0.9393 0.9034 0.5235 0.02574 0.01198 0.002758
23/199 5.5G 0.02844 0.01312 0.002691 0.04425 61 640 0.7538 0.9641 0.9451 0.5865 0.02476 0.01212 0.002733
24/199 5.5G 0.02794 0.01307 0.003061 0.04407 77 640 0.7917 0.9625 0.953 0.6104 0.02222 0.01142 0.002723
25/199 5.5G 0.02666 0.01266 0.003206 0.04253 73 640 0.7684 0.967 0.9545 0.605 0.02178 0.0112 0.002701
26/199 5.5G 0.02568 0.01208 0.003123 0.04089 69 640 0.8077 0.9574 0.9495 0.624 0.0207 0.01111 0.002672
27/199 5.5G 0.02489 0.01271 0.003188 0.04079 104 640 0.8295 0.9738 0.9647 0.6792 0.01927 0.01076 0.002675
28/199 5.5G 0.02553 0.01246 0.00322 0.0412 88 640 0.8346 0.963 0.9547 0.6253 0.02178 0.01121 0.002647
29/199 5.5G 0.02517 0.01259 0.003102 0.04087 78 640 0.8061 0.9808 0.9713 0.672 0.0198 0.0112 0.002641
30/199 5.5G 0.02418 0.01201 0.003134 0.03932 93 640 0.8159 0.9818 0.9703 0.7031 0.01792 0.01055 0.002608
31/199 5.5G 0.02318 0.01209 0.002887 0.03816 103 640 0.8184 0.9739 0.9651 0.6684 0.01984 0.01075 0.002557
32/199 5.5G 0.02278 0.01198 0.003154 0.03792 77 640 0.8038 0.9758 0.963 0.6675 0.01784 0.01002 0.002556
33/199 5.5G 0.02251 0.01201 0.002631 0.03714 105 640 0.8109 0.9696 0.9601 0.6539 0.01886 0.01035 0.002519
34/199 5.5G 0.02223 0.01127 0.002827 0.03633 94 640 0.8291 0.9715 0.9619 0.68 0.01925 0.01038 0.002522
35/199 5.5G 0.02253 0.01173 0.002551 0.03681 101 640 0.8282 0.9718 0.9639 0.6711 0.01869 0.01046 0.002508
36/199 5.5G 0.0217 0.01161 0.003003 0.03631 68 640 0.8083 0.9629 0.9542 0.6303 0.02012 0.01041 0.002487
37/199 5.5G 0.02159 0.01134 0.002782 0.03571 74 640 0.8345 0.9639 0.9562 0.6158 0.0204 0.01058 0.002517
38/199 5.5G 0.0209 0.01127 0.002866 0.03504 69 640 0.8467 0.9746 0.9685 0.6845 0.01824 0.01024 0.00247
39/199 5.5G 0.02125 0.01112 0.003147 0.03552 88 640 0.8506 0.9796 0.972 0.6843 0.01783 0.01013 0.002489
40/199 5.5G 0.02107 0.01079 0.003228 0.03509 75 640 0.8408 0.9801 0.9726 0.6899 0.01788 0.01008 0.00249
41/199 5.5G 0.01984 0.0107 0.003184 0.03373 104 640 0.8264 0.9798 0.9714 0.6794 0.01733 0.00976 0.002449
42/199 5.5G 0.02049 0.01088 0.002656 0.03402 81 640 0.8543 0.9813 0.9755 0.7083 0.01679 0.009795 0.002432
43/199 5.5G 0.02056 0.01077 0.003152 0.03449 65 640 0.8321 0.9784 0.9689 0.6924 0.01729 0.009819 0.002442
