基于多模态融合的驾驶人愤怒情绪识别方法研究 本研究基于多模态融合的驾驶人愤怒情绪识别方法研究,旨在提高交通安全。研究表明,当驾驶员情绪愤怒时,其感知、判断、决策和执行能力将受到负面影响,导致驾驶能力下降,容易发生交通事故,造成人员伤亡和财产损失。因此,研究驾驶人愤怒识别方法是提高交通安全的重要步骤。 目前,国内外研究者已进行了大量的研究工作,但大多数只关注单模态情绪识别。由于单模态无法充分表达情绪信息,因此基于单模态的情绪识别准确性和鲁棒性较低。本研究建立了基于ECG信号和驾驶行为信号的多模态融合驾驶人愤怒识别方法,构建了驾驶人愤怒数据集,并使用支持向量机对愤怒和平静情绪进行分类和识别。 研究的具体步骤如下: 对情感诱导材料进行筛选。通过互联网平台对情感诱导视频材料进行筛选,分析情感材料校准测试结果和离散情感模型的DES量表,选择成功率最高和情感诱导平均分最高的视频材料作为激发参与者的愤怒和平静情绪。 设计驾驶愤怒模拟实验,并收集测试数据。使用情感诱导测试来激发参与者的愤怒和平静情绪,并进行驾驶模拟测试,记录ECG信号和驾驶行为信号,结合DES量表的主观评价。 研究了ECG信号和驾驶行为信号之间的关系,并使用支持向量机对愤怒和平静情绪进行分类和识别。研究结果表明,本方法可以较好地识别驾驶人的愤怒情绪,提高交通安全。 相关知识点: 1. 多模态融合:通过将多种模态信息(如ECG信号、驾驶行为信号等)融合,提高情绪识别的准确性和鲁棒性。 2. 