# GMM-Gaussian-Mixture-Model-
These code snippets utilize the Gaussian Mixture Model (GMM) to forecast future data for solar energy and channel gain in IoT devices. Within the GMM.py file, there are three distinct GMM fitting methods tailored for variables of different dimensions.
这些代码片段使用高斯混合模型(GMM)来预测太阳能和IoT设备中的信道增益的未来数据。在GMM.py文件中,有三种针对不同维度变量的GMM拟合方法。
mec3 is developed using Pyomo and represents a MILP (Mixed Integer Linear Programming) model.
RHC serves as an experimental file to analyze the energy consumption of heuristic solutions.
test is an experimental file designed to evaluate the energy consumption of both optimal and random solutions.
random_decision is tailored for generating random strategies concerning channel allocation and mode execution approximations.
Both NetworkBasics and Solar are crucial files responsible for generating key parameters. Notably, both them were provided by my tutor.
mec3 是使用Pyomo开发的,并代表一个MILP(混合整数线性编程)模型。
RHC 作为一个实验文件,用来分析启发式解决方案的能耗。
test 是一个实验文件,旨在评估最优解和随机解的能耗。
random_decision 专门用于生成关于信道分配和近似模式执行的随机策略。
NetworkBasics 和 Solar 都是生成关键参数的关键文件, 值得注意的是,它们两个都是由我的导师提供的的。

2401_87496566
- 粉丝: 521
- 资源: 2303
最新资源
- 基于SPH-FEM泥石流模拟冲击拦挡坝视频教程 此教程为SPH泥石流浆体冲击FEM拦挡坝,详细介绍了浆体和拦挡坝建模,两者之间的
- PSIM仿真,升降压谐振变器复现仿真 解析文档
- 基于Java语言的文件操作基础模块设计源码
- FLUENT与MATLAB联合仿真计算,基于UDP,可在MATLAB实现复杂数据计算处理 提供两个软件数据交互方法和接口,FL
- (三台)集群式并联-构网型VSG逆变器并联,采用电压电流双闭环控制方式,VSG控制 支持simulink2022以下版本,联系
- 基于Python的核设备二大作业排布图生成软件源码设计
- 基于鸿蒙系统的ZBar条形码阅读器设计源码
- 基于Java及多语言整合的实习项目代码库设计源码
- COMSOL岩石损伤、水力压裂、三轴试验 岩石在膨胀剂的膨胀作用下的损伤; 相场法与水力压裂(6个模型); 不固结不排水三轴试验
- 基于HTML和JavaScript的tableExport.jquery.plugin表格导出插件源码分享
资源上传下载、课程学习等过程中有任何疑问或建议,欢迎提出宝贵意见哦~我们会及时处理!
点击此处反馈


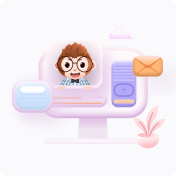