44/199 5.5G 0.01972 0.01073 0.002701 0.03315 93 640 0.8579 0.9772 0.9709 0.6958 0.01726 0.009836 0.002438
45/199 5.5G 0.02028 0.01094 0.002755 0.03397 91 640 0.8654 0.9729 0.9682 0.6935 0.01723 0.009874 0.002422
46/199 5.5G 0.01876 0.01054 0.002466 0.03176 107 640 0.8726 0.9763 0.9722 0.6873 0.01729 0.009513 0.002389
47/199 5.5G 0.01985 0.01087 0.002883 0.0336 116 640 0.8725 0.9803 0.9731 0.6871 0.0172 0.00981 0.002432
48/199 5.5G 0.0197 0.01091 0.002649 0.03327 64 640 0.834 0.9796 0.9738 0.7034 0.01685 0.009948 0.00237
49/199 5.5G 0.01952 0.01066 0.002617 0.03279 88 640 0.8404 0.9755 0.9694 0.6924 0.01676 0.009517 0.002365
50/199 5.5G 0.01912 0.01061 0.002854 0.03259 97 640 0.8458 0.9822 0.9765 0.7183 0.01591 0.009379 0.002347
51/199 5.5G 0.01835 0.01042 0.002469 0.03124 89 640 0.8601 0.9818 0.9773 0.7302 0.01587 0.009428 0.002314
52/199 5.5G 0.01879 0.01039 0.003126 0.03231 76 640 0.844 0.9742 0.9693 0.6886 0.01726 0.009644 0.00233
没有合适的资源?快使用搜索试试~ 我知道了~
温馨提示
1、资源包含yolov5检测源码+车辆车牌检测模型(监控视角)+使用说明 2、附有训练pr曲线、损失值曲线、召回率曲线、精确度曲线、mAP等评估指标曲线 3、迭代200次,模型拟合较好。 【特别强调】 1、csdn上资源保证是完整最新,会不定期更新优化; 2、通过第三方代下载,而不是直接自己账号在csdn官方下载,博主不对下载的资源作任何保证,且不提供任何形式的技术支持和答疑!!!
资源推荐
资源详情
资源评论
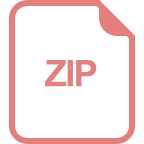
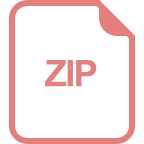
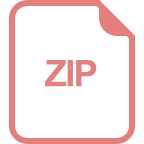
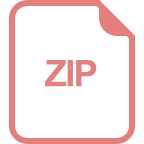
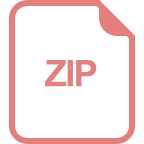
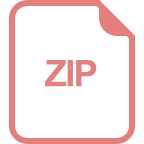
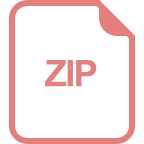
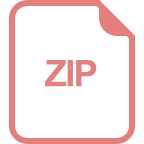
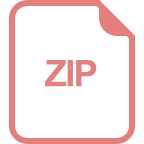
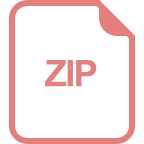
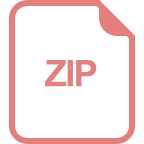
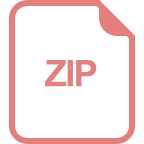
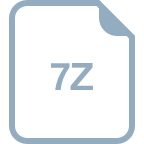
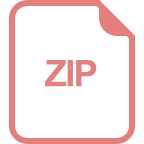
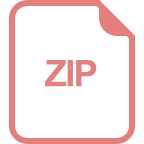
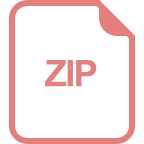
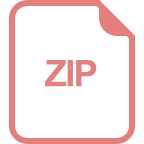
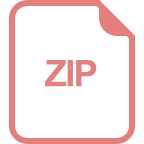
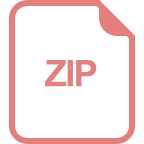
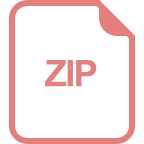
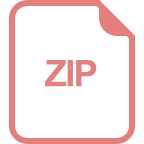
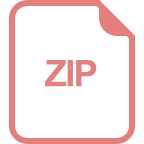
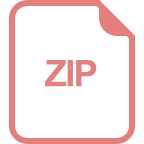
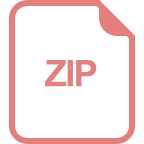
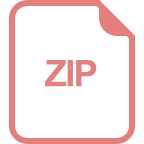
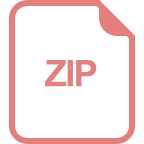
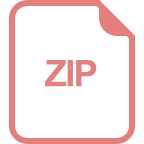
收起资源包目录