驱动人愤怒情绪识别:研究驾驶人的愤怒情绪识别方法,以提高交通安全。 3. ECG信号:使用ECG信号来识别驾驶人的愤怒情绪。 4. 驾驶行为信号:使用驾驶行为信号来识别驾驶人的愤怒情绪。 5. 支持向量机:使用支持向量机对愤怒和平静情绪进行分类和识别。 6. 情感诱导测试:使用情感诱导测试来激发参与者的愤怒和平静情绪。 7. DES量表:使用DES量表来评价参与者的情感状态。 8. 驱动模拟实验:设计驾驶愤怒模拟实验来收集测试数据。 9. 多模态融合认知:研究多模态融合认知的应用于驾驶人愤怒情绪识别。 10. 交通安全:研究驾驶人愤怒情绪识别方法的应用于提高交通安全。
















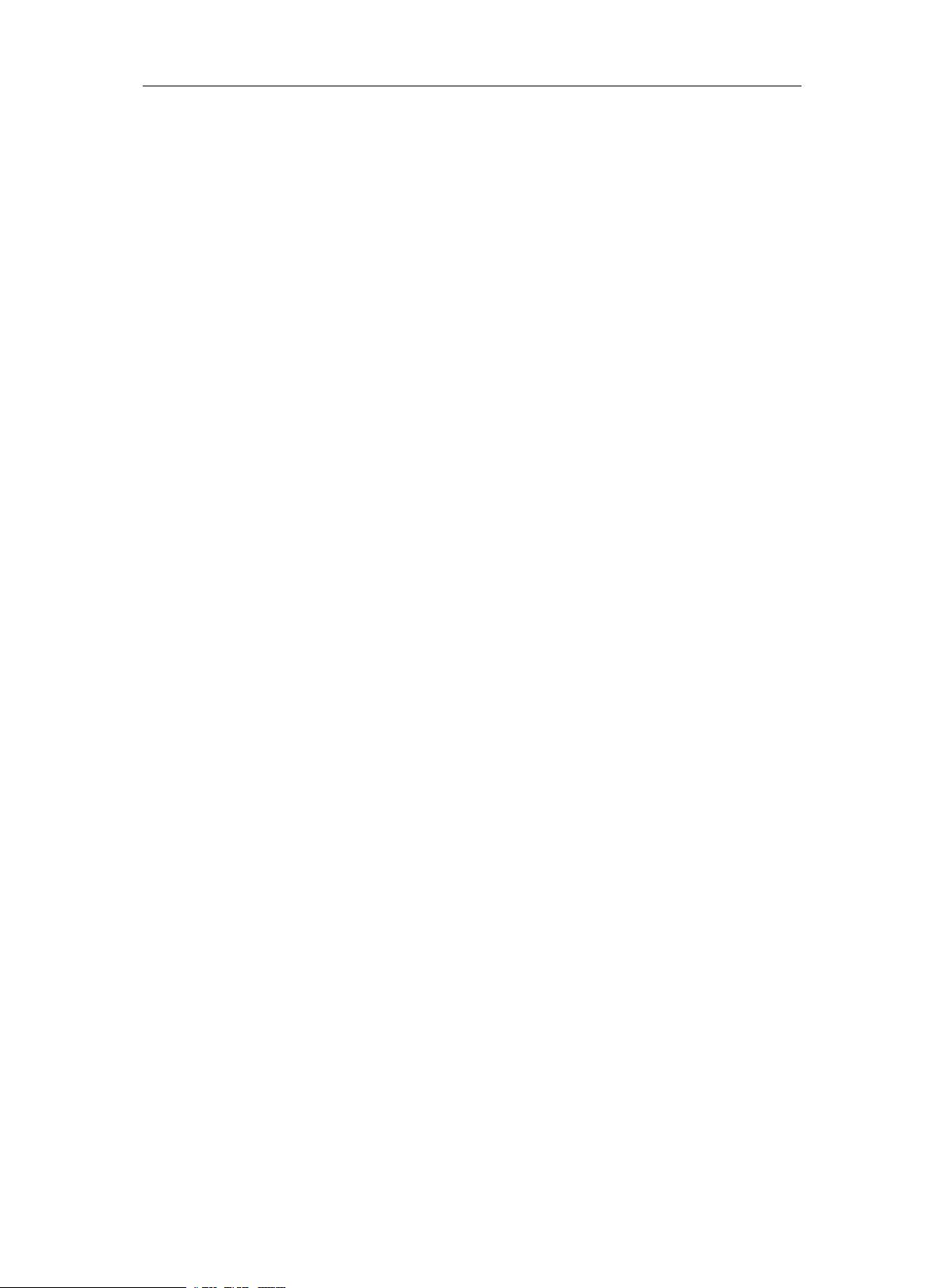