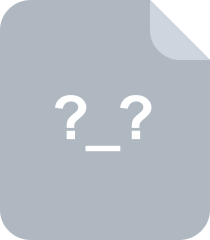
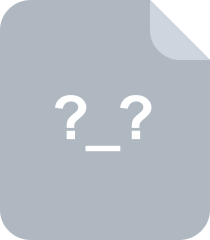

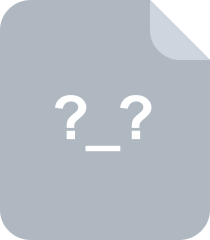
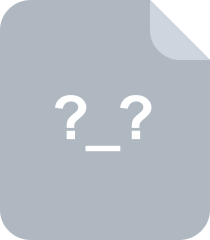
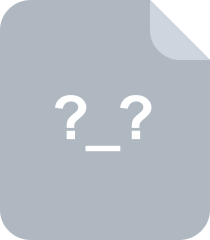
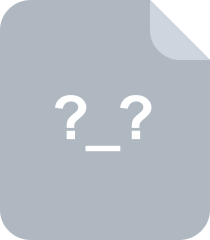

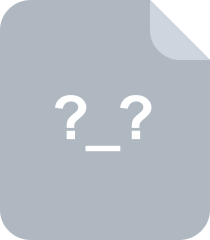
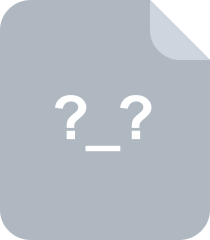
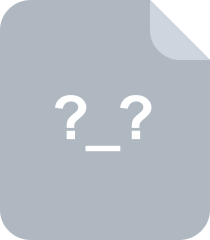
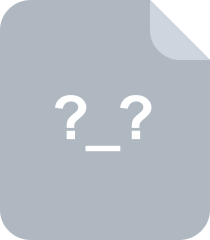
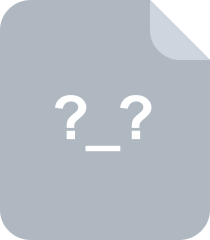
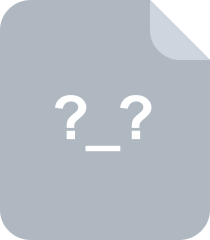
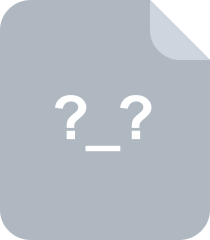
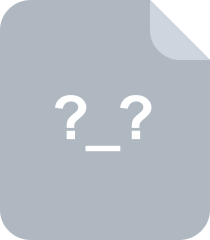
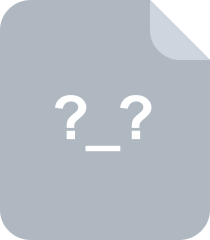
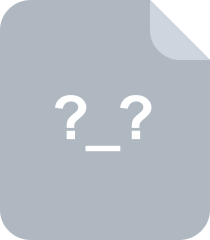
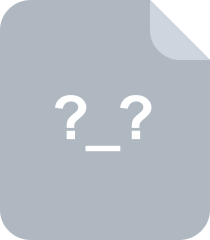
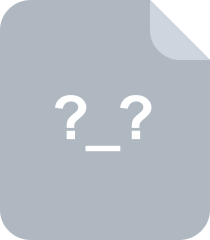
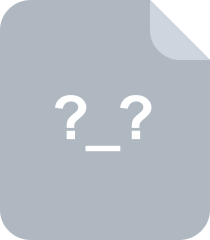
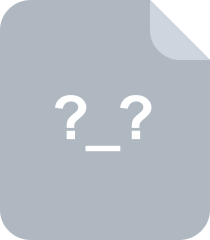


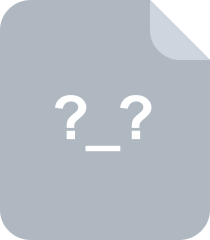
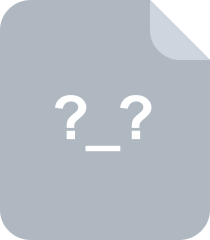

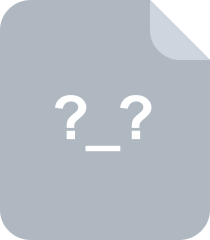

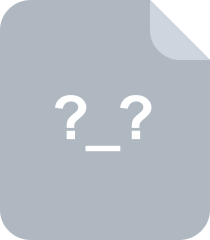
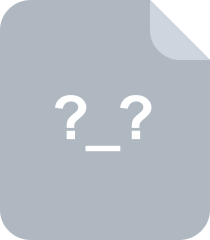
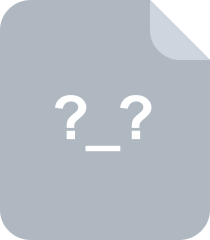
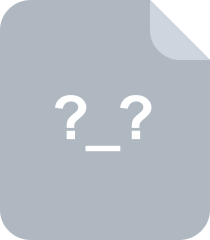
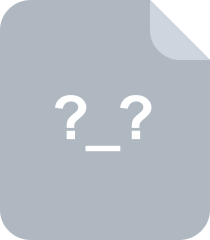

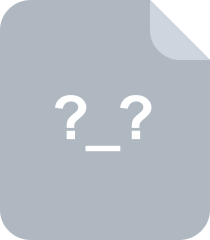
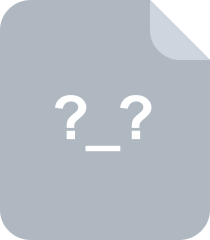
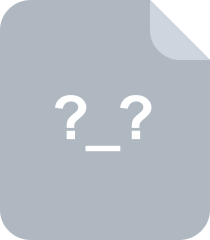
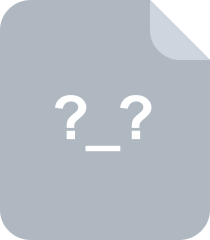
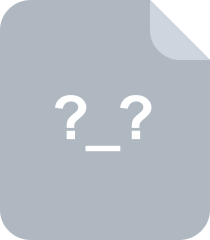

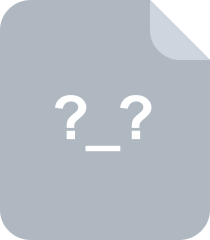
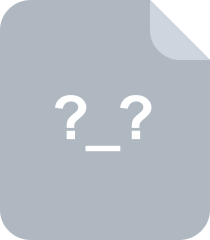
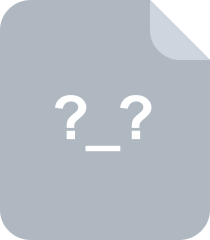
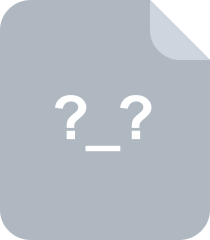
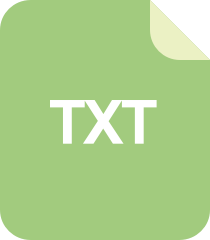