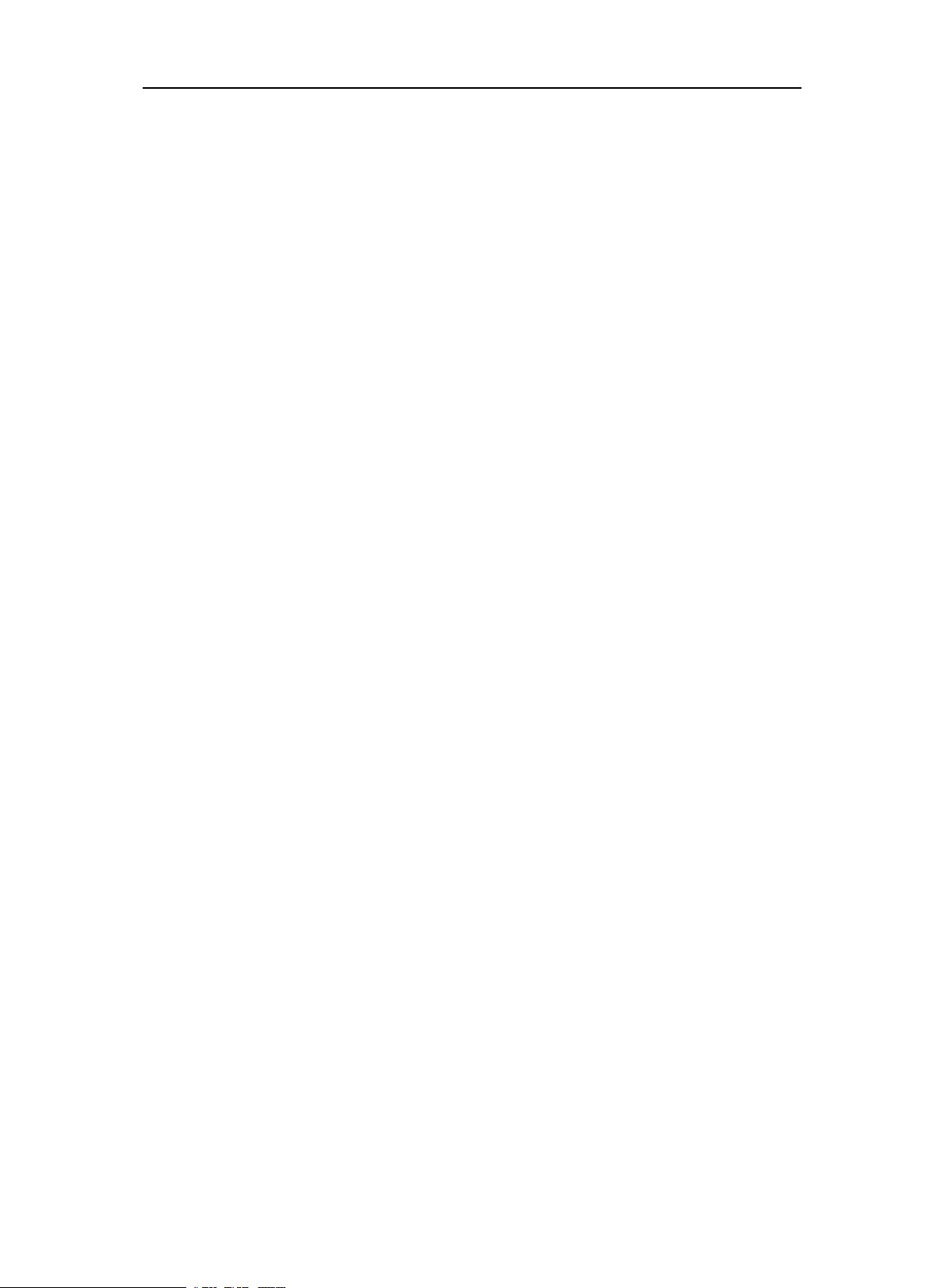
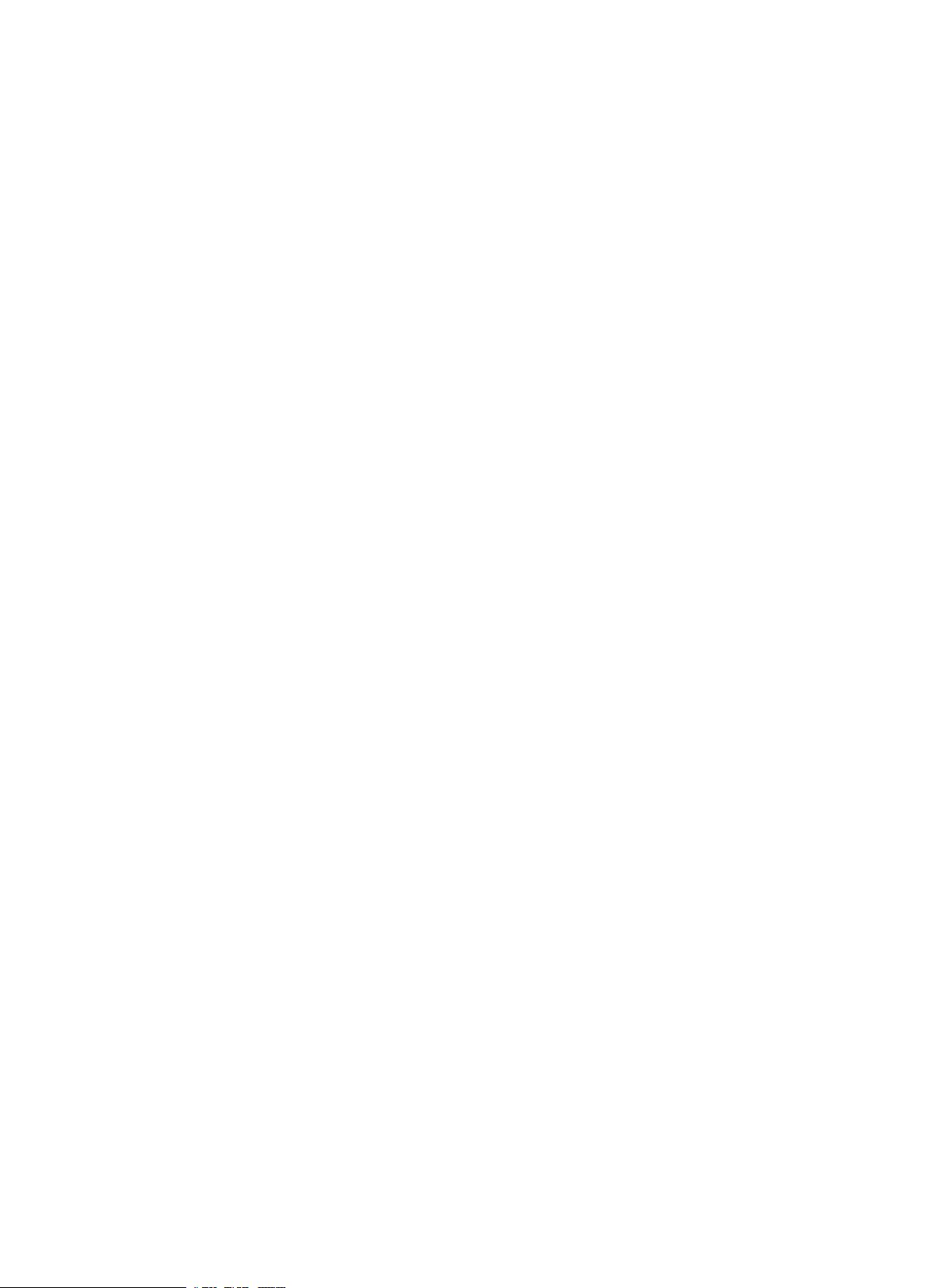
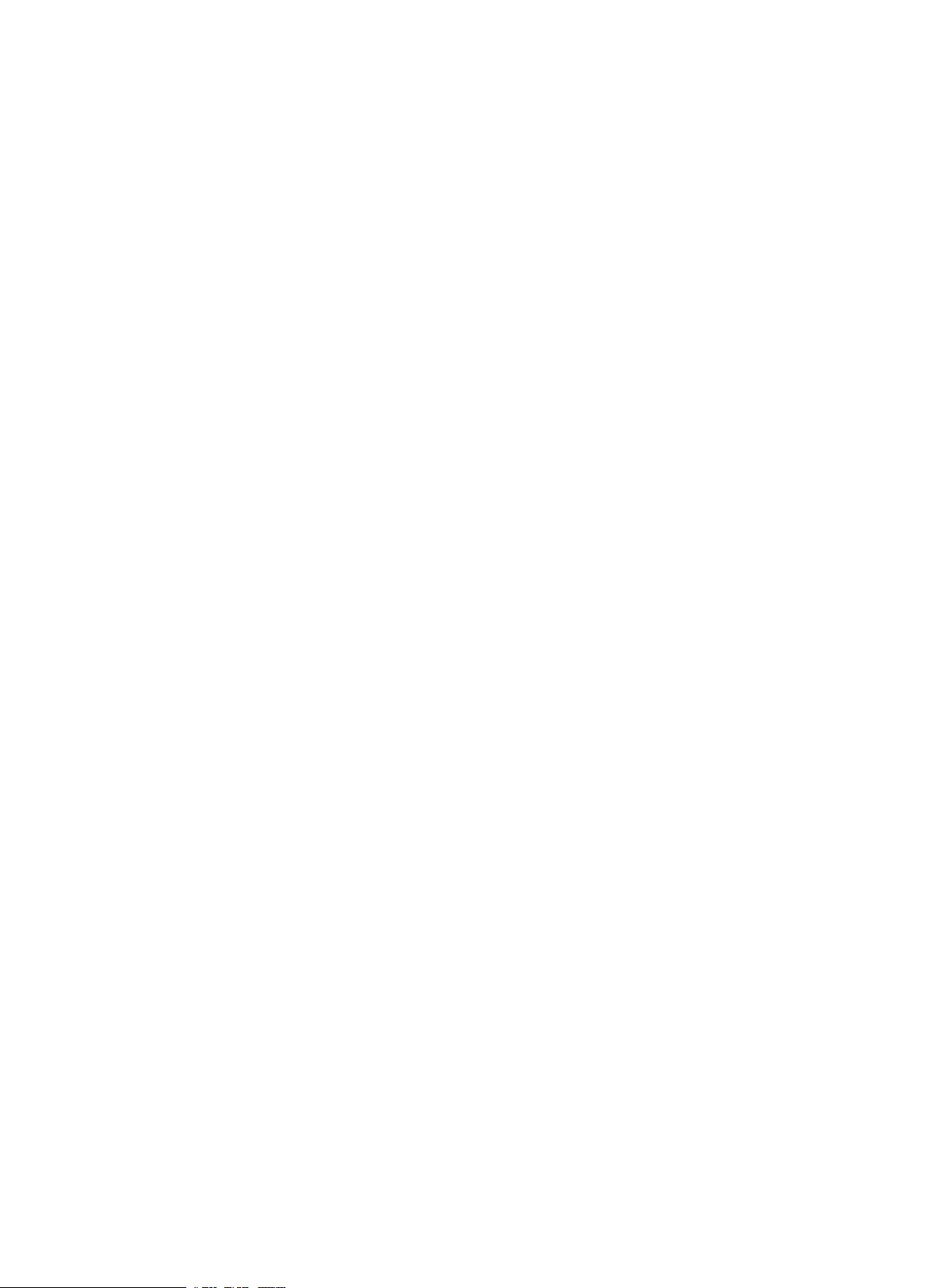
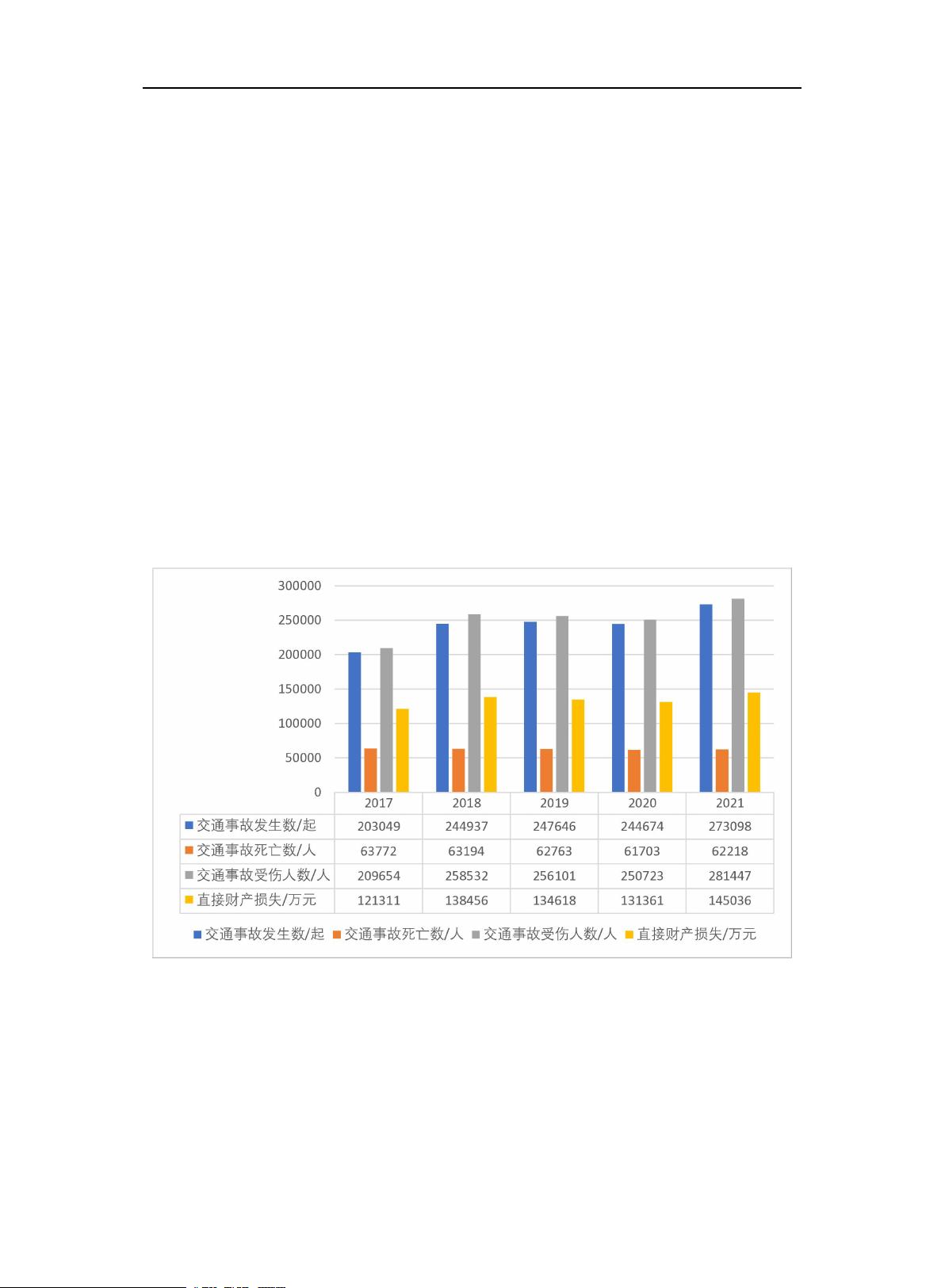
剩余72页未读,继续阅读
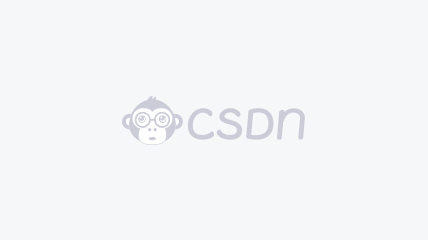
- 2301_768119552024-10-15资源不错,内容挺好的,有一定的使用价值,值得借鉴,感谢分享。


- 粉丝: 29
- 资源: 7802