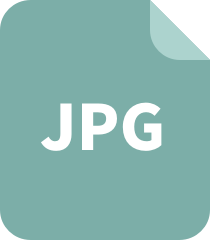

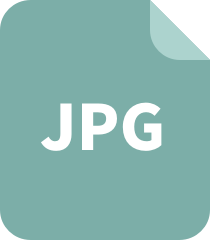



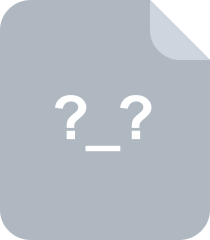
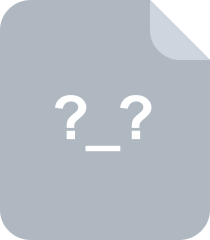
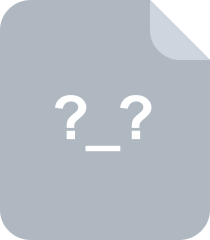
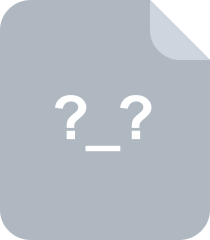
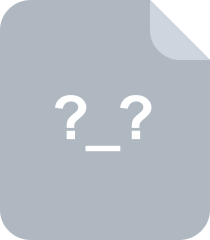
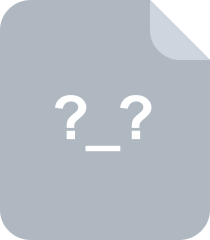
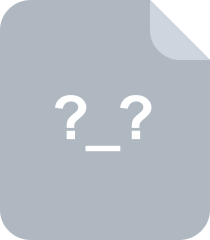
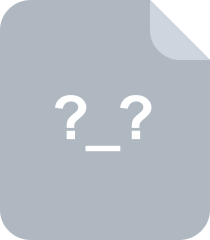
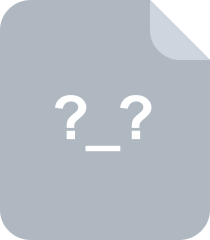
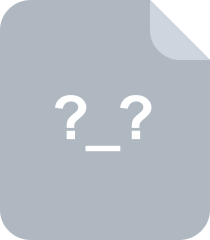
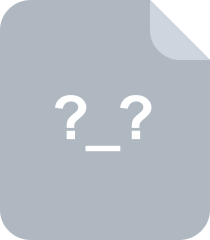
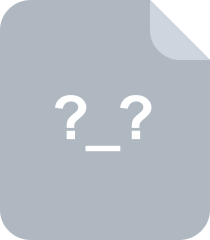
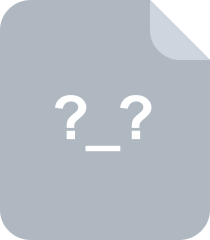
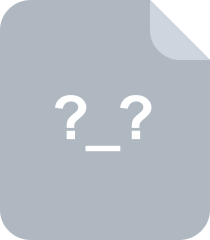
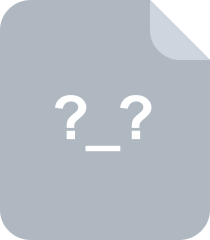
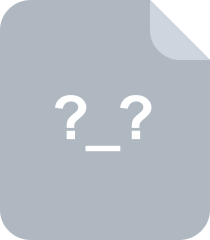
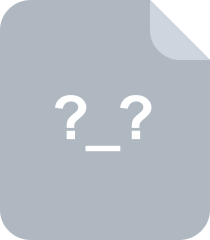
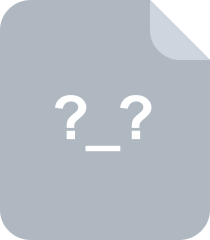

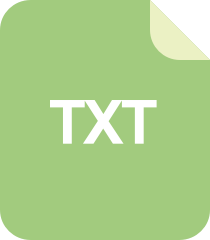
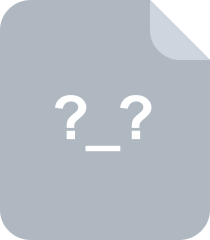
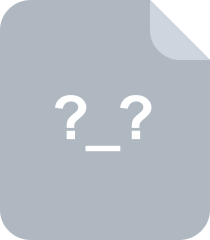
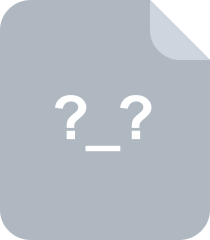
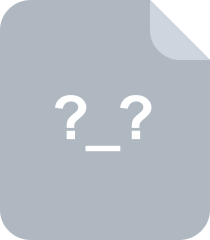
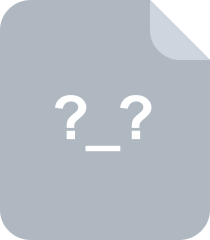
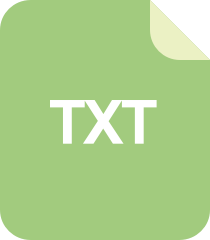

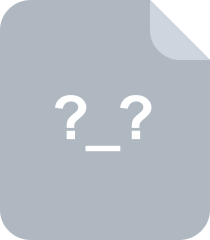
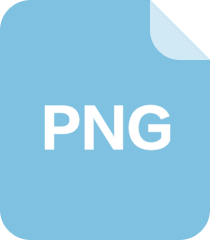
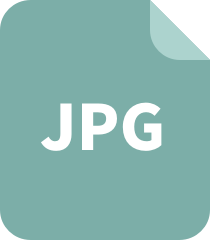
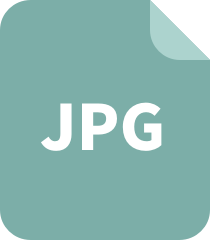
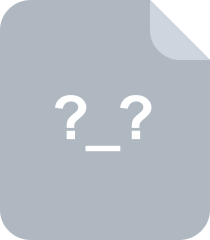
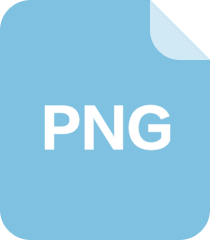
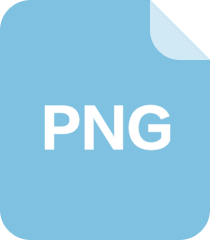
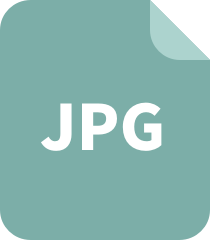
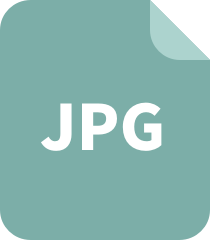
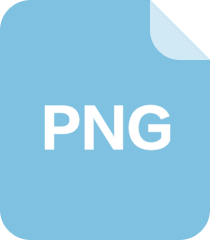
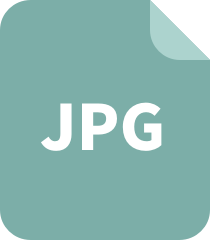

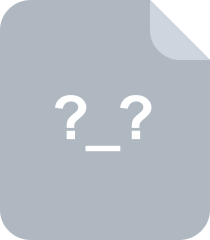
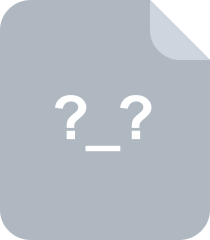
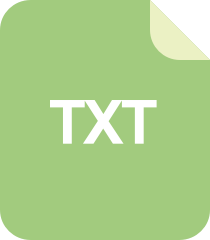
共 79 条
- 1
资源评论
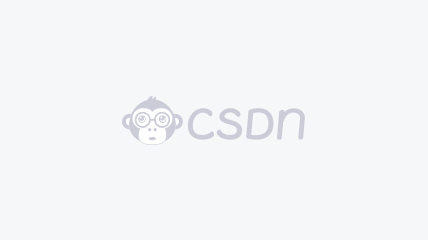
- MA_Zero2024-05-17资源值得借鉴的内容很多,那就浅学一下吧,值得下载!onnx2024-11-01undefined
- xhnwhhh2024-04-27资源内容详细,总结地很全面,与描述的内容一致,对我启发很大,学习了。onnx2024-05-10谢谢认可和支持!
- 看、风吹落叶2023-03-11总算找到了想要的资源,搞定遇到的大问题,赞赞赞!onnx2024-05-10嗯嗯,加油啊啊

onnx
- 粉丝: 9501
- 资源: 5595
上传资源 快速赚钱
我的内容管理 展开
我的资源 快来上传第一个资源
我的收益
登录查看自己的收益我的积分 登录查看自己的积分
我的C币 登录后查看C币余额
我的收藏
我的下载
下载帮助

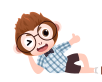
最新资源
资源上传下载、课程学习等过程中有任何疑问或建议,欢迎提出宝贵意见哦~我们会及时处理!
点击此处反馈


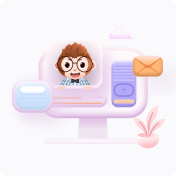
安全验证
文档复制为VIP权益,开通VIP直接复制