我的内容管理 展开
我的资源 快来上传第一个资源
我的收益
登录查看自己的收益我的积分 登录查看自己的积分
我的C币 登录后查看C币余额
我的收藏
我的下载
下载帮助

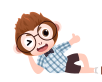
最新资源
- 01-【管理制度】-55-公司员工培训手册管理人力资源管理制度.docx
- 01-【管理制度】-59-人力资源管理制度汇编.doc
- 01-【管理制度】-60-人力资源管理制度汇编.doc
- 02-【管理流程】-01-人力资源管理流程图.docx
- 02-【管理流程】-04-招聘录用流程图.docx
- 02-【管理流程】-02-人力资源管理流程.doc
- 02-【管理流程】-03-HR工作流程图.docx
- 02-【管理流程】-05-招聘流程图.docx
- 02-【管理流程】-06-员工入职流程图.docx
- 02-【管理流程】-07-员工入职流程图.docx
- 02-【管理流程】-10-员工转正流程图.docx
- 02-【管理流程】-08-入职流程图.docx
- 02-【管理流程】-09-新员工入职培训流程图.docx
- 02-【管理流程】-11-试用期转正流程图.docx
- 02-【管理流程】-13-员工晋升流程图.docx
- 02-【管理流程】-12-晋升管理流程图.docx

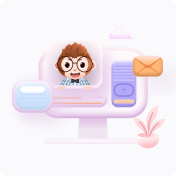
